The Manifold Tangent Classifier.
NIPS(2011)
摘要
We combine three important ideas present in previous work for building classi- fiers: the semi-supervised hypothesis (the input distribution contains information about the classifier), the unsupervised manifold hypothesis (data density concen- trates near low-dimensional manifolds), and the manifold hypothesis for classifi- cation (different classes correspond to disjoint manifolds separated by low den- sity). We exploit a novel algorithm for capturing manifold structure (high-order contractive auto-encoders) and we show how it builds a topological atlas of charts, each chart being characterized by the principal singular vectors of the Jacobian of a representation mapping. This representation learning algorithm can be stacked to yield a deep architecture, and we combine it with a domain knowledge-free version of the TangentProp algorithm to encourage the classifier to be insensitive to local directions changes along the manifold. Record-breaking classification results are obtained.
更多查看译文
AI 理解论文
溯源树
样例
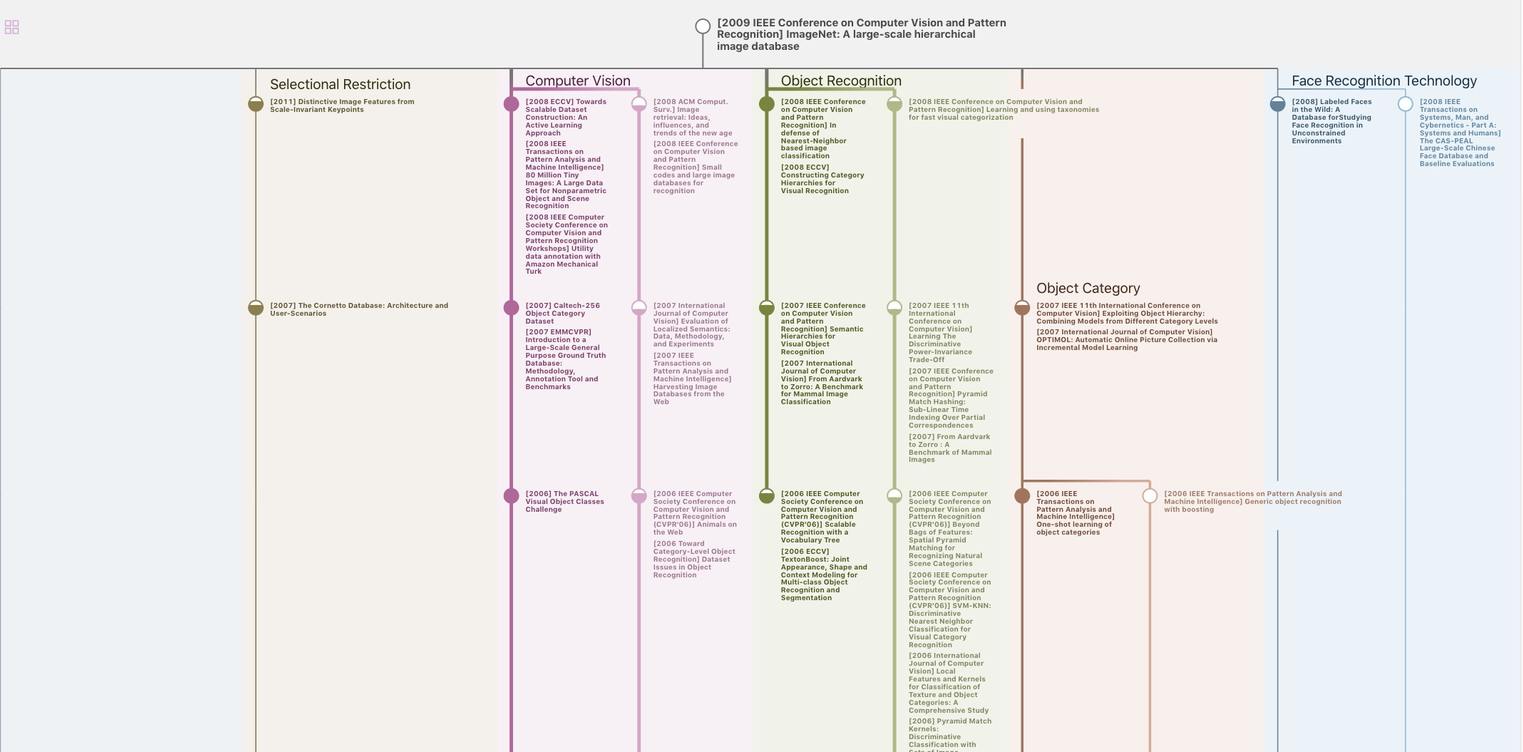
生成溯源树,研究论文发展脉络
Chat Paper
正在生成论文摘要