Robust Unsupervised Feature Selection.
IJCAI '13: Proceedings of the Twenty-Third international joint conference on Artificial Intelligence(2013)
摘要
A new unsupervised feature selection method, i.e., Robust Unsupervised Feature Selection (RUFS), is proposed. Unlike traditional unsupervised feature selection methods, pseudo cluster labels are learned via local learning regularized robust nonnegative matrix factorization. During the label learning process, feature selection is performed simultaneously by robust joint l 2,1 norms minimization. Since RUFS utilizes l 2,1 norm minimization on processes of both label learning and feature learning, outliers and noise could be effectively handled and redundant or noisy features could be effectively reduced. Our method adopts the advantages of robust non-negative matrix factorization, local learning, and robust feature learning. In order to make RUFS be scalable, we design a (projected) limited-memory BFGS based iterative algorithm to efficiently solve the optimization problem of RUFS in terms of both memory consumption and computation complexity. Experimental results on different benchmark real world datasets show the promising performance of RUFS over the state-of-the-arts.
更多查看译文
关键词
local learning,robust feature learning,RUFS utilizes l2,feature selection,new unsupervised feature selection,noisy feature,traditional unsupervised feature selection,robust joint l2,robust non-negative matrix factorization,robust nonnegative matrix factorization,robust unsupervised feature selection
AI 理解论文
溯源树
样例
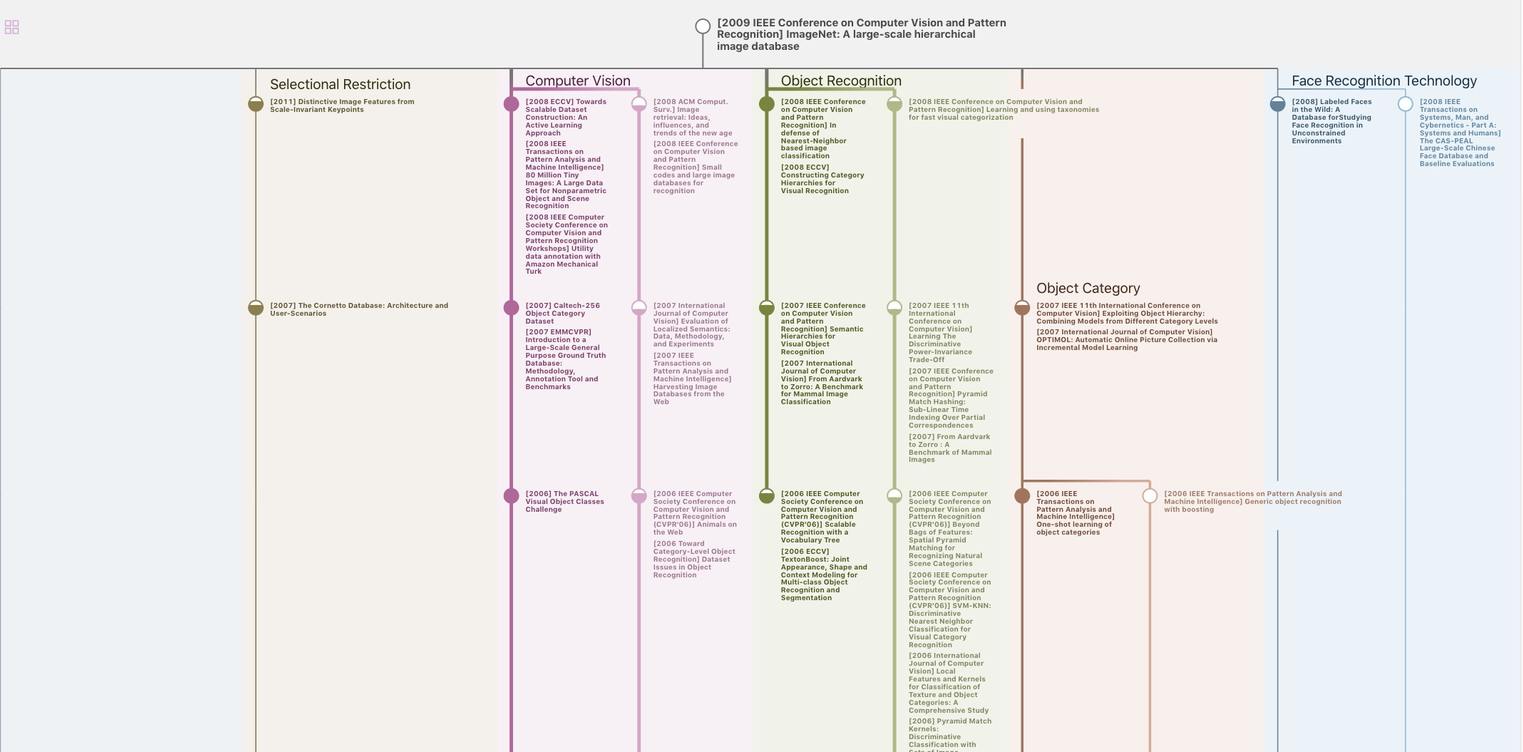
生成溯源树,研究论文发展脉络
Chat Paper
正在生成论文摘要