Transforming Auto-Encoders.
ICANN'11: Proceedings of the 21th international conference on Artificial neural networks - Volume Part I(2011)
摘要
The artificial neural networks that are used to recognize shapes typically use one or more layers of learned feature detectors that produce scalar outputs. By contrast, the computer vision community uses complicated, hand-engineered features, like SIFT [6], that produce a whole vector of outputs including an explicit representation of the pose of the feature. We show how neural networks can be used to learn features that output a whole vector of instantiation parameters and we argue that this is a much more promising way of dealing with variations in position, orientation, scale and lighting than the methods currently employed in the neural networks community. It is also more promising than the hand-engineered features currently used in computer vision because it provides an efficient way of adapting the features to the domain.
更多查看译文
关键词
Invariance, auto-encoder, shape representation
AI 理解论文
溯源树
样例
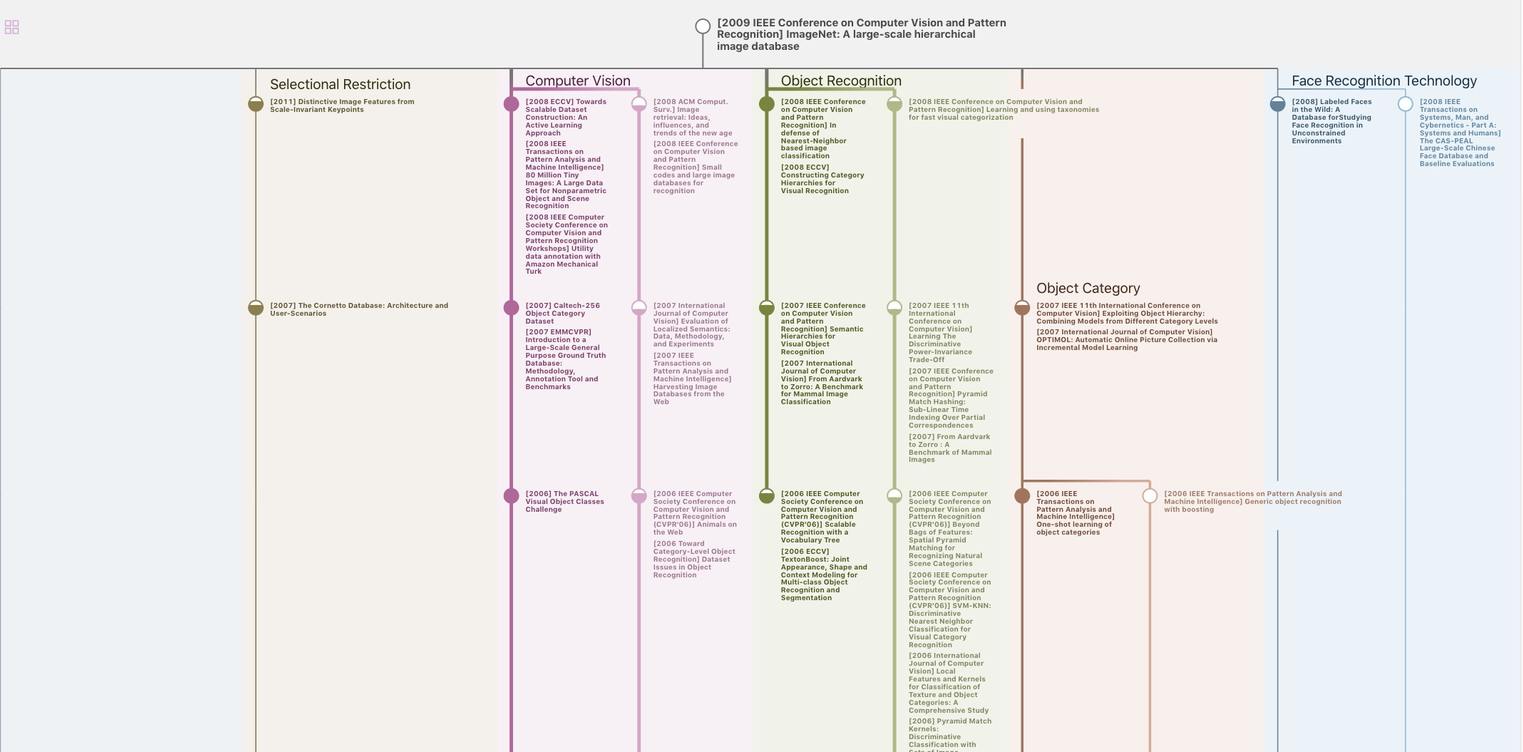
生成溯源树,研究论文发展脉络
Chat Paper
正在生成论文摘要