Hierarchical Discriminative Sparse Coding Via Bidirectional Connections
2011 INTERNATIONAL JOINT CONFERENCE ON NEURAL NETWORKS (IJCNN)(2011)
摘要
Conventional sparse coding learns optimal dictionaries of feature bases to approximate input signals; however, it is not favorable to classify the inputs. Recent research has focused on building discriminative sparse coding models to facilitate the classification tasks. In this paper, we develop a new discriminative sparse coding model via bidirectional flows. Sensory inputs (from bottom-up) and discriminative signals (supervised from top-down) are propagated through a hierarchical network to form sparse representations at each level. The l(0)-constrained sparse coding model allows highly efficient online learning and does not require iterative steps to reach a fixed point of the sparse representation. The introduction of discriminative top-down information flows helps to group reconstructive features belonging to the same class and thus to benefit the classification tasks. Experiments are conducted on multiple data sets including natural images, handwritten digits and 3-D objects with favorable results. Compared with unsupervised sparse coding via only bottom-up directions, the two-way discriminative approach improves the recognition performance significantly.
更多查看译文
关键词
top down,fixed point,information flow,bottom up,sparse coding,sparse representation,learning artificial intelligence,image classification
AI 理解论文
溯源树
样例
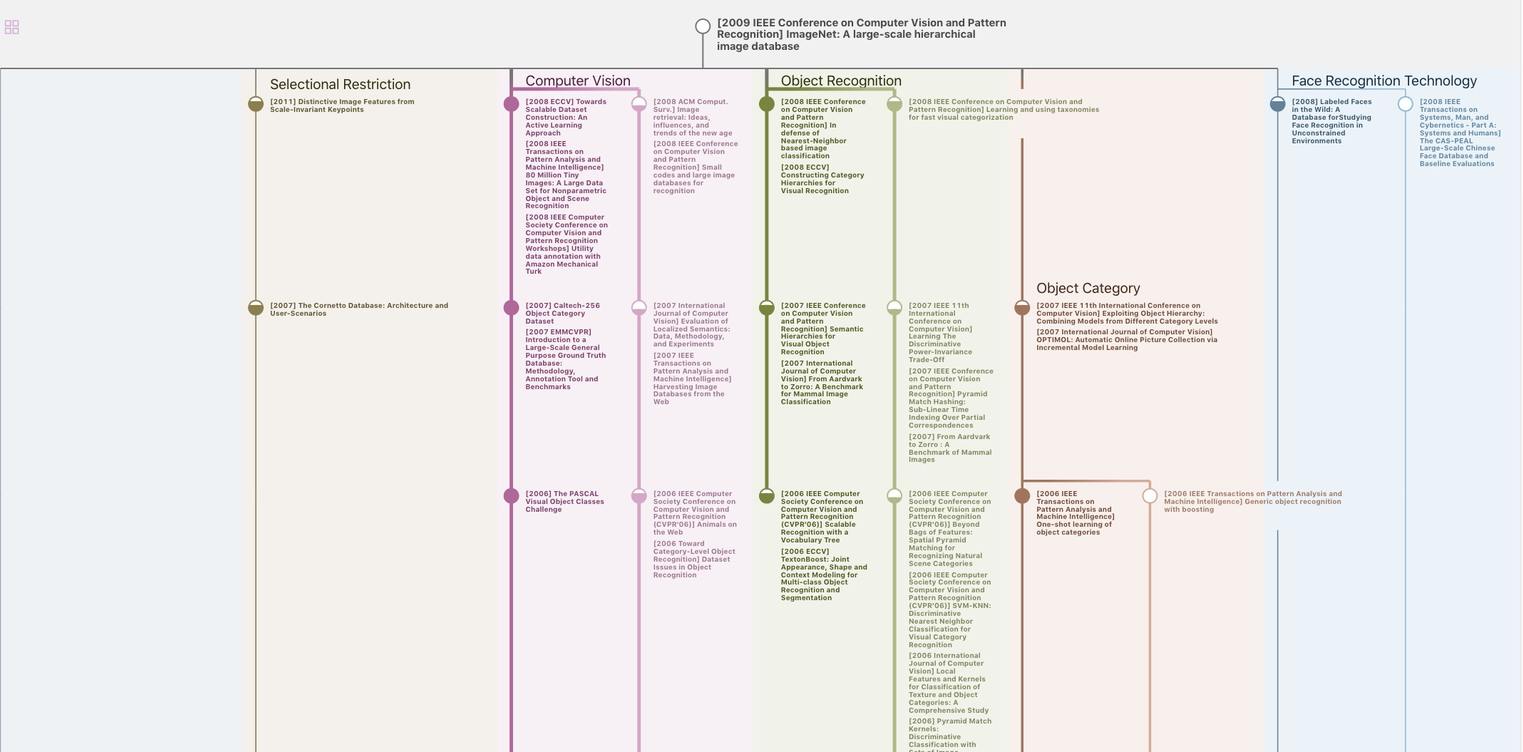
生成溯源树,研究论文发展脉络
Chat Paper
正在生成论文摘要