Multi-Label Associative Classification
DEMAND-DRIVEN ASSOCIATIVE CLASSIFICATION(2011)
摘要
A typical assumption in classification is that outputs are mutually exclusive, so that an input can be mapped to only one output (i.e., single-label classification). However, due to ambiguity or multiplicity, it is quite natural that many applications violate this assumption, allowing inputs to be mapped to multiple outputs simultaneously. Multi-label classification is a generalization of single-label classification, and its generality makes it much more difficult to solve. Despite its importance, research on multi-label classification is still lacking. Common approaches simply learn independent functions (Brinker et al. Unified model for multilabel classification and ranking. In: Proceedings of the European Conference on Artificial Intelligence, ECAI, pp. 489-493, 2006), not exploiting dependencies among outputs (Boutell et al. Learning multi-label scene classification. Pattern Recogn. 37(9), 1757-1771, 2004; Clare and King, Knowledge discovery in multi-label phenotype data. In: Proceedings of the European Conference on Principles and Practice of Knowledge Discovery in Databases (PKDD), Springer, pp. 42-53, 2001). Also, several small disjuncts may appear due to the possibly large number of combinations of outputs, and neglecting these small disjuncts may degrade classification performance (Proceedings of the International Conference on Information and Knowledge Management, CIKM, 2005; Proceedings of the Conference on Computer Vision and Pattern Recognition, 2006). In this chapter we extend demand-driven associative classification to multi-label classification.
更多查看译文
关键词
Multi-label classification,Ambiguity,Multiplicity,Independent outputs,Correlated outputs,Label focusing,Document categorization,Gene functional analysis
AI 理解论文
溯源树
样例
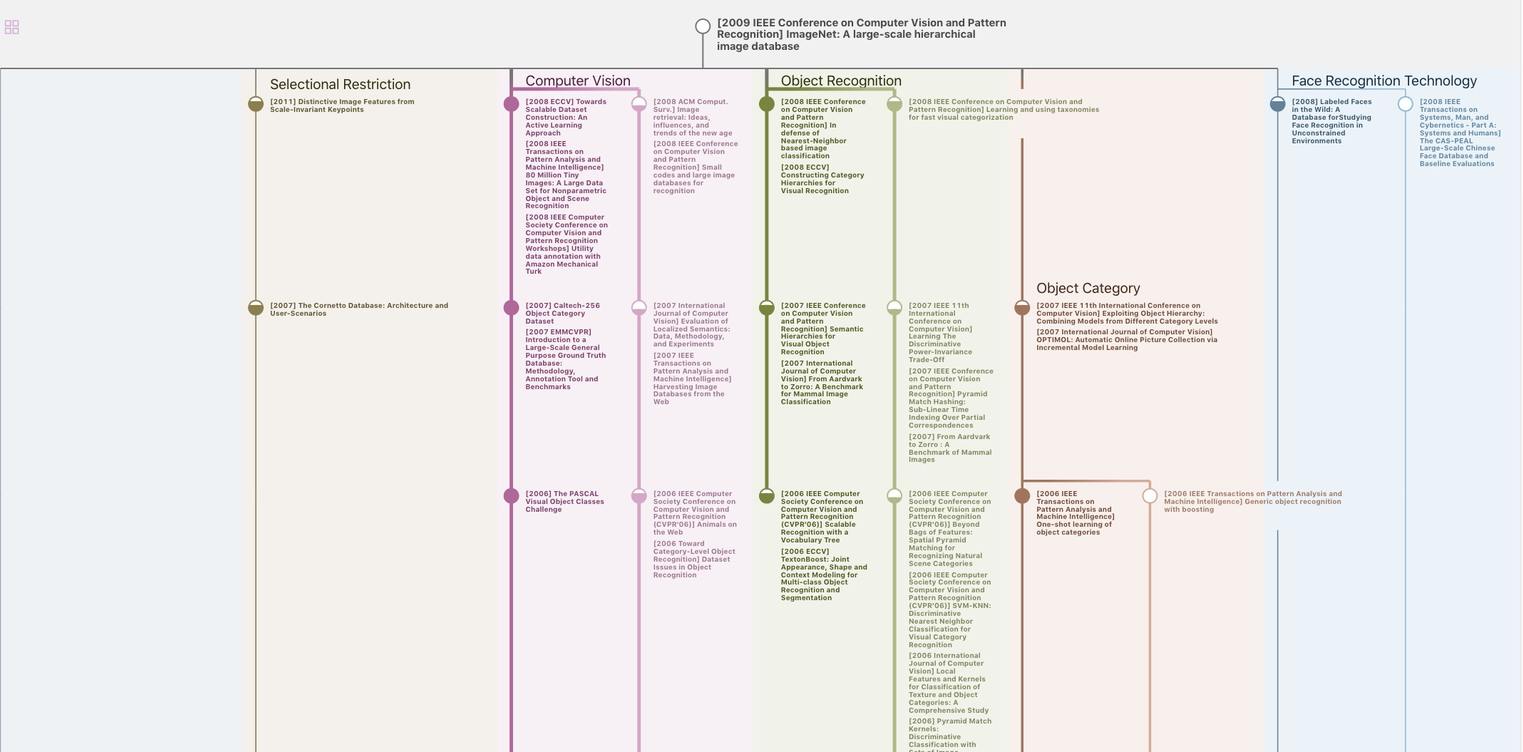
生成溯源树,研究论文发展脉络
Chat Paper
正在生成论文摘要