Multiclass Discriminative Fields for Parts-Based Object Detection
msra(2004)
摘要
In this paper, we present a discriminative framework for parts-based ob- ject detection based on the multiclass extensions of binary discriminative fields described in (1). These fields allow simultaneous discriminative modeling of the appearance of individual parts and the geometric rela- tionship between them. The conventional Markov Random Field (MRF) formulations cannot be used for this purpose because they do not allow the use of data while modeling interaction between labels which is crucial for enforcing geometric consistencies between parts. The proposed tech- nique can handle object deformations, occlusions and multiple-instance detection in a single trained model with no added computational efforts. The parameters of the field are learned using efficient maximum marginal approximations and inference is carried out using loopy belief propaga- tion. We demonstrate the efficacy of this approach through controlled preliminary experiments on rigid and deformable synthetic toy objects.
更多查看译文
关键词
discriminative model
AI 理解论文
溯源树
样例
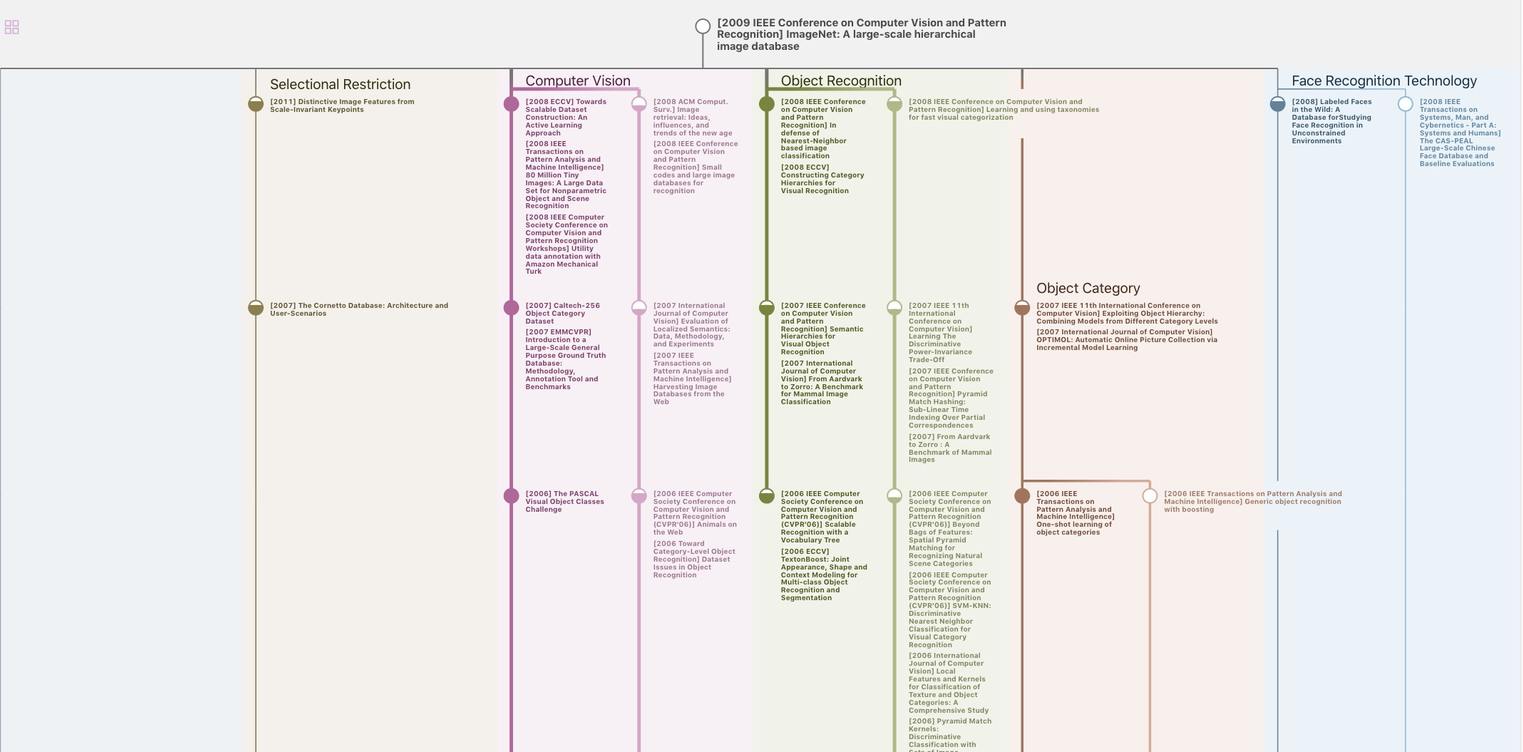
生成溯源树,研究论文发展脉络
Chat Paper
正在生成论文摘要