Predicting the parameters of a prostate IMRT objective function based on dose statistics under fixed parameter settings
Medical Physics(2007)
摘要
Purpose: To simplify the trial‐and‐error process of adjusting objective function parameters (e.g. weights, dose limits) in prostate IMRT planning, we present a feasibility study showing that machine learning followed by a sensitivity‐driven greedy search can quickly and automatically determine parameters that lead to a plan meeting the clinical requirements. Method and Materials: The training database is composed of 39 plans treated effectively under a five‐field 8640cGy prostate IMRT protocol. For each plan, the output features include the clinical parameter values, and the input features include simple dose statistics resulting from optimizing the objective function starting from several fixed parameter values. Given the same parameters, these dose statistics vary between patients, representing different degrees of competition between the PTV and OARs. We predict a new plan based on the 3 plans in the training database that have the most similar input features. Starting from such a “pre‐plan”, a sensitivity‐based automatic parameter search is applied to improve the plan's deficiencies. Results: Experiments on the 39‐patient dataset showed that a clinically acceptable prostate IMRT plan could be automatically determined within 15 iterations of optimization (using simplified dose calculations). Compared with the clinical ground truth, the mean absolute differences for PTV V95, PTV Dmin, rectwall V54 and rectwall Dmax are 1.1%, 2.4%, 2.6% and 0.9%, respectively. The adjusted plans met DVH constraints defining clinical acceptability and were comparable to manually‐determined plans. Conclusion: Prior knowledge gained through historical plans can facilitate rapid parameter selection for dose‐volume‐based IMRT objectives. The combination of machine learning and automatic sensitivity‐based parameter searching may also be applicable to other types of objective functions, and has the potential to ease the manual burden of IMRT planning in more complex sites. Supported by National Cancer Institute grant 5P01CA59017‐13 and the NSF Center for Subsurface Sensing and Imaging Systems, grant EEC‐9986821.
更多查看译文
关键词
objective function
AI 理解论文
溯源树
样例
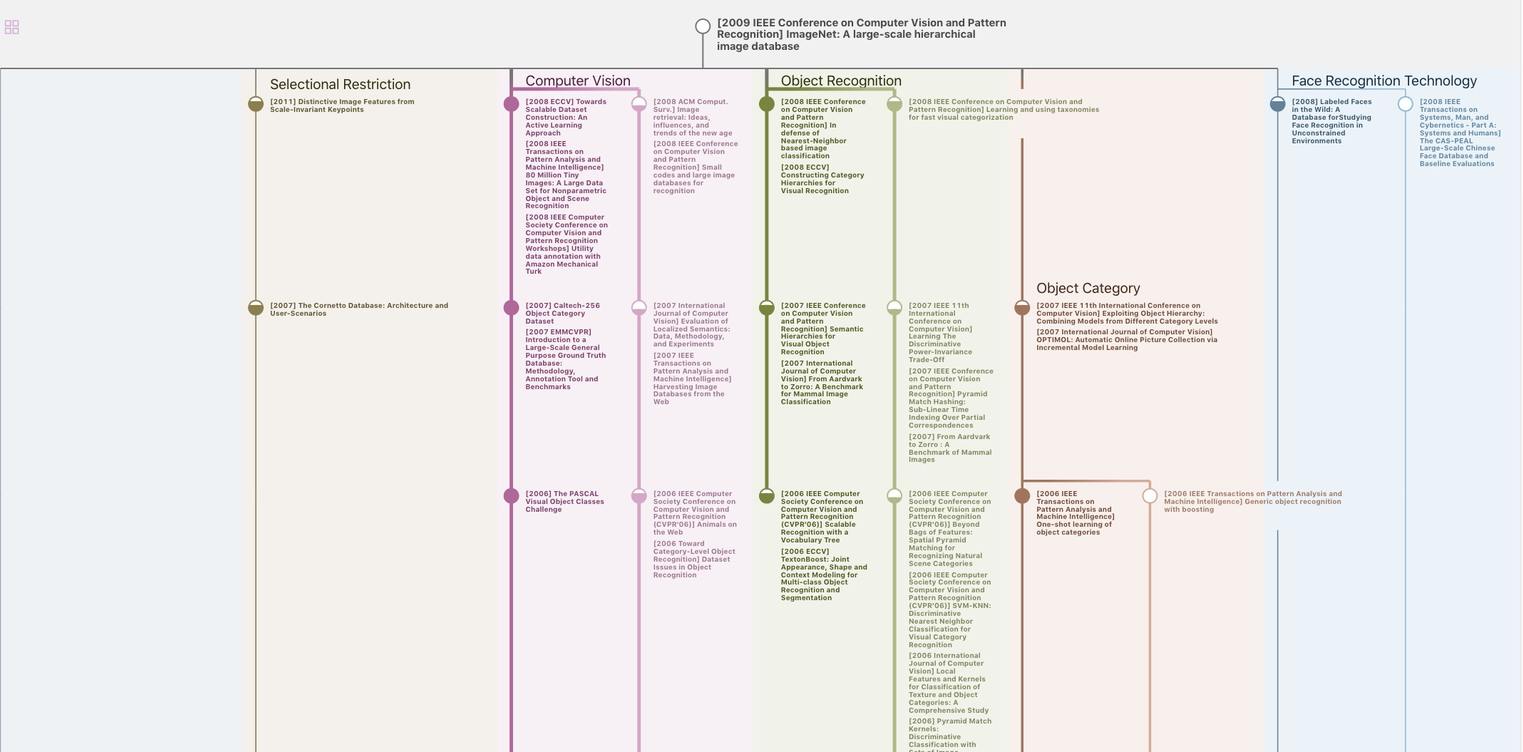
生成溯源树,研究论文发展脉络
Chat Paper
正在生成论文摘要