ℓ1-Penalized Linear Mixed-Effects Models for BCI
ICANN'11: Proceedings of the 21th international conference on Artificial neural networks - Volume Part I(2011)
摘要
A recently proposed novel statistical model estimates population effects and individual variability between subgroups simultaneously, by extending Lasso methods. We apply this l 1 -penalized linear regression mixed-effects model to a large scale real world problem: by exploiting a large set of brain computer interface data we are able to obtain a subject-independent classifier that compares favorably with prior zero-training algorithms. This unifying model inherently compensates shifts in the input space attributed to the individuality of a subject. In particular we are now able to differentiate within-subject and between-subject variability. A deeper understanding both of the underlying statistical and physiological structure of the data is gained.
更多查看译文
关键词
Linear Discriminant Analysis, Brain Computer Interface, Common Spatial Pattern, Balance Dataset, Unbalanced Dataset
AI 理解论文
溯源树
样例
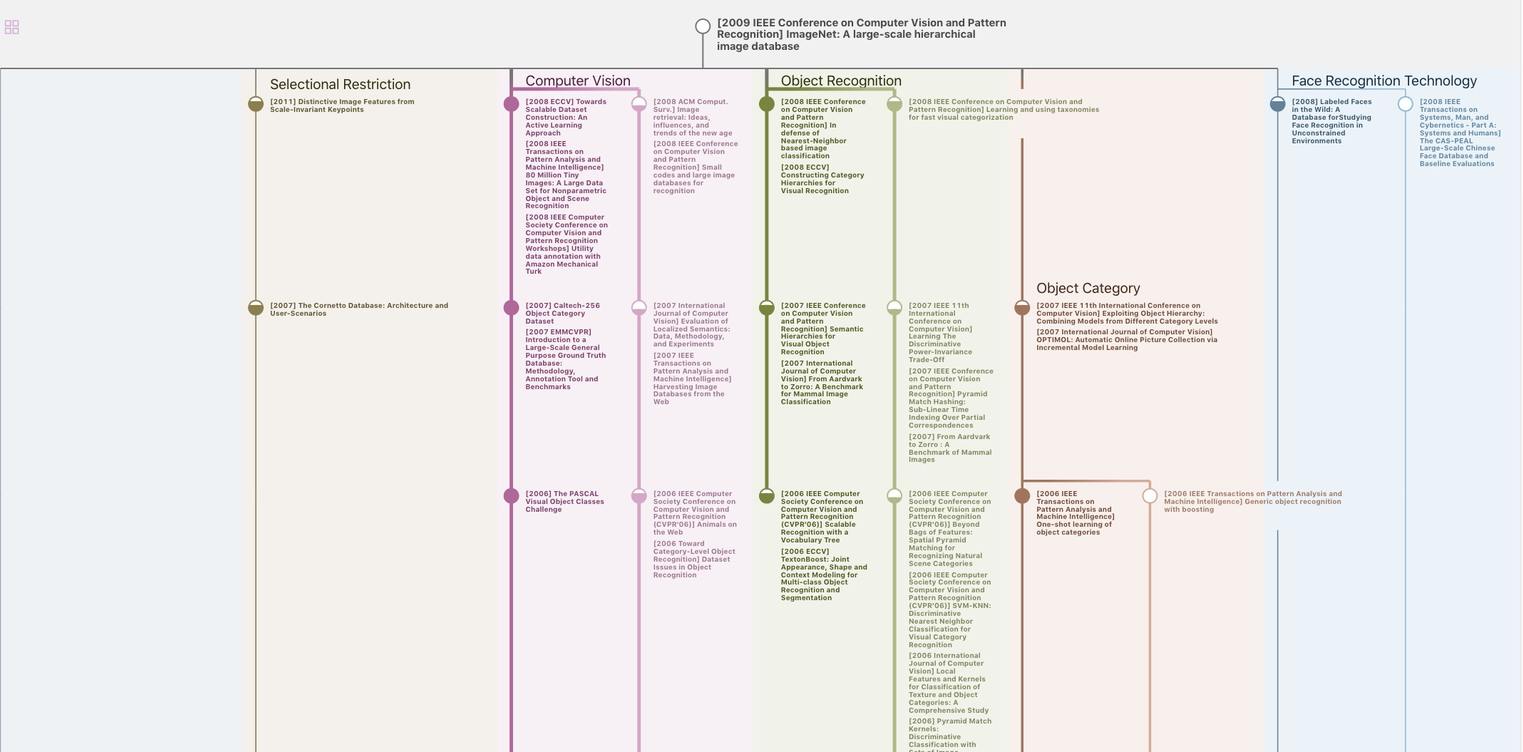
生成溯源树,研究论文发展脉络
Chat Paper
正在生成论文摘要