The Utility of Knowledge Transfer with Noisy Training Sets
msra(2013)
摘要
Knowledge transfer has traditionally concerned itself with the transfer of relevant features. Yet, in this paper, we will highlight the importance of transferring knowl- edge of which features are irrelevant. When attempting to acquire a new concept from sen- sory data, a learner is exposed to significant volumes of extraneous data. In order to use knowledge transfer for quickly acquiring new concepts, within a given class (e.g. learning a new character from the set of characters, a new face from the set of faces, a new vehicle from the set of vehicles etc.), a learner must know which features are ignorable or repeatedly be forced to relearn them. We have previously demonstrated knowledge transfer in deep convolutional neural nets (DCNN's) (Gutstein, Fuentes, & Freudenthal 2007). In this paper, we give experimental results that demonstrate the increased im- portance of knowledge transfer when learning new con- cepts from noisy data. Additionally, we exploit the layered nature of deep con- volutional neural nets (DCNN's) to discover more effi- cient and targeted methods of transfer. We observe that most of the transfer occurs within the 3.2% of weights that are closest to the input image.
更多查看译文
关键词
neural net
AI 理解论文
溯源树
样例
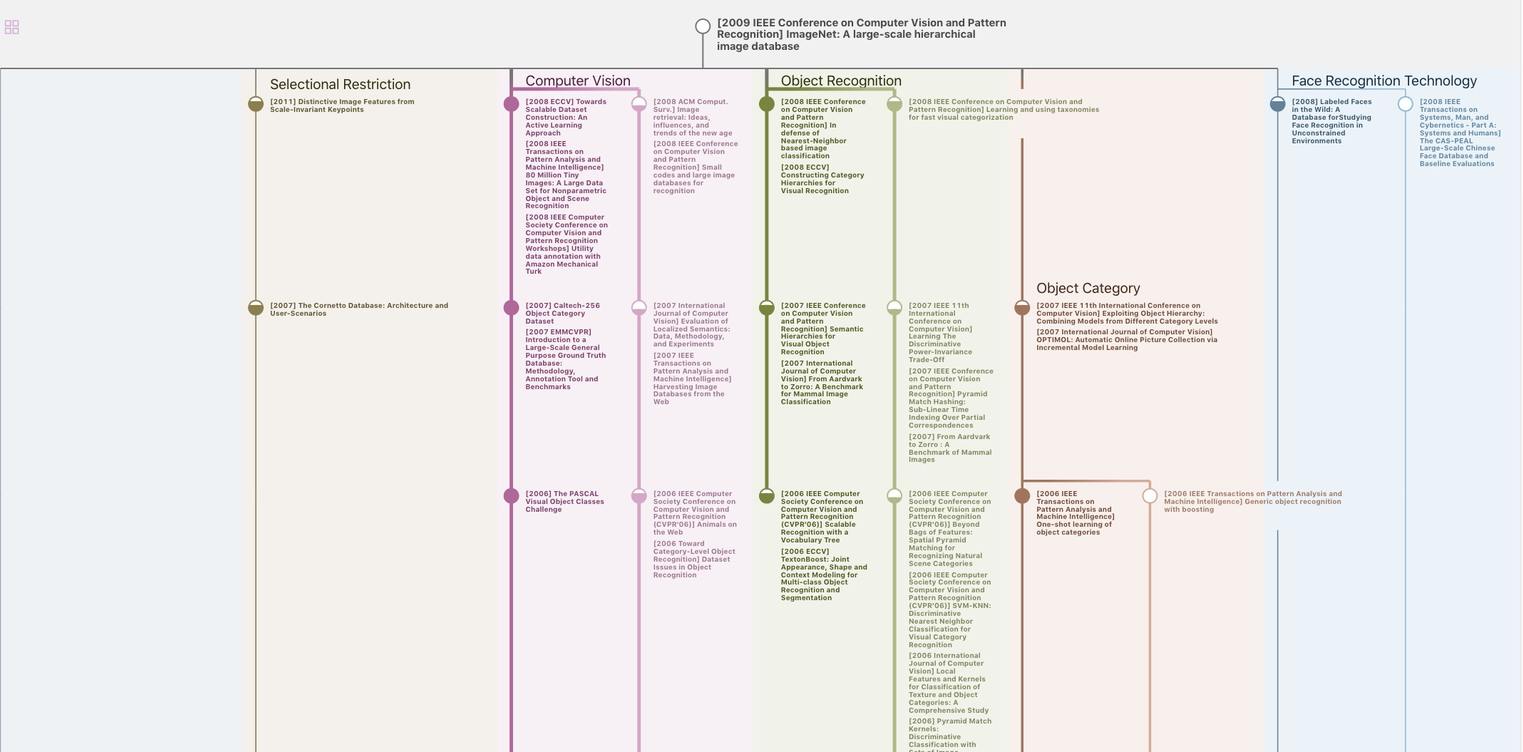
生成溯源树,研究论文发展脉络
Chat Paper
正在生成论文摘要