A connection between score matching and denoising autoencoders.
Neural Computation(2011)
摘要
Denoising autoencoders have been previously shown to be competitive alternatives to restricted Boltzmann machines for unsupervised pretraining of each layer of a deep architecture. We show that a simple denoising autoencoder training criterion is equivalent to matching the score (with respect to the data) of a specific energy-based model to that of a nonparametric Parzen density estimator of the data. This yields several useful insights. It defines a proper probabilistic model for the denoising autoencoder technique, which makes it in principle possible to sample from them or rank examples by their energy. It suggests a different way to apply score matching that is related to learning to denoise and does not require computing second derivatives. It justifies the use of tied weights between the encoder and decoder and suggests ways to extend the success of denoising autoencoders to a larger family of energy-based models.
更多查看译文
关键词
Denoising autoencoders,denoising autoencoder technique,simple denoising autoencoder training,energy-based model,proper probabilistic model,score matching,specific energy-based model,competitive alternative,deep architecture,larger family,denoising autoencoders
AI 理解论文
溯源树
样例
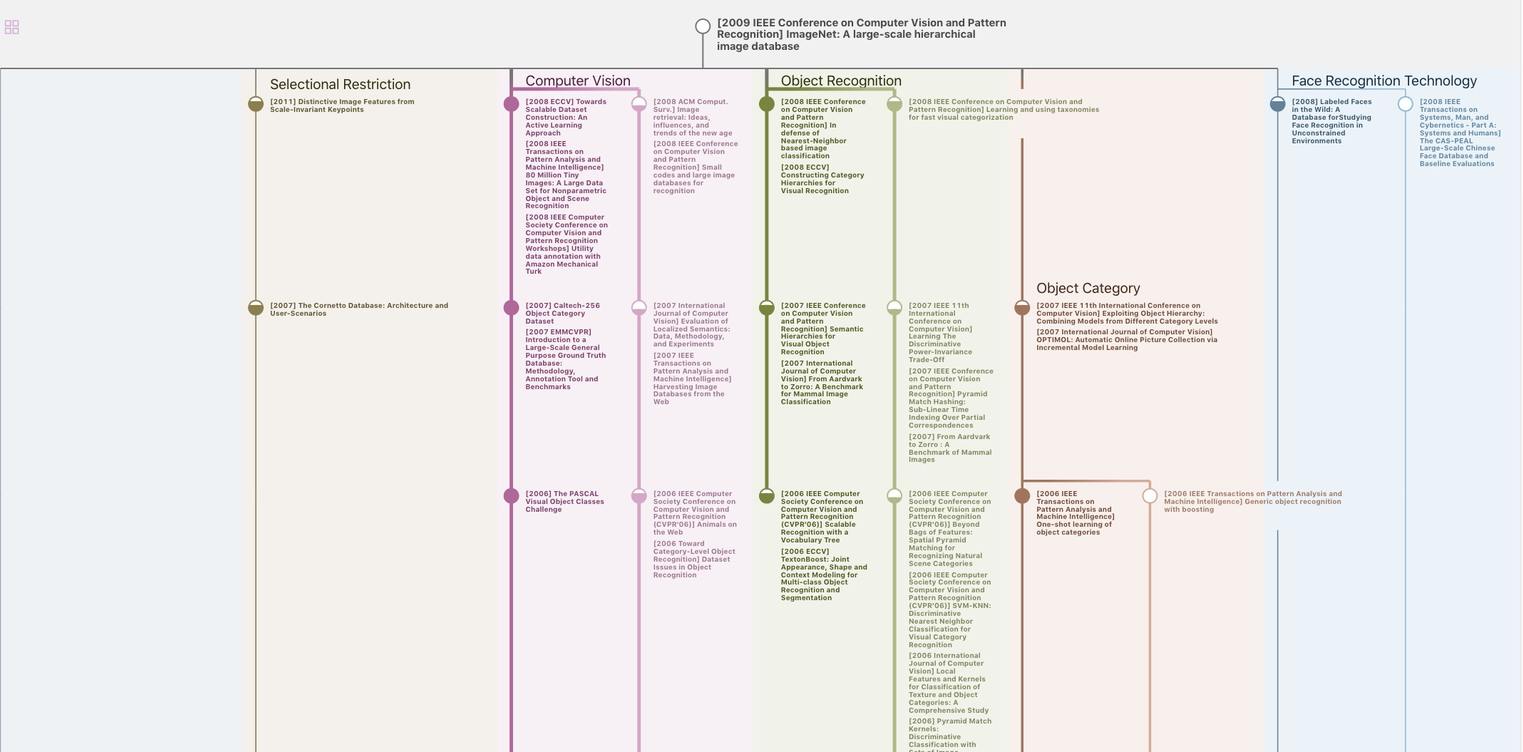
生成溯源树,研究论文发展脉络
Chat Paper
正在生成论文摘要