Unsupervised image ranking.
MM09: ACM Multimedia Conference Beijing China October, 2009(2009)
摘要
In the paper, we propose and test an unsupervised approach for image ranking. Prior solutions are based on image content and the similarity graph connecting images. We generalize this idea by directly estimating the likelihood of each photo in a feature space. We hypothesize the photos at the peaks of this distribution are the most likely photos for any given category and therefore these images are the most representative.
Our approach is unsupervised and allows for various feature modalities. We demonstrate the effectiveness of our approach using both visual-content-based and tag-based features. The experimental evaluation shows that the presented model outperforms baseline approaches. Moreover, the performance of our method will only get better with time as more images move online and it is thus possible to build more detailed models based on the approach presented here.
更多查看译文
AI 理解论文
溯源树
样例
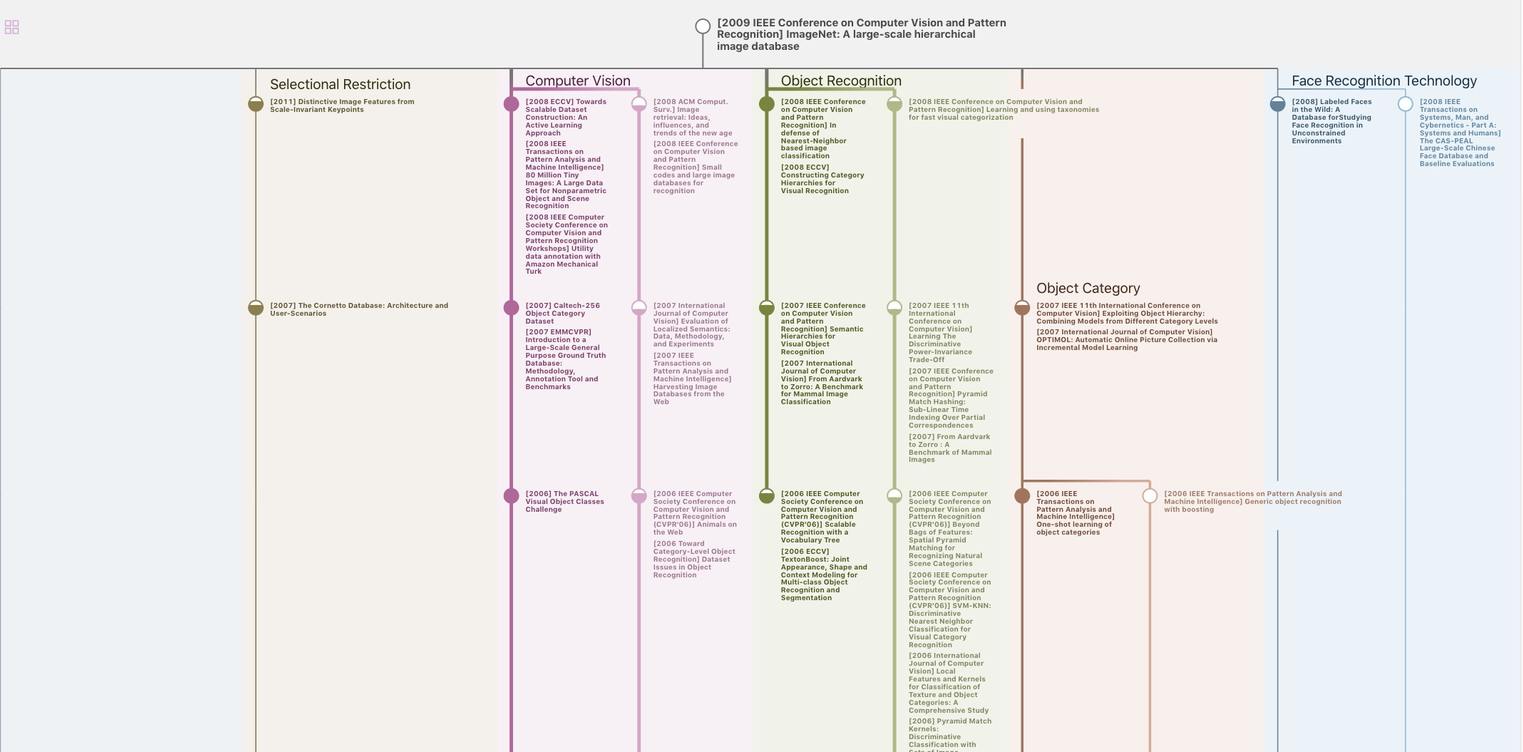
生成溯源树,研究论文发展脉络
Chat Paper
正在生成论文摘要