Joining feature-based and similarity-based pattern description paradigms for object detection.
ICPR(2012)
摘要
In pattern recognition, two of the main paradigms for describing objects are the feature-based and the (dis)similarity-based one. The former aims at encoding tangible features that characterize the object per-se. The latter gives a relational description of the object, considering the similarities with other reference entities. In this paper, we propose the marriage between these two philosophies: this is possible by considering an object as described by its local parts. Actually, object parts can be described by features, and structural information can be extracted considering the similarities between parts. We cast our intuition in an object detection framework, where we select HOG as feature and simple euclidean distances for the similarity computation. The results show how this hybrid representation outperforms the single paradigms, demonstrating their complementarity.
更多查看译文
关键词
feature extraction,image coding,object detection,Euclidean distances,HOG,feature-based pattern description paradigms,local parts,object detection,object parts,pattern recognition,similarity computation,similarity-based pattern description paradigms,structural information extraction,tangible feature encoding
AI 理解论文
溯源树
样例
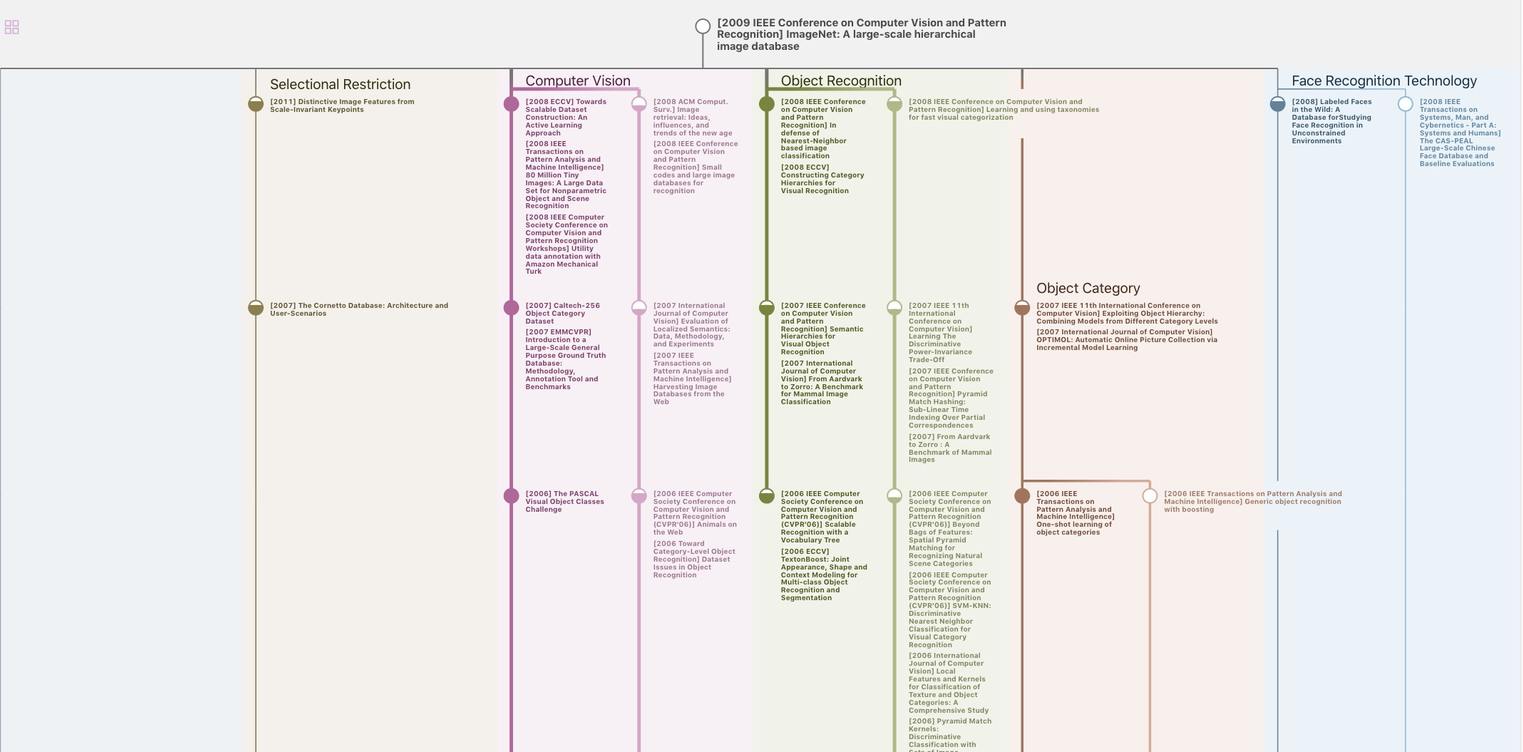
生成溯源树,研究论文发展脉络
Chat Paper
正在生成论文摘要