Ternary Sparse Coding.
LVA/ICA'12: Proceedings of the 10th international conference on Latent Variable Analysis and Signal Separation(2012)
摘要
We study a novel sparse coding model with discrete and symmetric prior distribution. Instead of using continuous latent variables distributed according to heavy tail distributions, the latent variables of our approach are discrete. In contrast to approaches using binary latents, we use latents with three states (-1, 0, and 1) following a symmetric and zero-mean distribution. While using discrete latents, the model thus maintains important properties of standard sparse coding models and of its recent variants. To efficiently train the parameters of our probabilistic generative model, we apply a truncated variational EM approach (Expectation Truncation). The resulting learning algorithm infers all model parameters including the variance of data noise and data sparsity. In numerical experiments on artificial data, we show that the algorithm efficiently recovers the generating parameters, and we find that the applied variational approach helps in avoiding local optima. Using experiments on natural image patches, we demonstrate large-scale applicability of the approach and study the obtained Gabor-like basis functions.
更多查看译文
关键词
Hide Variable, Primary Visual Cortex, Sparse Code, Data Noise, Probabilistic Generative Model
AI 理解论文
溯源树
样例
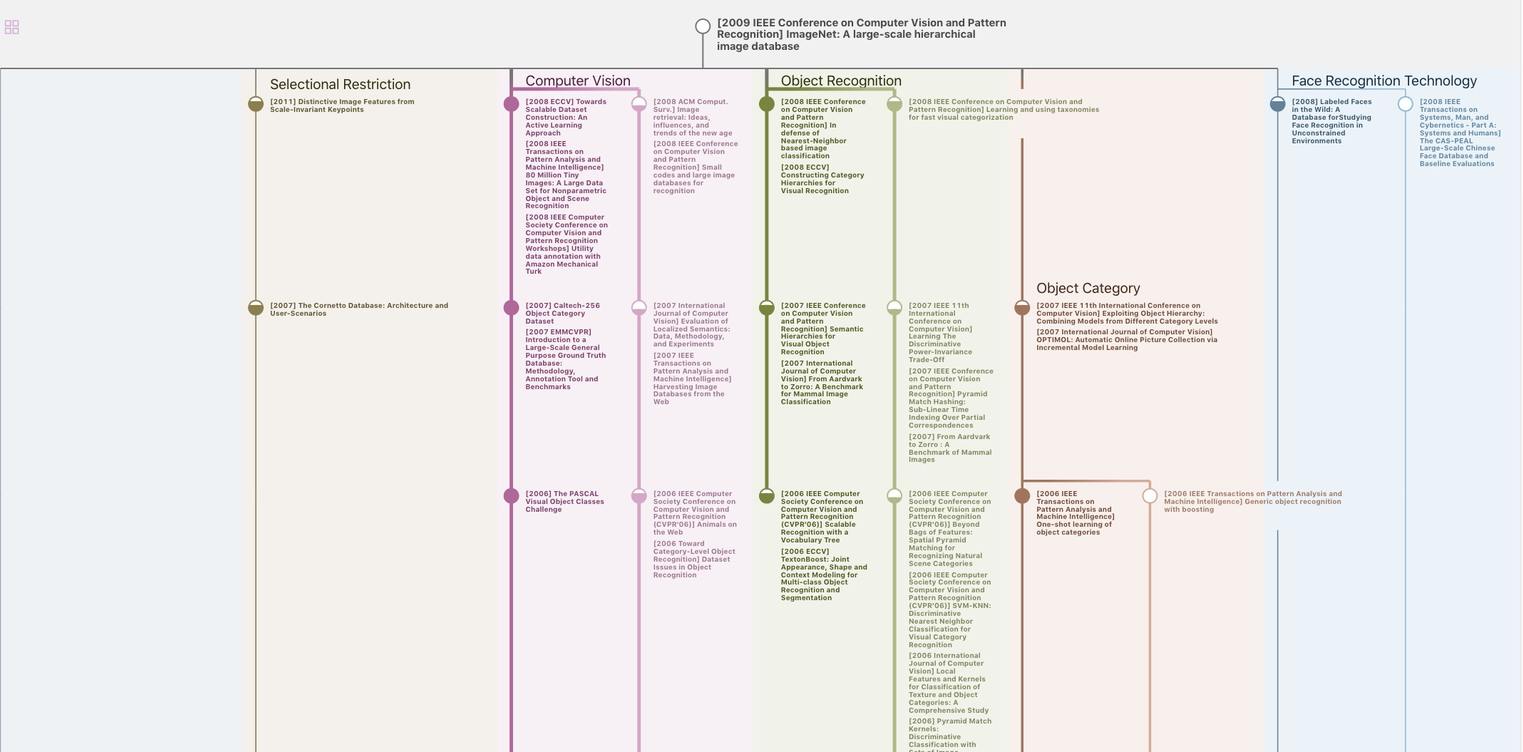
生成溯源树,研究论文发展脉络
Chat Paper
正在生成论文摘要