Ligand prediction from protein sequence and small molecule information using support vector machines and fingerprint descriptors.
JOURNAL OF CHEMICAL INFORMATION AND MODELING(2009)
摘要
Support vector machine (SVM) database search strategies are presented that aim at the identification of small molecule ligands for targets for which no ligand information is currently available. In pharmaceutical research and chemical biology, this situation is faced, for example, when studying orphan targets or newly identified members of protein families. To investigate methods for de novo ligand identification in the absence of known three-dimensional target structures or active molecules, we have focused on combining sequence and ligand information for closely and distantly related proteins. To provide a basis for these investigatiors, a set of I I protease targets from different families was assembled together with more than 2000 inhibitors directed against individual proteases. We have compared SVM approaches that combine protein sequence and ligand information in different ways and utilize 2D fingerprints as ligand descriptors. These methodologies were applied to search for inhibitors of individual proteases not taken into account during learning. A target sequence-ligand kernel and, in particular, a linear combination of multiple target-directed SVMs consistently identified inhibitors with high accuracy including test cases where homology-based similarity searching using data fusion and conventional SVM ranking nearly or completely failed. The SVM linear combination and target-ligand kernel methods described herein are intuitive and straightforward to adopt for ligand prediction against other targets.
更多查看译文
关键词
protein sequence,support vector machine
AI 理解论文
溯源树
样例
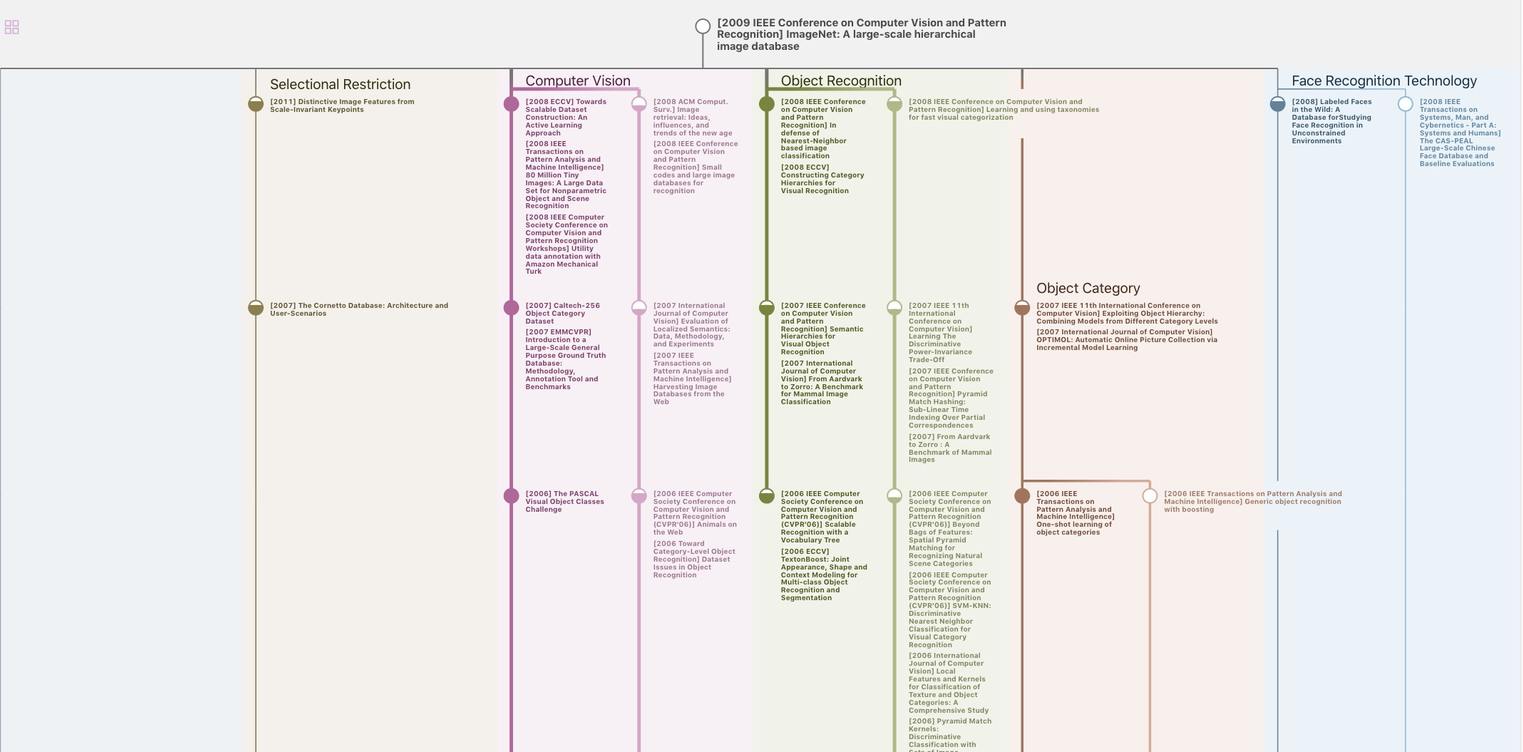
生成溯源树,研究论文发展脉络
Chat Paper
正在生成论文摘要