Parameter Sensitive Detectors
CVPR(2007)
摘要
Object detection can be challenging when the object class exhibits large variations. One commonly-used strategy is to first partition the space of possible object variations and then train separate classifiers for each portion. However, with continuous spaces the partitions tend to be arbitrary since there are no natural boundaries (for example, consider the continuous range of human body poses). In this paper, a new formulation is proposed, where the detectors themselves are associated with continuous parameters, and reside in a parameterized function space. There are two advantages of this strategy. First, a-priori partitioning of the parameter space is not needed; the detectors themselves are in a parameterized space. Second, the underlying parameters for object variations can be learned from training data in an unsupervised manner. In profile face detection experiments, at a fixed false alarm number of 90, our method attains a detection rate of 75% vs. 70% for the method of Viola-Jones. In hand shape detection, at a false positive rate of 0.1%, our method achieves a detection rate of 99.5% vs. 98% for partition based methods. In pedestrian detection, our method reduces the miss detection rate by a factor of three at a false positive rate of 1%, compared with the method of Dalal-Triggs.
更多查看译文
关键词
face recognition,pattern classification,parameter sensitive detectors,image sensors,a priori partitioning,object detection,classifiers,profile face detection,parameter estimation,technical report,function space,human body,shape,detectors,false positive rate,face detection,parameter space
AI 理解论文
溯源树
样例
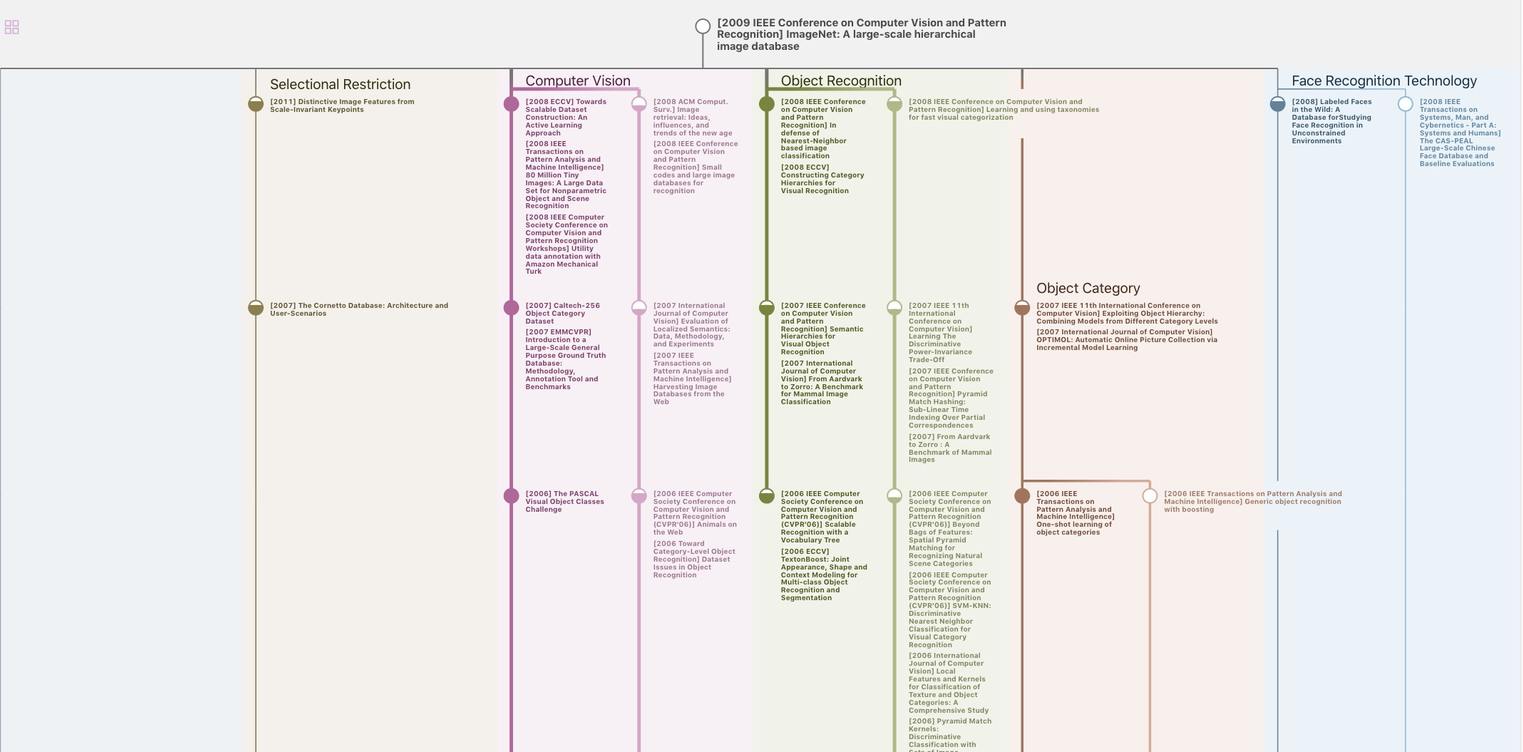
生成溯源树,研究论文发展脉络
Chat Paper
正在生成论文摘要