Clustering and Representing Movements in an Uncertain World
international conference on data mining(2009)
摘要
Knowledge discovery in Trajectory Databases (TD) is an emerging field which has recently gained great interest; on the other hand the inherent presence of uncertainty in TD during the mining process has not been taken yet into account. Current approaches group trajectories together by accounting only the degree of their similarity, ignoring at the same time the degree in which they are unrelated and more importantly the hesitancy introduced by the uncertainty. In this paper we study the effect of uncertainty on clustering TD, as well as the representation of the clusters and introduce a three-step approach to deal with this challenge. Firstly, we propose an intuitionistic point vector representation of trajectories that encompasses the underlying uncertainty and we introduce an effective distance metric to cope with uncertainty. At the second step, we devise CenTra, a novel algorithm which tackles the problem of discovering the Centroid Trajectory of a group of movements taking into advantage the local similarity between portions of trajectories. At the final step, we devise CenTR-I-FCM a variant of the Fuzzy C-Means (FCM) clustering algorithm, appropriately modified in the context of Intuitionistic Fuzzy Set (IFS) theory, which embodies CenTra as its update procedure when producing the cluster centroids at each iteration. The experimental evaluation with real world trajectory datasets demonstrates the efficiency and effectiveness of our approach.
更多查看译文
关键词
distance metric
AI 理解论文
溯源树
样例
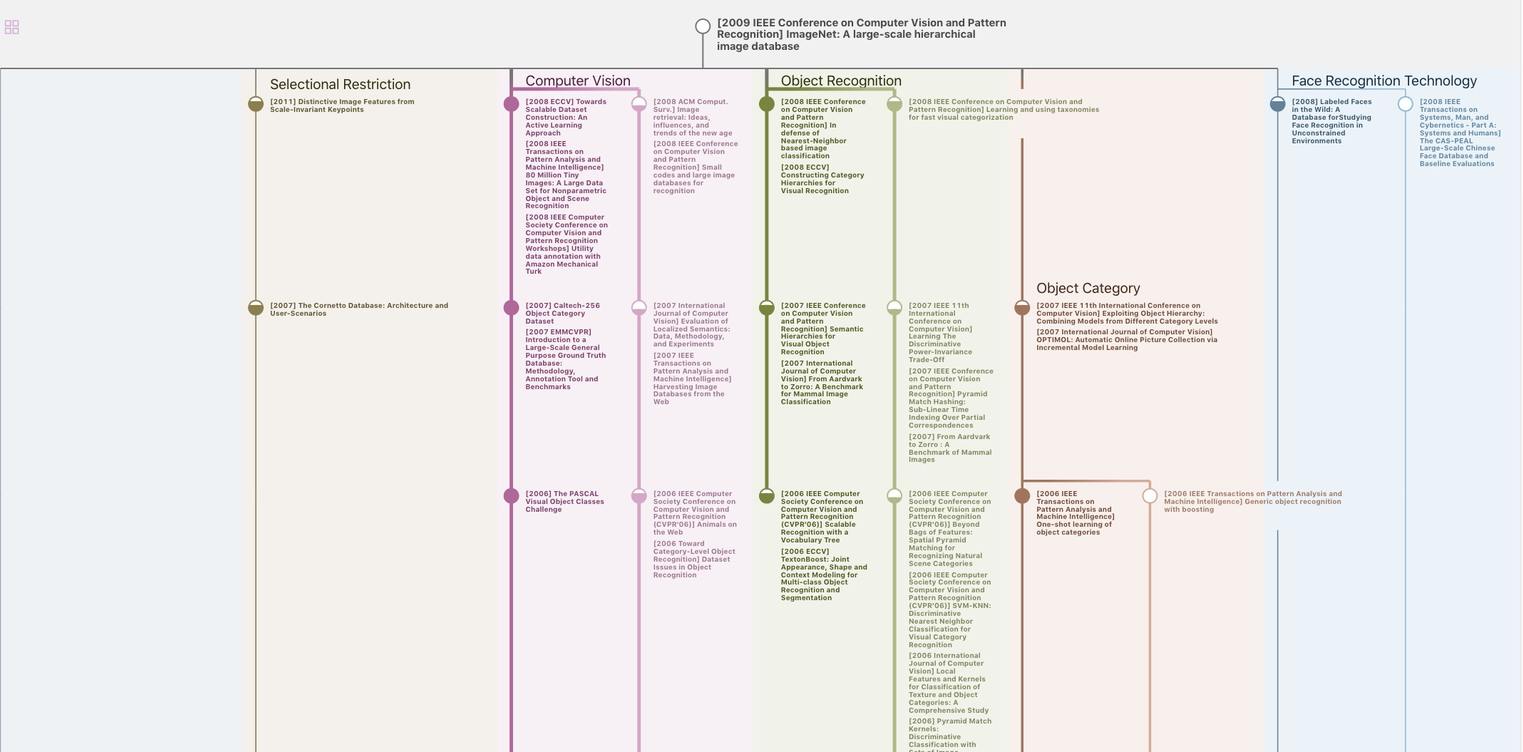
生成溯源树,研究论文发展脉络
Chat Paper
正在生成论文摘要