A neighborhood graph based approach to regional co-location pattern discovery: a summary of results.
GIS '11: 19th SIGSPATIAL International Conference on Advances in Geographic Information Systems Chicago Illinois November, 2011(2011)
摘要
Regional co-location patterns (RCPs) represent collections of feature types frequently located together in certain localities. For example, RCP < (Bar, Alcohol -- Crimes), Downtown >suggests that a co-location pattern involving alcohol-related crimes and bars is often localized to downtown regions. Given a set of Boolean feature types, their geo-located instances, a spatial neighbor relation, and a prevalence threshold, the RCP discovery problem finds all prevalent RCPs (pairs of co-locations and their prevalence localities). RCP discovery is important in many societal applications, including public safety, public health, climate science and ecology. The RCP discovery problem involves three major challenges: (a) an exponential number of subsets of feature types, (b) an exponential number of candidate localities and (c) a tradeoff between accurately modeling pattern locality and achieving computational efficiency. Related work does not provide computationally efficient methods to discover all interesting RCPs with their natural prevalence localities. To address these limitations, this paper proposes a neighborhood graph based approach that discovers all interesting RCPs and is aware of a pattern's prevalence localities. We identify partitions based on the pattern instances and neighbor graph. We introduce two new interest measures, a regional participation ratio and a regional participation index to quantify the strength of RCPs. We present two new algorithms, Pattern Space (PS) enumeration and Maximal Locality (ML) enumeration and show that they are correct and complete. Experiments using real crime datasets show that ML pruning outperforms PS enumeration.
更多查看译文
AI 理解论文
溯源树
样例
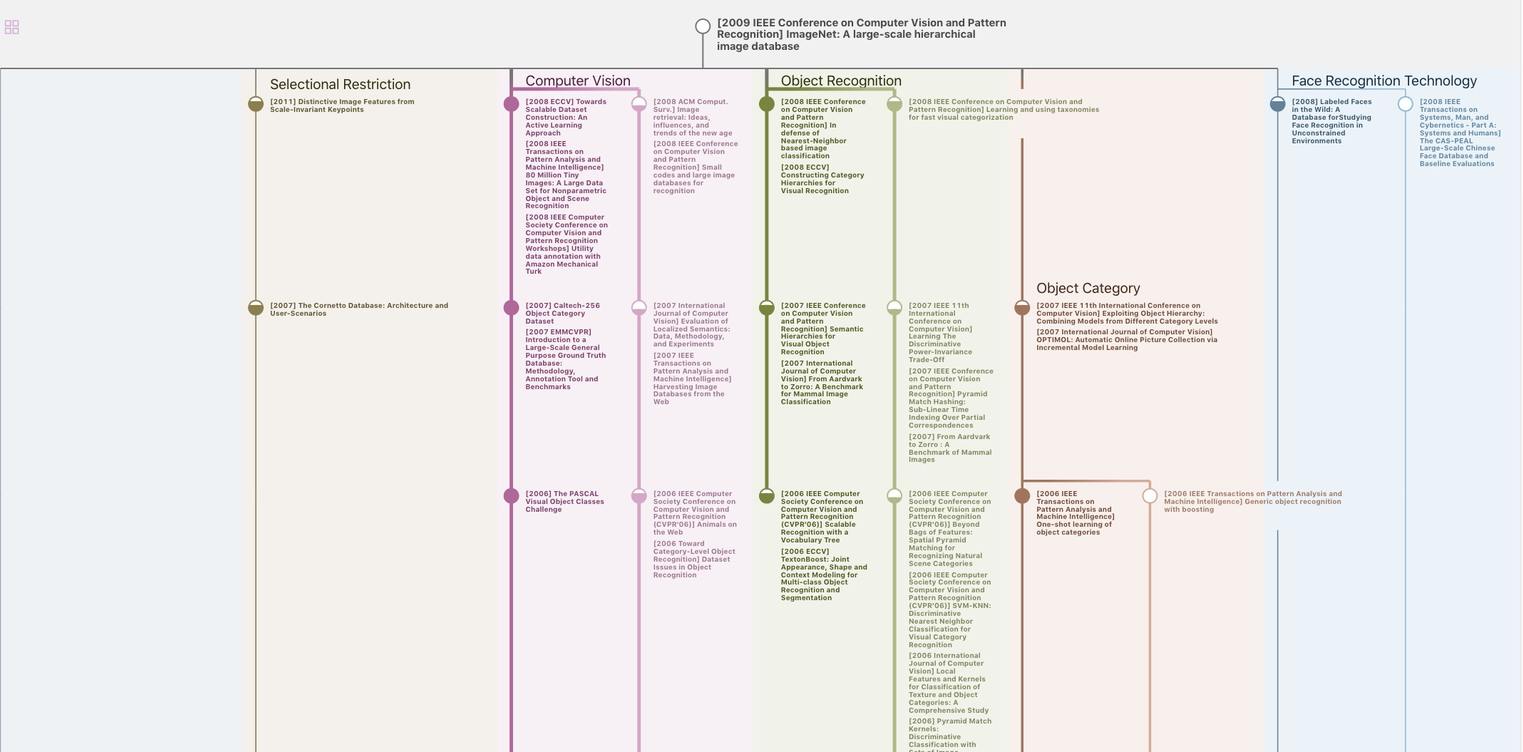
生成溯源树,研究论文发展脉络
Chat Paper
正在生成论文摘要