Learning Term-weighting Functions for Similarity Measures.
EMNLP '09: Proceedings of the 2009 Conference on Empirical Methods in Natural Language Processing: Volume 2 - Volume 2(2009)
摘要
Measuring the similarity between two texts is a fundamental problem in many NLP and IR applications. Among the existing approaches, the cosine measure of the term vectors representing the original texts has been widely used, where the score of each term is often determined by a TFIDF formula. Despite its simplicity, the quality of such cosine similarity measure is usually domain dependent and decided by the choice of the term-weighting function. In this paper, we propose a novel framework that learns the term-weighting function. Given the labeled pairs of texts as training data, the learning procedure tunes the model parameters by minimizing the specified loss function of the similarity score. Compared to traditional TFIDF term-weighting schemes, our approach shows a significant improvement on tasks such as judging the quality of query suggestions and filtering irrelevant ads for online advertising.
更多查看译文
关键词
term-weighting function,cosine similarity measure,similarity score,specified loss function,traditional TFIDF term-weighting scheme,TFIDF formula,cosine measure,IR application,existing approach,fundamental problem
AI 理解论文
溯源树
样例
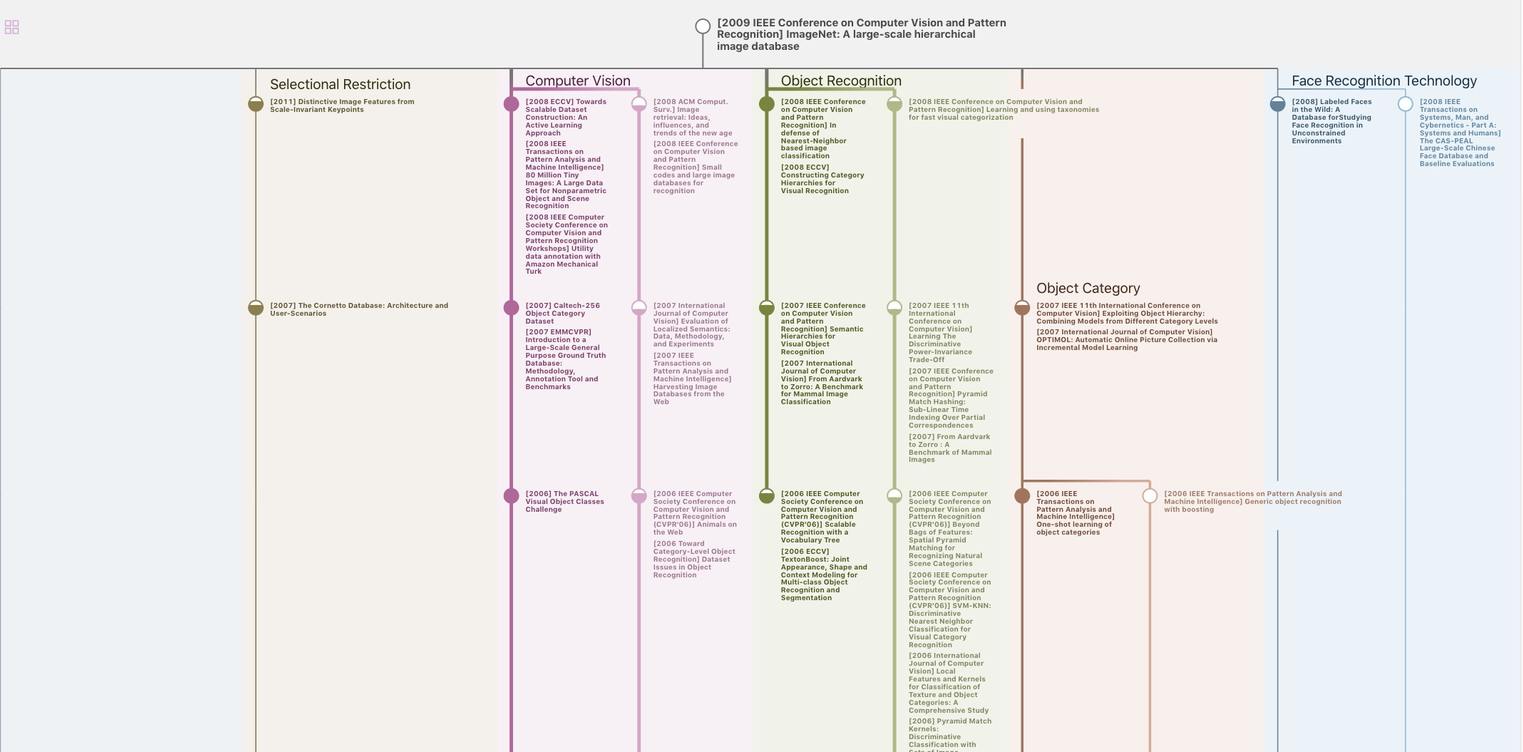
生成溯源树,研究论文发展脉络
Chat Paper
正在生成论文摘要