A New Perspective On Learning Linear Separators With Large Lqlp Margins
AISTATS(2014)
摘要
We give theoretical and empirical results that provide new insights into large margin learning. We prove a bound on the generalization error of learning linear separators with large LqLp margins (where L-q and L-p are dual norms) for any finite p >= 1. The bound leads to a simple data-dependent sufficient condition for fast learning in addition to extending and improving upon previous results. We also provide the first study that shows the benefits of taking advantage of margins with p < 2 over margins with p >= 2. Our experiments confirm that our theoretical results are relevant in practice.
更多查看译文
AI 理解论文
溯源树
样例
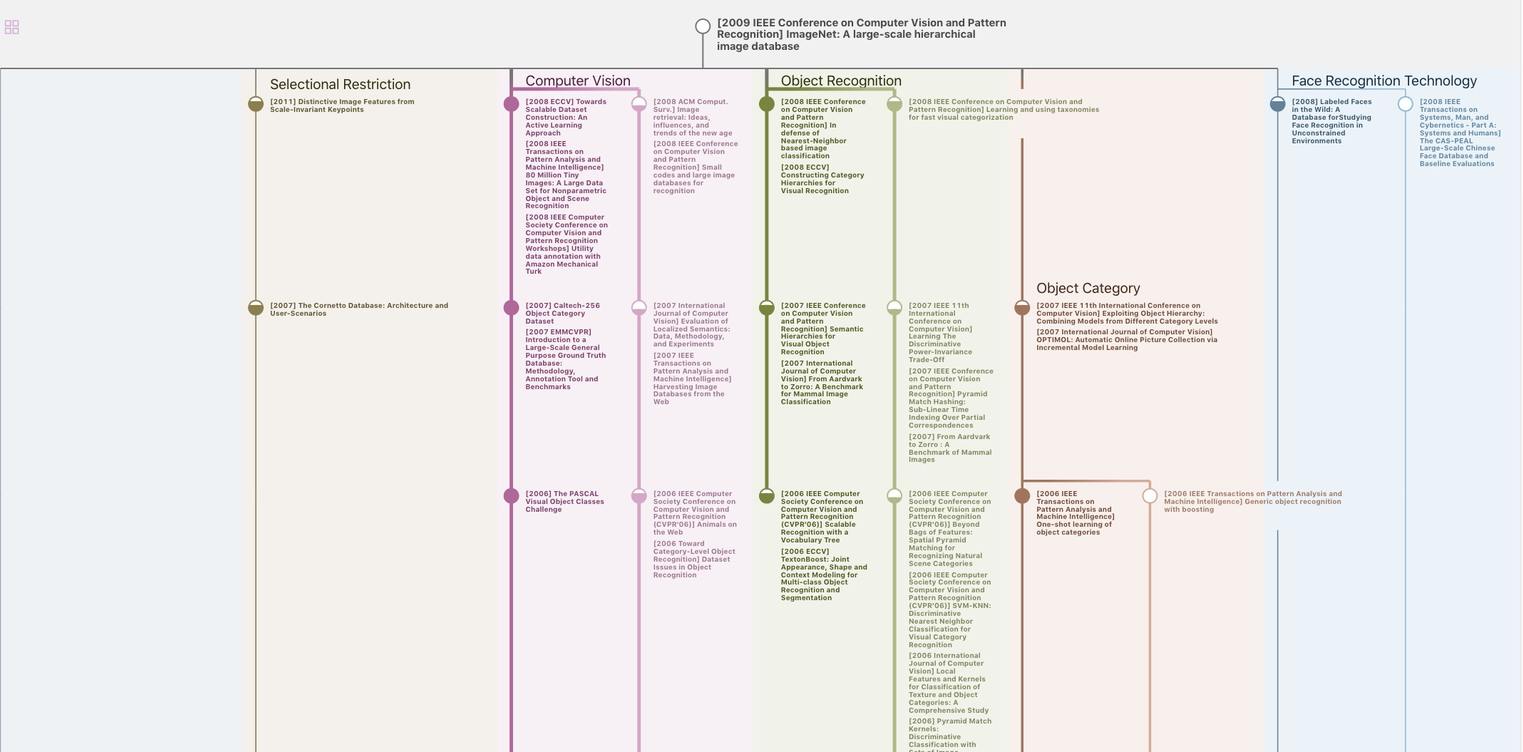
生成溯源树,研究论文发展脉络
Chat Paper
正在生成论文摘要