Rough set based cluster ensemble selection
Fusion(2013)
摘要
Ensemble clustering have been attracting lots of attentions, which combining several base data partitions to generate a single consensus partition with improved stability and robustness. Diversity is critical for the success of ensemble clustering. To enhance this characteristic, a subset of cluster ensemble is selected by removing the redundant partitions. Combined with ranking and forward selection strategies, the significance of attribute defined in rough set theory is employed as a heuristic to find the subset of cluster ensemble. Experimental results on the UCI machine learning repository demonstrate that the proposed algorithm is feasible and effective.
更多查看译文
关键词
rough set theory,cluster ensemble selection,pattern clustering,attribute significance,ensemble clustering,feature selection,rough set,set theory,glass,clustering algorithms,information entropy
AI 理解论文
溯源树
样例
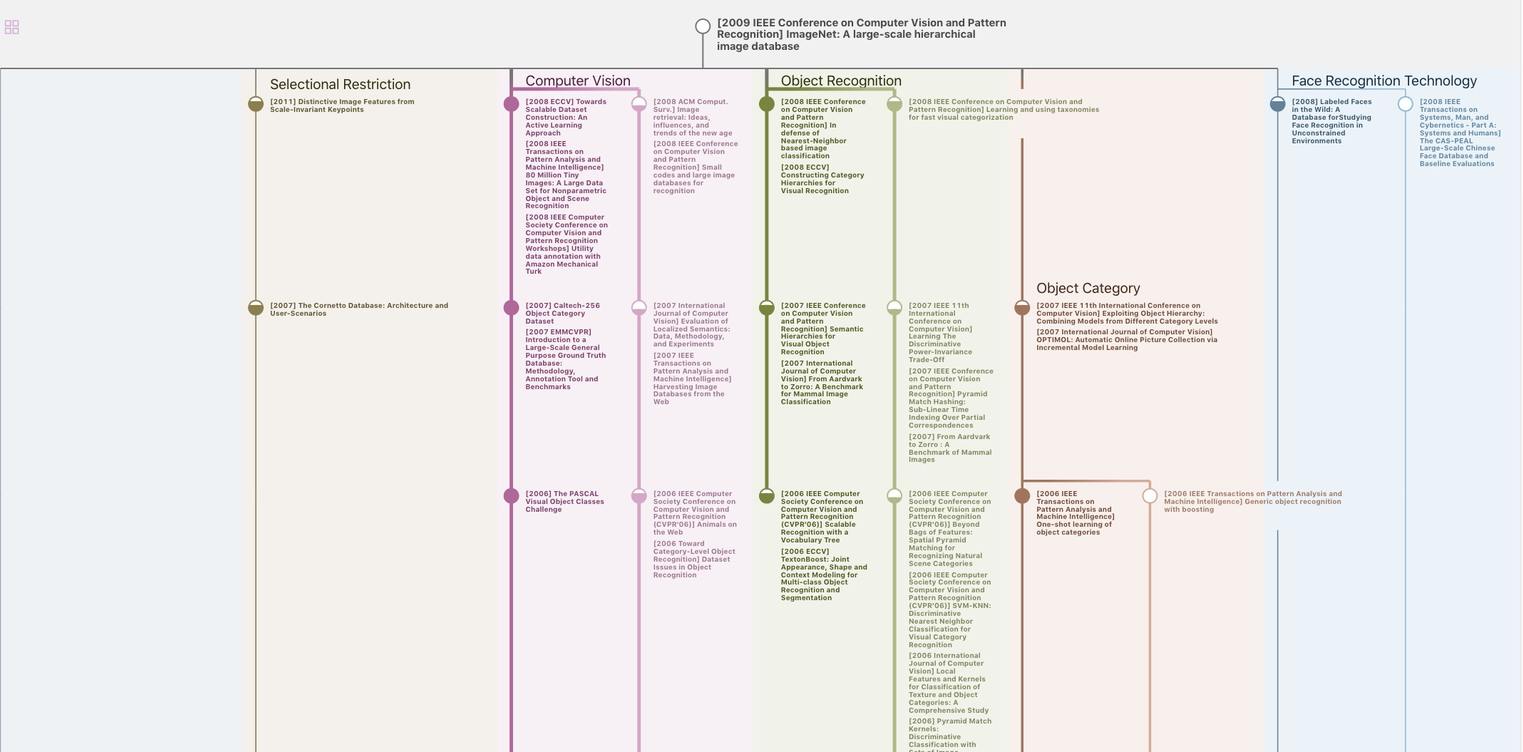
生成溯源树,研究论文发展脉络
Chat Paper
正在生成论文摘要