Adaptive power management using reinforcement learning.
ICCAD(2009)
摘要
ABSTRACTSystem level power management must consider the uncertainty and variability that comes from the environment, the application and the hardware. A robust power management technique must be able to learn the optimal decision from past history and improve itself as the environment changes. This paper presents a novel online power management technique based on model-free constrained reinforcement learning (RL). It learns the best power management policy that gives the minimum power consumption for a given performance constraint without any prior information of workload. Compared with existing machine learning based power management techniques, the RL based learning is capable of exploring the trade-off in the power-performance design space and converging to a better power management policy. Experimental results show that the proposed RL based power management achieves 24% and 3% reduction in power and latency respectively comparing to the existing expert based power management.
更多查看译文
关键词
learning (artificial intelligence),power consumption,power engineering computing,power system management,adaptive power management,expert based power management,machine learning,model-free constrained reinforcement learning,online power management technique,performance constraint,power consumption,power management policy,power management techniques,power-performance design space,system level power management,Power management,Q-learning,model-free,reinforcement learning,
AI 理解论文
溯源树
样例
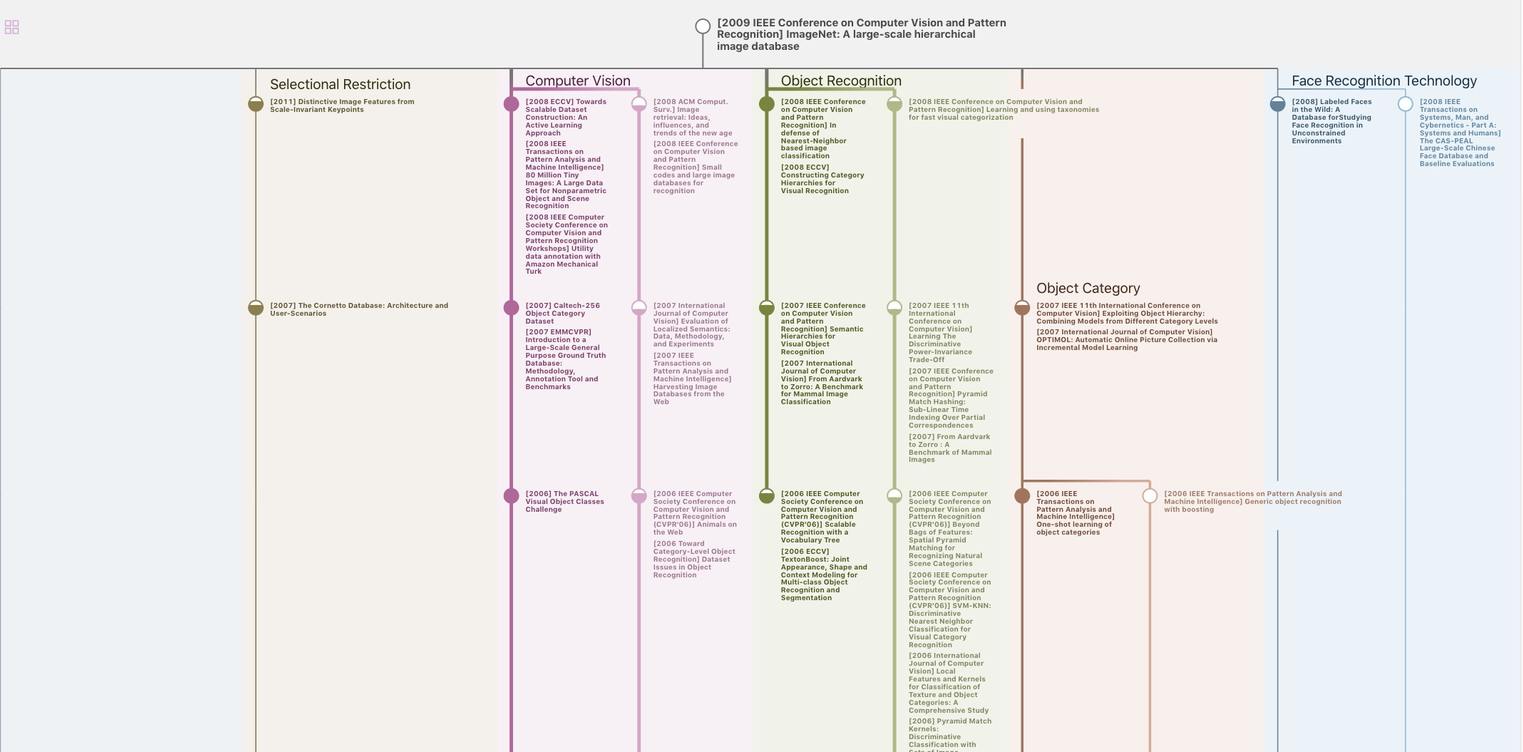
生成溯源树,研究论文发展脉络
Chat Paper
正在生成论文摘要