Reverse engineering gene regulatory network from microarray data using linear time-variant model
BMC Bioinformatics(2010)
摘要
Background Gene regulatory network is an abstract mapping of gene regulations in living cells that can help to predict the system behavior of living organisms. Such prediction capability can potentially lead to the development of improved diagnostic tests and therapeutics. DNA microarrays, which measure the expression level of thousands of genes in parallel, constitute the numeric seed for the inference of gene regulatory networks. In this paper, we have proposed a new approach for inferring gene regulatory networks from time-series gene expression data using linear time-variant model. Here, Self-Adaptive Differential Evolution , a versatile and robust Evolutionary Algorithm, is used as the learning paradigm. Results To assess the potency of the proposed work, a well known nonlinear synthetic network has been used. The reconstruction method has inferred this synthetic network topology and the associated regulatory parameters with high accuracy from both the noise-free and noisy time-series data. For validation purposes, the proposed approach is also applied to the simulated expression dataset of cAMP oscillations in Dictyostelium discoideum and has proved it's strength in finding the correct regulations. The strength of this work has also been verified by analyzing the real expression dataset of SOS DNA repair system in Escherichia coli and it has succeeded in finding more correct and reasonable regulations as compared to various existing works. Conclusion By the proposed approach, the gene interaction networks have been inferred in an efficient manner from both the synthetic, simulated cAMP oscillation expression data and real expression data. The computational time of this approach is also considerably smaller, which makes it to be more suitable for larger network reconstruction. Thus the proposed approach can serve as an initiate for the future researches regarding the associated area.
更多查看译文
关键词
Mean Square Error,Differential Evolution,Gene Regulatory Network,False Positive Regulation,Time Series Expression Data
AI 理解论文
溯源树
样例
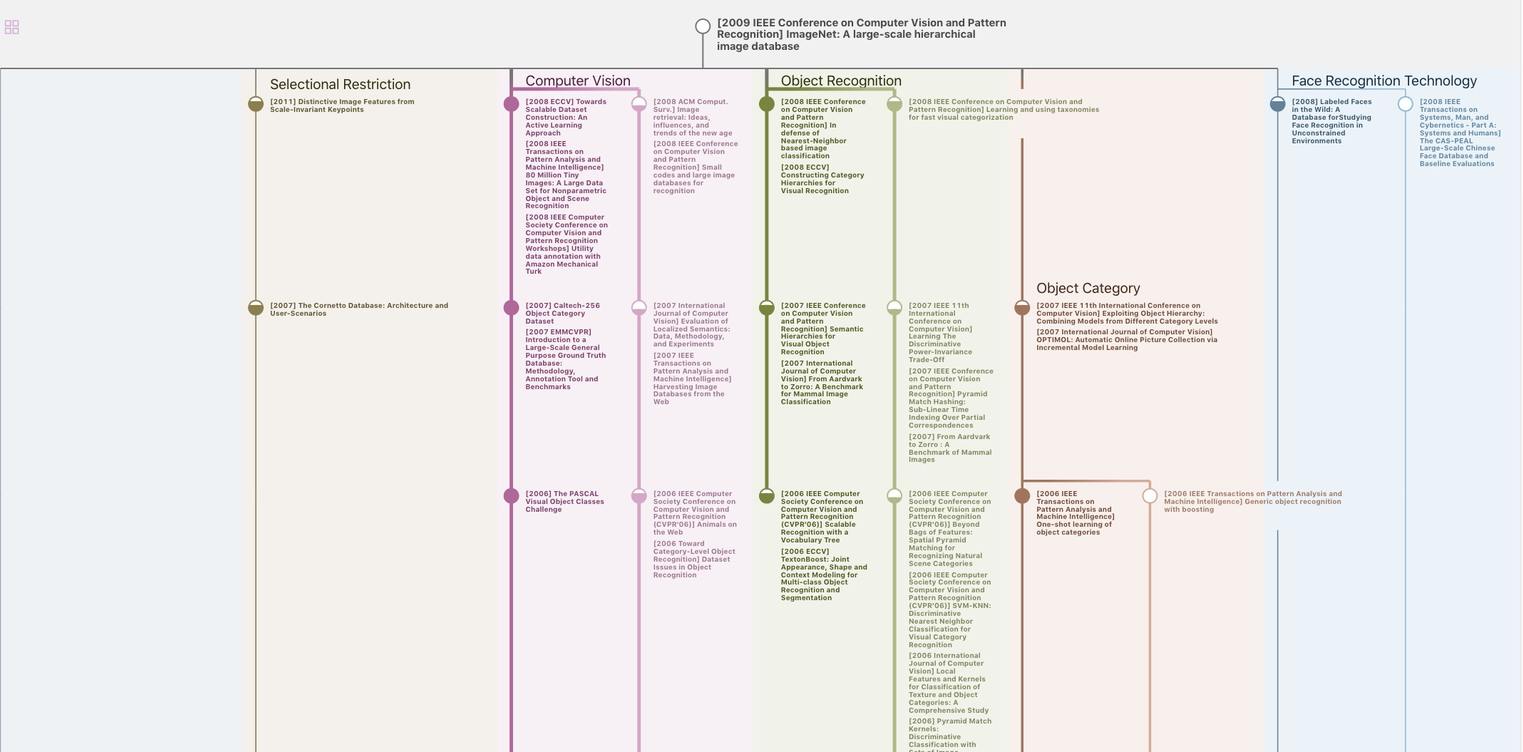
生成溯源树,研究论文发展脉络
Chat Paper
正在生成论文摘要