Towards a fully stand-alone analog/RF BIST: A cost-effective implementation of a neural classifier.
VTS(2012)
摘要
A recently proposed Built-In Self-Test (BIST) method for analog/RF circuits requires stimuli generator, measurement acquisition, and decision making circuits to be integrated on-chip along with the Device Under Test (DUT). Practical implementation of this approach hinges on the ability to meet strict area and power constraints of the circuits dedicated to test. In this work, we investigate a cost-efficient implementation of a neural classifier, which is the central component of this BIST method. We present the design of a reconfigurable analog neural network (ANN) experimentation platform and address the key questions concerning its cost-efficiency: a fully analog implementation with strict area and power budgets, a learning ability of the proposed architecture, fast dynamic programming of the weight memory during training, and high precision non-volatile storage of weight coefficients during operation or standby. Using this platform, we implement an ontogenic neural network (ONN) along with the corresponding training algorithms. Finally, we demonstrate the learning ability of the proposed architecture with a real-world case study wherein we train the ANN to predict the results of production specification testing for a large number of RF transceiver chips fabricated by Texas Instruments.
更多查看译文
关键词
built-in self test,decision circuits,dynamic programming,integrated circuit testing,neural nets,transceivers,ANN,DUT,ONN,RF transceiver chips,Texas Instruments,analog neural network,built-in self-test,cost-effective implementation,decision making circuits,device under test,dynamic programming,integrated on-chip,measurement acquisition,neural classifier,ontogenic neural network,stand-alone analog/RF BIST,stimuli generator,weight memory
AI 理解论文
溯源树
样例
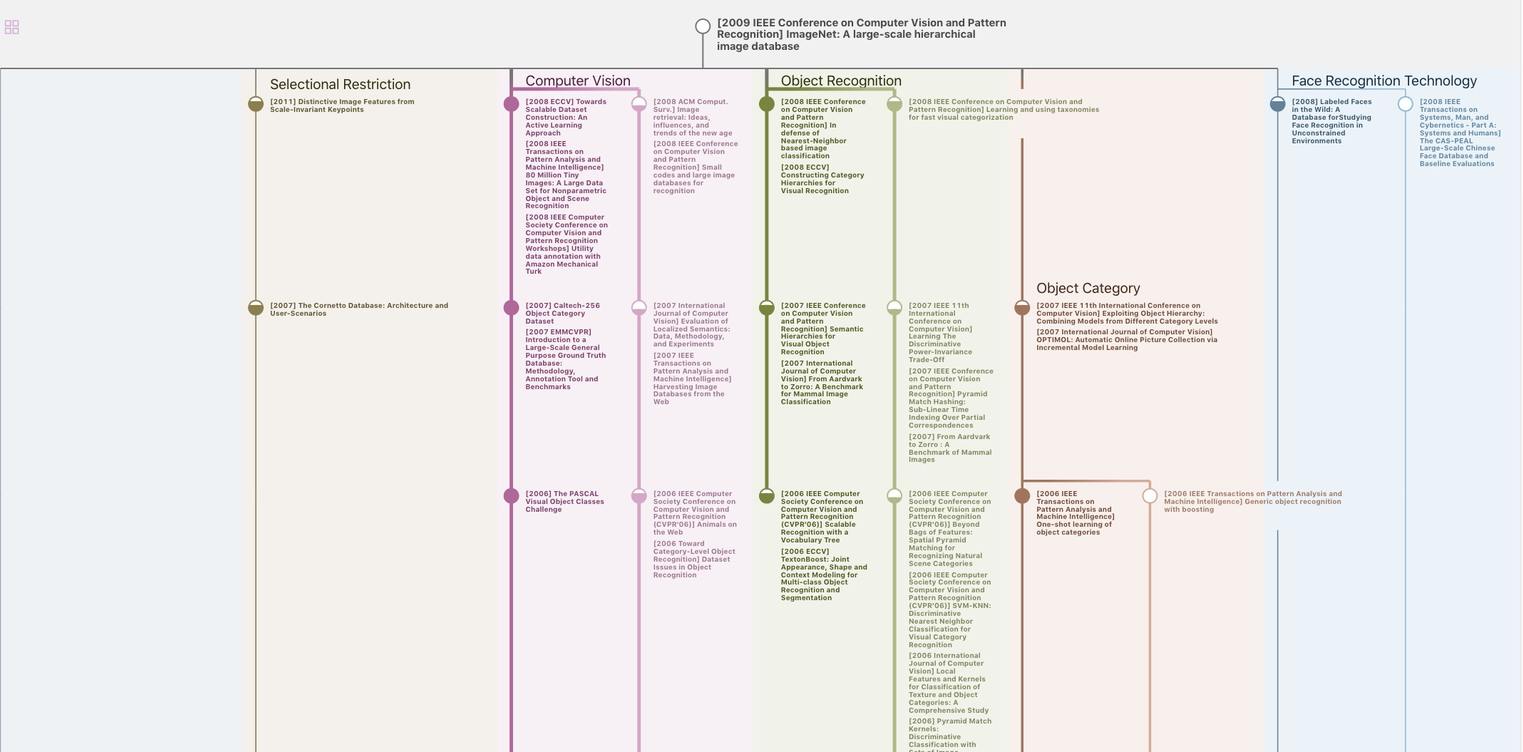
生成溯源树,研究论文发展脉络
Chat Paper
正在生成论文摘要