Minerva: Learning To Infer Network Path Properties
27TH IEEE CONFERENCE ON COMPUTER COMMUNICATIONS (INFOCOM), VOLS 1-5(2008)
摘要
Knowledge of the network path properties such as latency, hop count, loss and bandwidth is key to the performance of overlay networks, grids and p2p applications. Network operators also use these metrics for managing and diagnosing problems in their networks. However, the size of the Internet makes the task of measuring these metrics immensely difficult. A more scalable approach of inference and estimation of these metrics based on partial measurements has been recently adopted.Current inference approaches do not adapt to different network topologies and the evolution of the network over time. In this paper, we propose a novel learning based approach, called Minerva, for the inferencing of inter-node properties. Minerva uses partial measurements to create signature-like profiles for the participating nodes. These signatures are later used as input to a trained Bayesian network module to estimate the different network properties. We have built a system based on our approach and present performance results from real network measurements obtained from the Planet-Lab testbed. The sensitivity of the system to different parameters including training set, measurement overhead, and size of network have also been studied in this paper.
更多查看译文
关键词
bayesian network,bandwidth,estimation,internet,estimation theory,system testing,p2p,network topology,learning artificial intelligence,bayesian methods,engines,overlay network
AI 理解论文
溯源树
样例
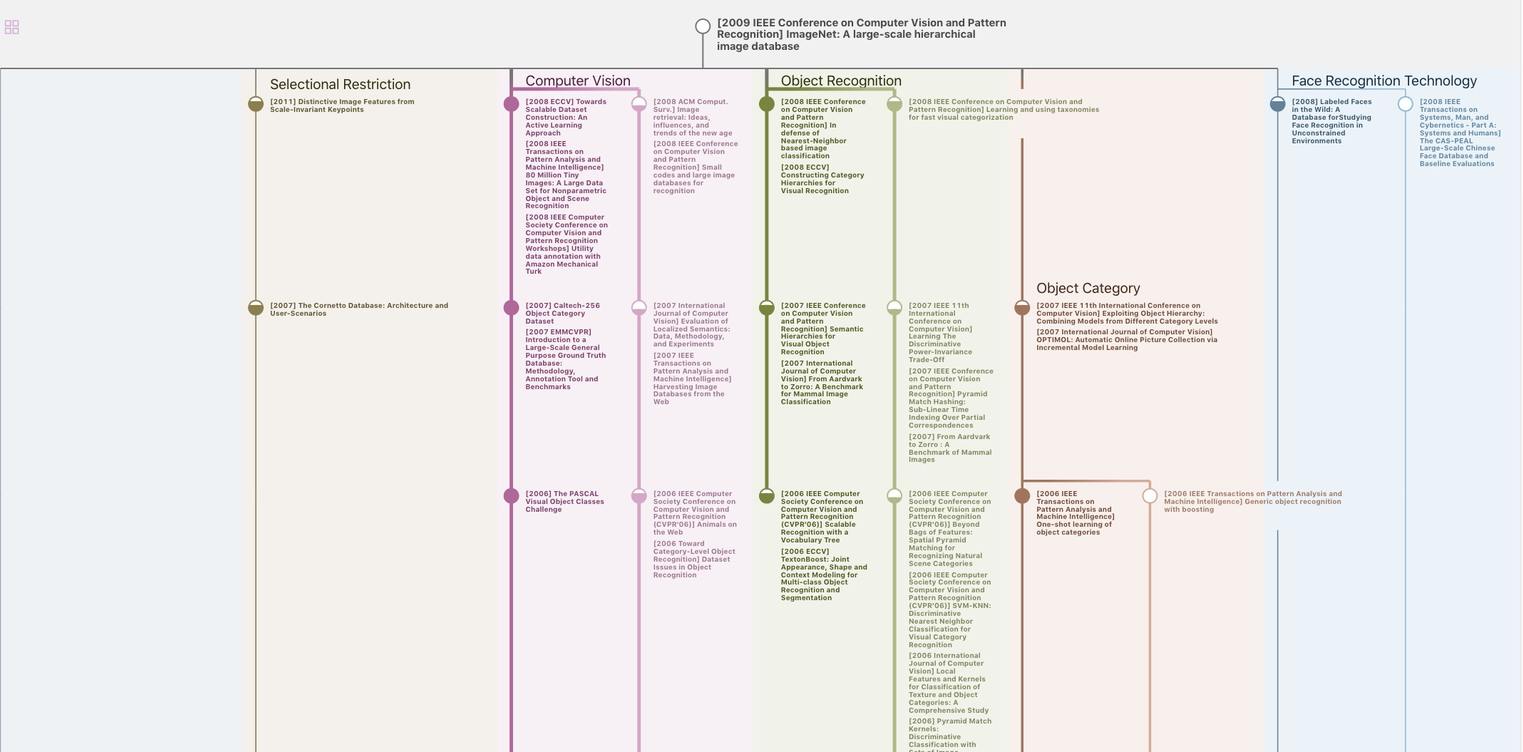
生成溯源树,研究论文发展脉络
Chat Paper
正在生成论文摘要