Semidefinite Ranking on Graphs
MLG(2007)
摘要
In this paper we tackle the problem of ranking the vertices in an undirected graph given some preference relation. We view the preferences as a preorder and aim at finding a linear order which conforms best with the undirected graph while at the same time violating as few as possible preference constraints. This problem has been tackled before using spectral relaxations. For many other problems, including combinatorial optimisation problems as well as clustering and classification problems, it has been observed that semidefinite relaxations oer several advantages over spectral ones. To solve ranking problems using semidefinite programming, we incorporate the preferences by enforcing certain angles between the embedding of the vertices. The final ordering is then obtained by the random projection method. Experiments on benchmark data sets show the expected improvements over spectral relaxations.
更多查看译文
关键词
linear order
AI 理解论文
溯源树
样例
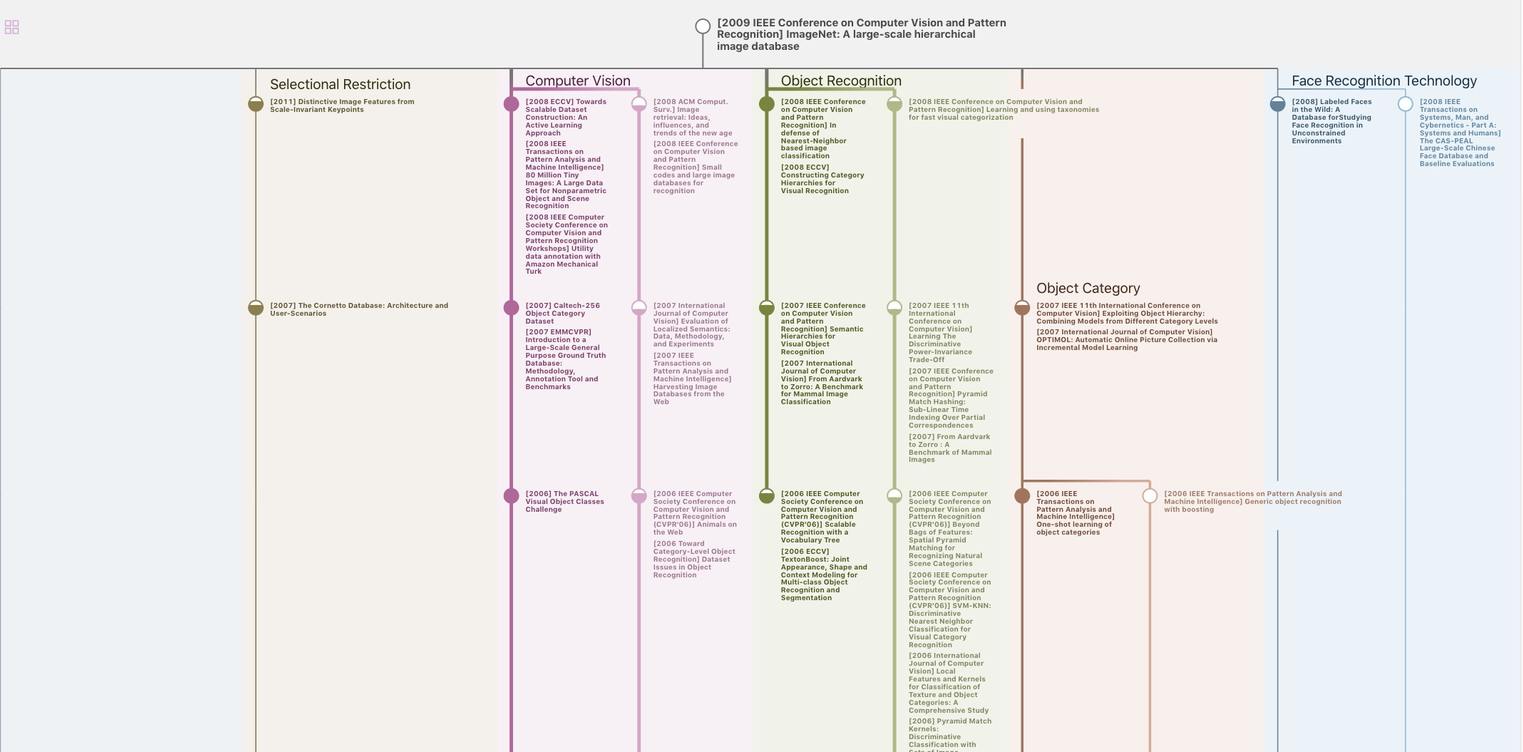
生成溯源树,研究论文发展脉络
Chat Paper
正在生成论文摘要