ON THE FIDELITY OF SAFs: CAN PERFORMANCE DATA HELP?
msra(2006)
摘要
A recent report developed by the National Research Council (NRC) for the Defense Modeling and Simulation Office (DMSO) encourages the use of real world, war-gaming, and laboratory data in support of the development and validation of human behavioral models for military simulations. Also encouraged in this report is the use of interdisciplinary teams embracing the disciplines of the psychological, computer, and military sciences to create such models. This paper describes the use of an artificial intelligence modeling framework, observational learning, to support these objectives. This framework combines the research methods of experimental psychology with the machine learning methods of computer science to develop behavioral models from data generated by military experts participating in live and/or simulated exercises. To date, research has demonstrated that behavioral models developed through this framework can be integrated into popular Semi-Automated Force (SAF) systems to enhance their performance. However, there has been no known investigation as to what the benefits of this approach are with respect to behavioral model fidelity. This paper introduces the interdisciplinary nature of observational learning by briefly surveying its history with respect to computer science and psychology and by illustrating how it can be used in conjunction with military experts. Next, this paper examines experimental evidence to determine whether a significant difference exists between SAF performance and human performance for a low-level, skill task. Finally, this paper demonstrates how behavioral models developed through human performance data generated by military SMEs can be used in conventional SAF systems to make SAF performance more "human-like".
更多查看译文
AI 理解论文
溯源树
样例
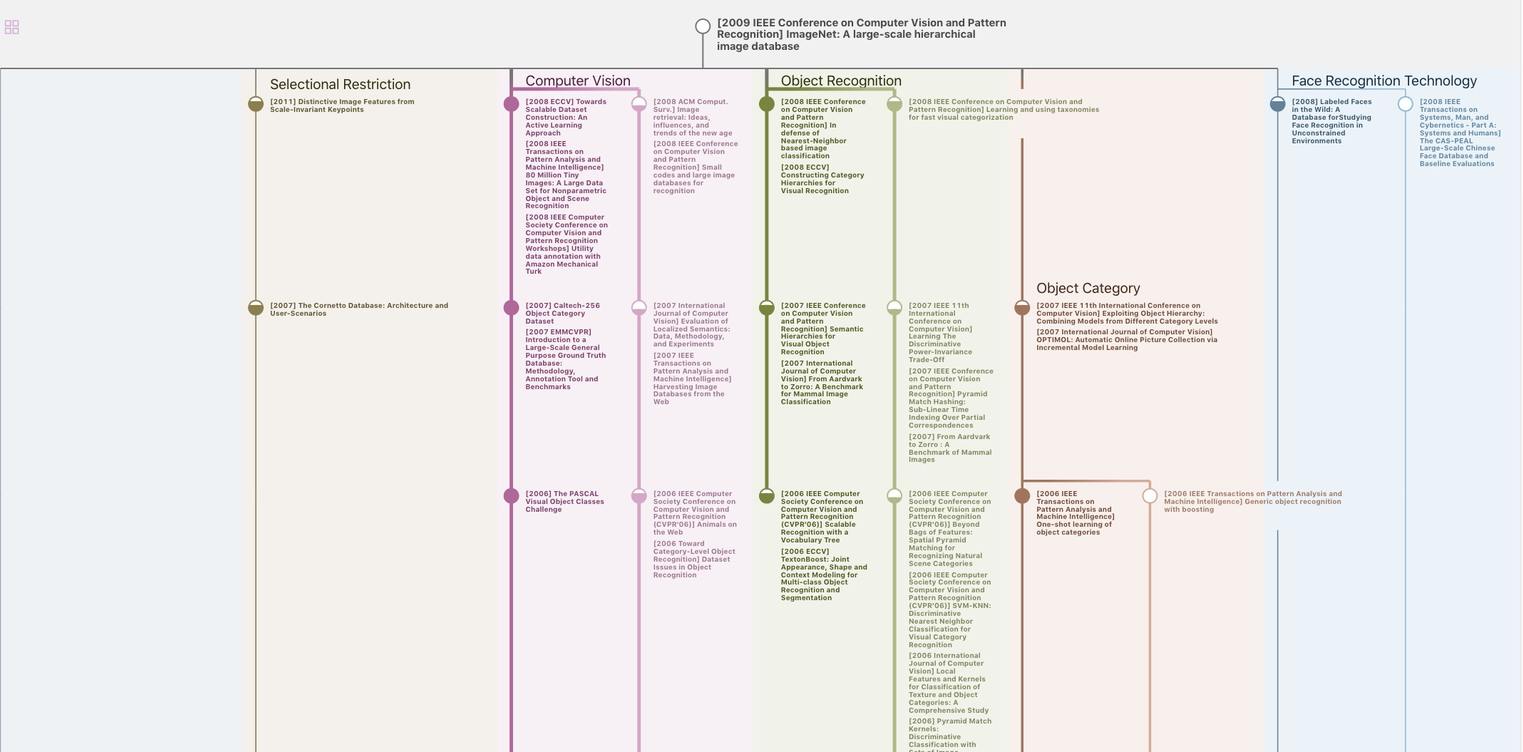
生成溯源树,研究论文发展脉络
Chat Paper
正在生成论文摘要