A latent class MDS model with spatial constraints for non-stationary spatial covariance estimation
Stochastic Environmental Research and Risk Assessment(2008)
摘要
Multidimensional scaling (MDS) has played an important role in non-stationary spatial covariance structure estimation and in analyzing the spatiotemporal processes underlying environmental studies. A combined cluster-MDS model, including geographical spatial constraints, has been previously proposed by the authors to address the estimation problem in oversampled domains in a least squares framework. In this paper is formulated a general latent class model with spatial constraints that, in a maximum likelihood framework, allows to partition the sample stations into classes and simultaneously to represent the cluster centers in a low-dimensional space, while the stations and clusters retain their spatial relationships. A model selection strategy is proposed to determine the number of latent classes and the dimensionality of the problem. Real and artificial data sets are analyzed to test the performance of the model.
更多查看译文
关键词
Multidimensional scaling,k-means clustering,Analysis of dispersion,Spatiotemporal processes,Nonstationarity
AI 理解论文
溯源树
样例
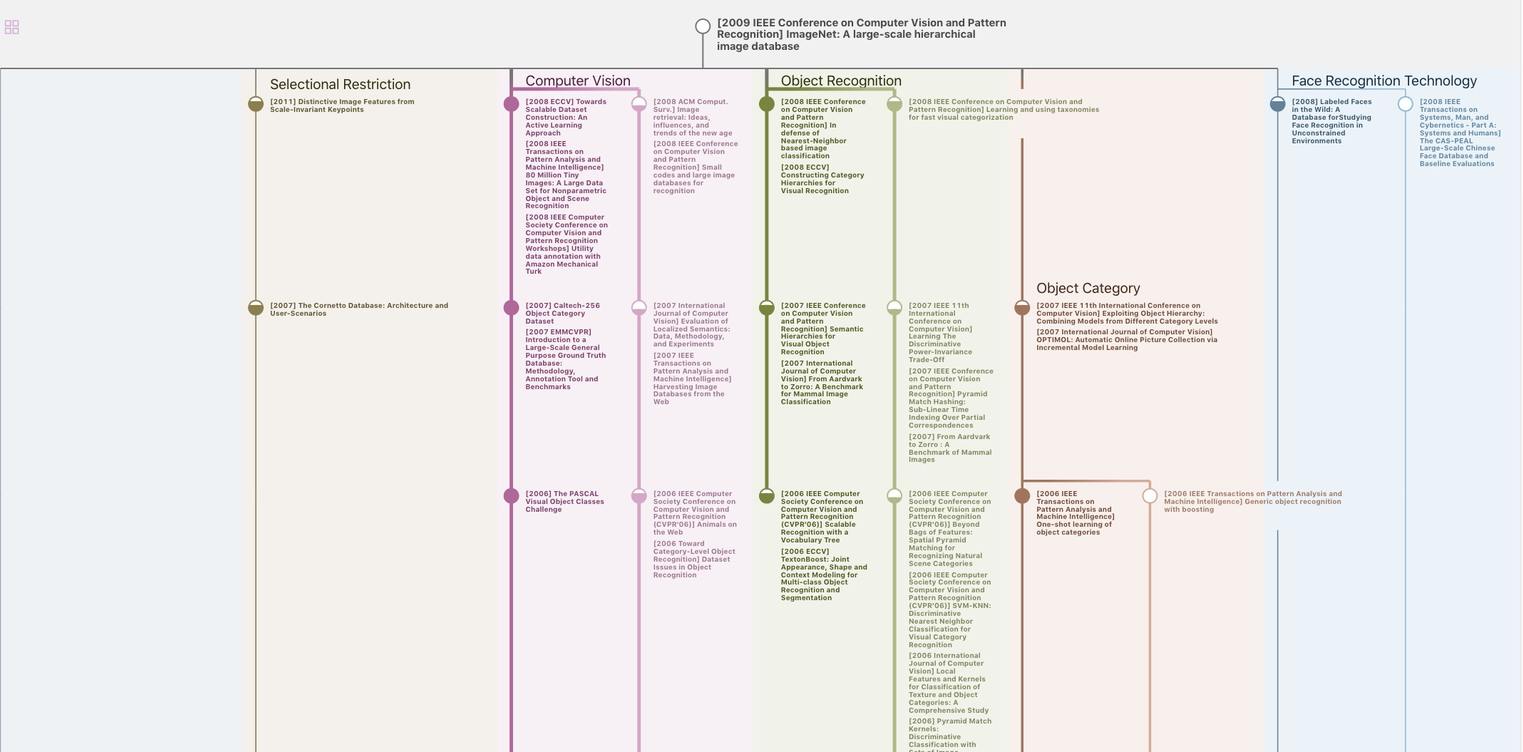
生成溯源树,研究论文发展脉络
Chat Paper
正在生成论文摘要