Explanation-Based Feature Construction
IJCAI(2007)
摘要
Choosing good features to represent objects can be crucial to the success of supervised machine learn- ing algorithms. Good high-level features are those that concentrate information about the classifica- tion task. Such features can often be constructed as non-linear combinations of raw or native input fea- tures such as the pixels of an image. Using many nonlinear combinations, as do SVMs, can dilute the classification information necessitating many train- ing examples. On the other hand, searching even a modestly-expressive space of nonlinear functions for high-information ones can be intractable. We describe an approach to feature construction where task-relevant discriminative features are automati- cally constructed, guided by an explanation-based interaction of training examples and prior domain knowledge. We show that in the challenging task of distinguishing handwritten Chinese characters, our automatic feature-construction approach performs particularly well on the most difficult and complex character pairs.
更多查看译文
关键词
training example,good feature,concentrate information,classification information,classification task,challenging task,explanation-based feature construction,nonlinear combination,nonlinear function,good high-level feature,automatic feature-construction approach,artificial intelligence,domain knowledge,machine learning,handwriting recognition,pattern recognition
AI 理解论文
溯源树
样例
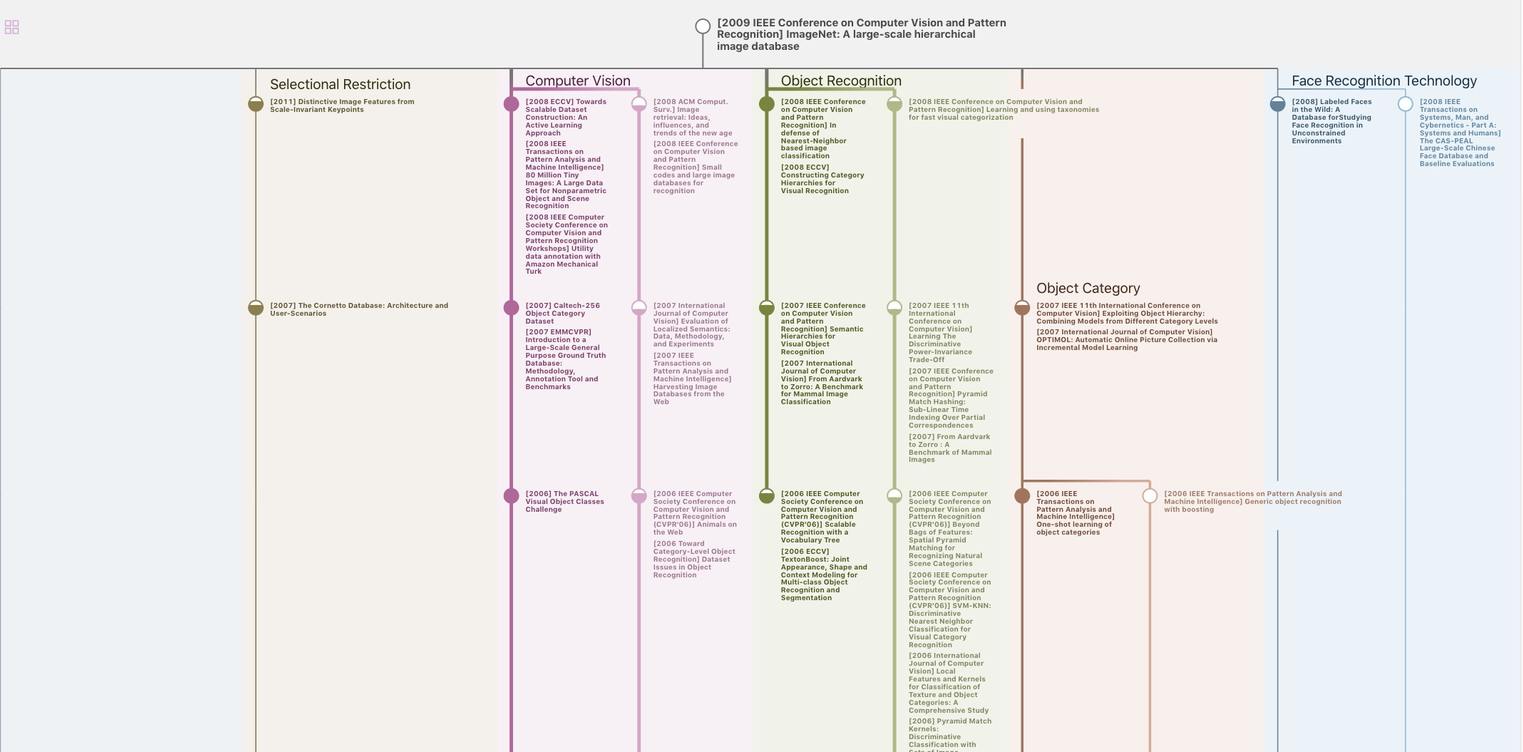
生成溯源树,研究论文发展脉络
Chat Paper
正在生成论文摘要