Enhanced Q-Learning Algorithm For Dynamic Power Management With Performance Constraint
Design, Automation, and Test in Europe(2010)
摘要
This paper presents a novel power management techniques based on enhanced Q-learning algorithms. By exploiting the submodularity and monotonic structure in the cost function of a power management system, the enhanced Q-learning algorithm is capable of exploring ideal trade-offs in the power-performance design space and converging to a better power management policy. We further propose a linear adaption algorithm that adapts the Lagrangian multiplier. to search for the power management policy that minimizes the power consumption while delivering the exact required performance. Experimental results show that, comparing to the existing expert-based power management, the proposed Q-learning based power management achieves up to 30% and 60% reduction in power saving for synthetic workload and real workload, respectively while in average maintain a performance within 7% variation of the given constraint.
更多查看译文
关键词
computer peripheral equipment,performance evaluation,Lagrangian multiplier,Q-learning algorithm,dynamic power management,linear adaption algorithm,performance constraint,power consumption,
AI 理解论文
溯源树
样例
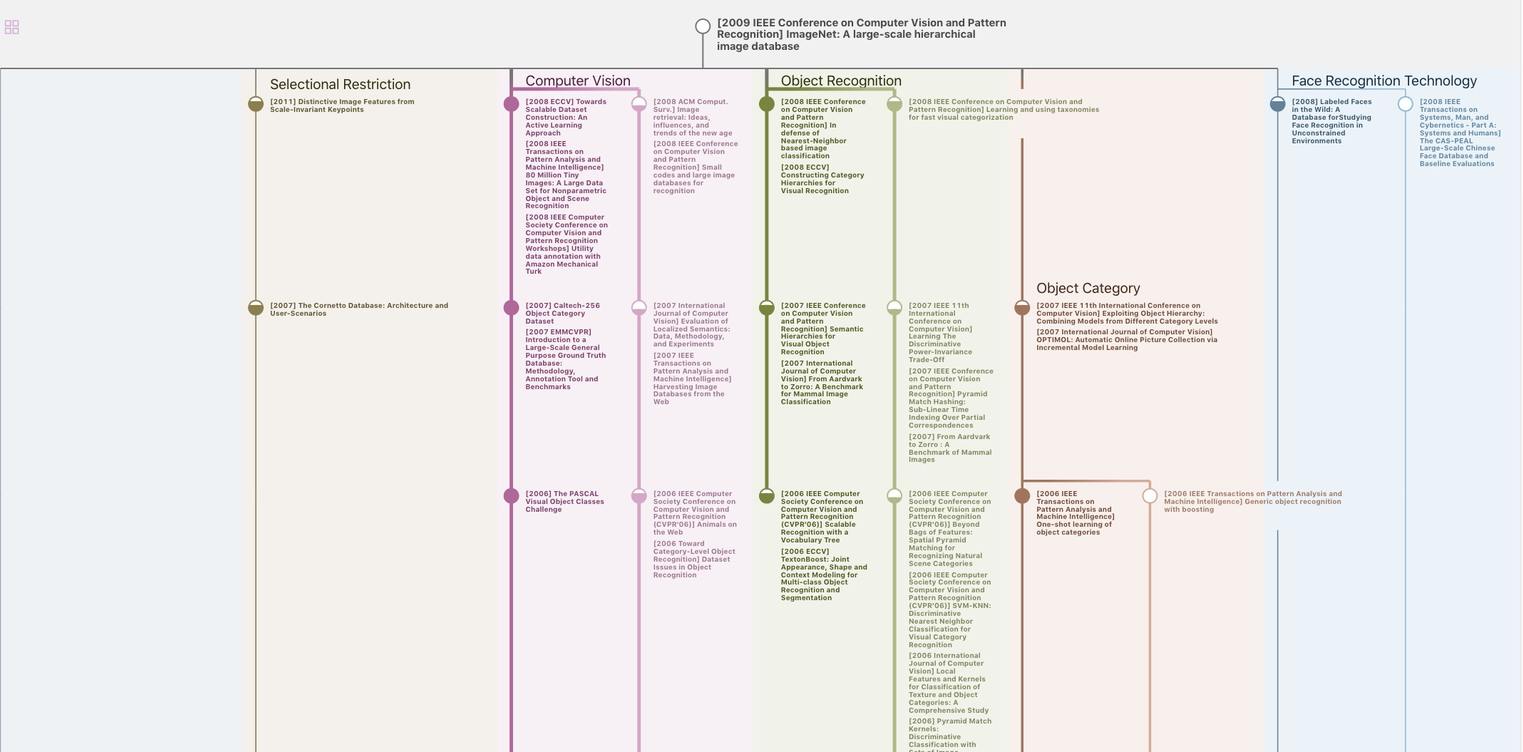
生成溯源树,研究论文发展脉络
Chat Paper
正在生成论文摘要