Combining Acoustic Embeddings And Decoding Features For End-Of-Utterance Detection In Real-Time Far-Field Speech Recognition Systems
2018 IEEE INTERNATIONAL CONFERENCE ON ACOUSTICS, SPEECH AND SIGNAL PROCESSING (ICASSP)(2018)
摘要
We present an end-of-utterance detector for real-time automatic speech recognition in far-field scenarios. The proposed system consists of three components: a long short-term memory (LSTM) neural network trained on acoustic features, an LSTM trained on 1-best recognition hypotheses of the automatic speech recognition (ASR) decoder, and a feed-forward deep neural network (DNN) combining embeddings derived from both LSTMs with pause duration features from the ASR decoder. At inference time, lower and upper latency (pause duration) bounds act as safeguards. Within the latency bounds, the utterance end-point is triggered as soon as the DNN posterior reaches a tuned threshold. Our experimental evaluation is carried out on real recordings of natural human interactions with voice-controlled far-field devices. We show that the acoustic embeddings are the single most powerful feature and particularly suitable for cross-lingual applications. We furthermore show the benefit of ASR decoder features, especially as a low cost alternative to ASR hypothesis embeddings.
更多查看译文
关键词
end-pointing, end-of-query detection, turn taking, dialog modeling, online speech recognition
AI 理解论文
溯源树
样例
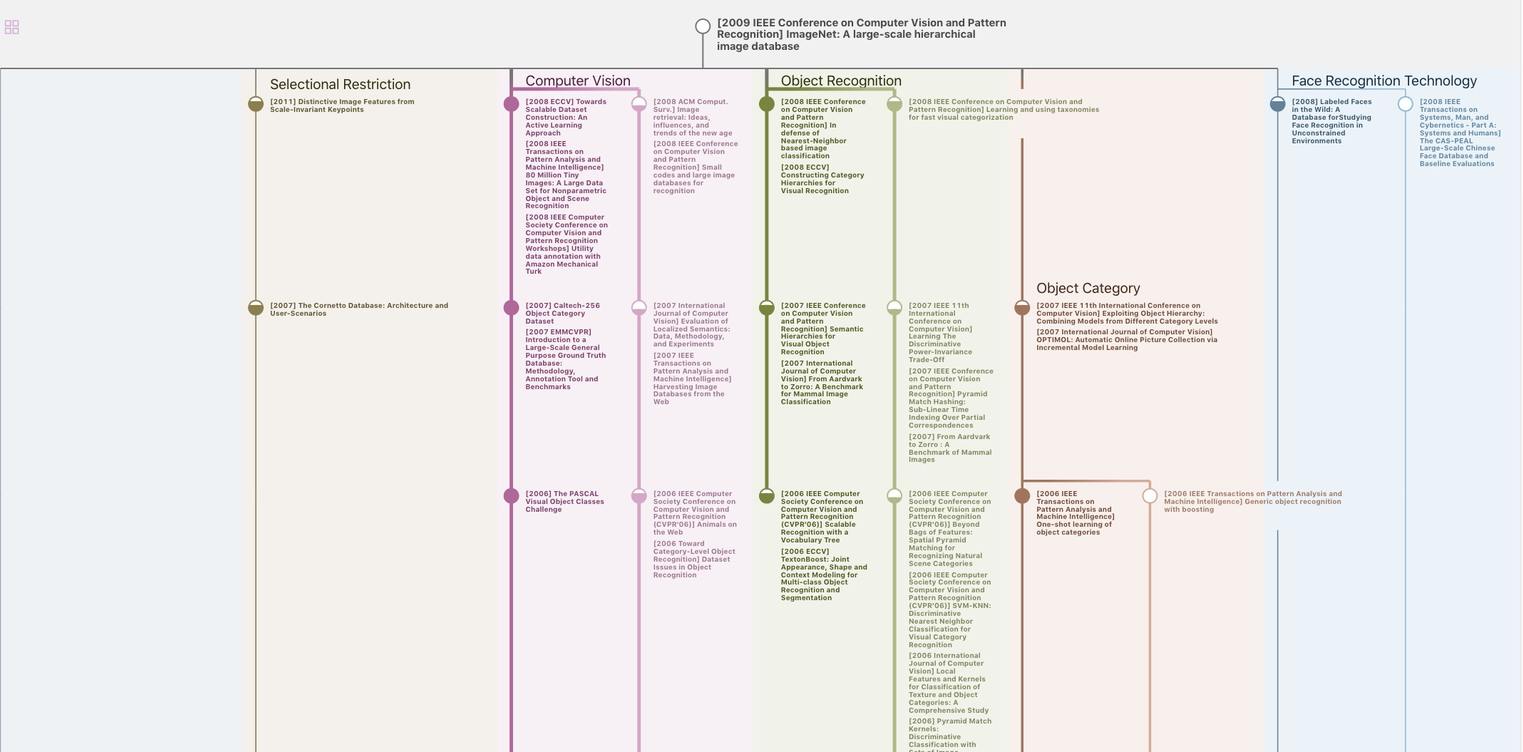
生成溯源树,研究论文发展脉络
Chat Paper
正在生成论文摘要