Particle swarm optimizer with adaptive tabu and mutation: A unified framework for efficient mutation operators
TAAS(2010)
摘要
Evolutionary Algorithms (EAs) and Swarm Intelligence (SI) are widely used to tackle black-box global optimization problems when no prior knowledge is available. In order to increase search diversity and avoid stagnation in local optima, the mutation operator was introduced and has been extensively studied in EAs and SI-based algorithms. However, the performance after introducing mutation can be affected in many aspects and the parameters used to perform mutations are very hard to determine. For the purpose of developing efficient mutation operators, this article proposes a unified tabu and mutation framework with parameter adaptations in the context of the Particle Swarm Optimizer (PSO). The proposed framework is a significant extension of our preliminary work [Wang et al. 2007]. Empirical studies on 25 benchmark functions indicate that under the proposed framework: (1) excellent performance can be achieved even with a small number of mutations; (2) the derived algorithm consistently performs well on diverse types of problems and overall performance even surpasses the state-of-the-art PSO variants and representative mutation-based EAs; and (3) fast convergence rates can be preserved despite the use of a long jump mutation operator (the Cauchy mutation).
更多查看译文
关键词
excellent performance,unified framework,mutation framework,efficient mutation operator,evolutionary algorithm,overall performance,long jump mutation operator,adaptive tabu,Cauchy mutation,proposed framework,swarm intelli- gence,Particle Swarm Optimizer,parameter adaptation,additional key words and phrases: global optimization,particle swarm optimizer,representative mutation-based EAs,mutation operator
AI 理解论文
溯源树
样例
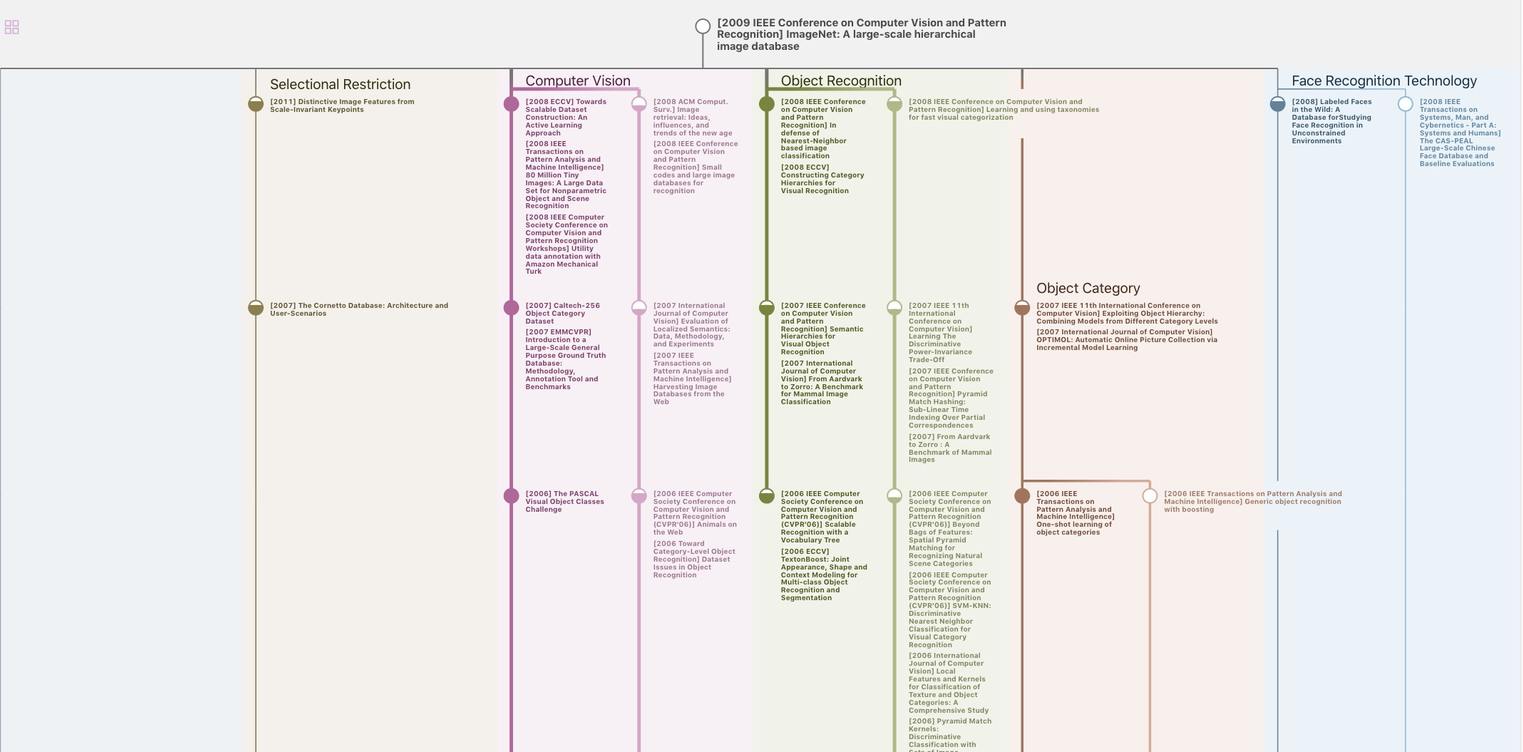
生成溯源树,研究论文发展脉络
Chat Paper
正在生成论文摘要