3UHGLFWLYH6RIWZDUH
msra
摘要
$EVWUDFW We examine the adaptation of classical machine learning selection criteria to ensure or improve the predictiveness of specifications. Moreover, inspired in incremental learning, software construction is also seen as an incremental process which must generate and revise the specification with the main goal of being predictive to requirements evolution. The new goal is not necessarily to achieve the highest accuracy at the end of a first prototype or version, but to maximise the cumulative benefits obtained throughout the entire software life-cycle. This suggests a new software life-cycle, whose main characteristic is to move modifications earlier, by using more eager inductive techniques, and reducing overall modification probability. This new predictive software life-cycle is particularised for the case of (functional) logic programming, placing the deductive/inductive techniques necessary for each stage of the life-cycle. The maturity of each stage and the practical possibilities for a (semi-)automation of the cycle based on declarative techniques are also discussed.
更多查看译文
关键词
predictive modelling,software life-cycles,data-mining,hypotheses selection criteria.,.h\zrugv software development,inductive logic programming ilp,machine learning ml
AI 理解论文
溯源树
样例
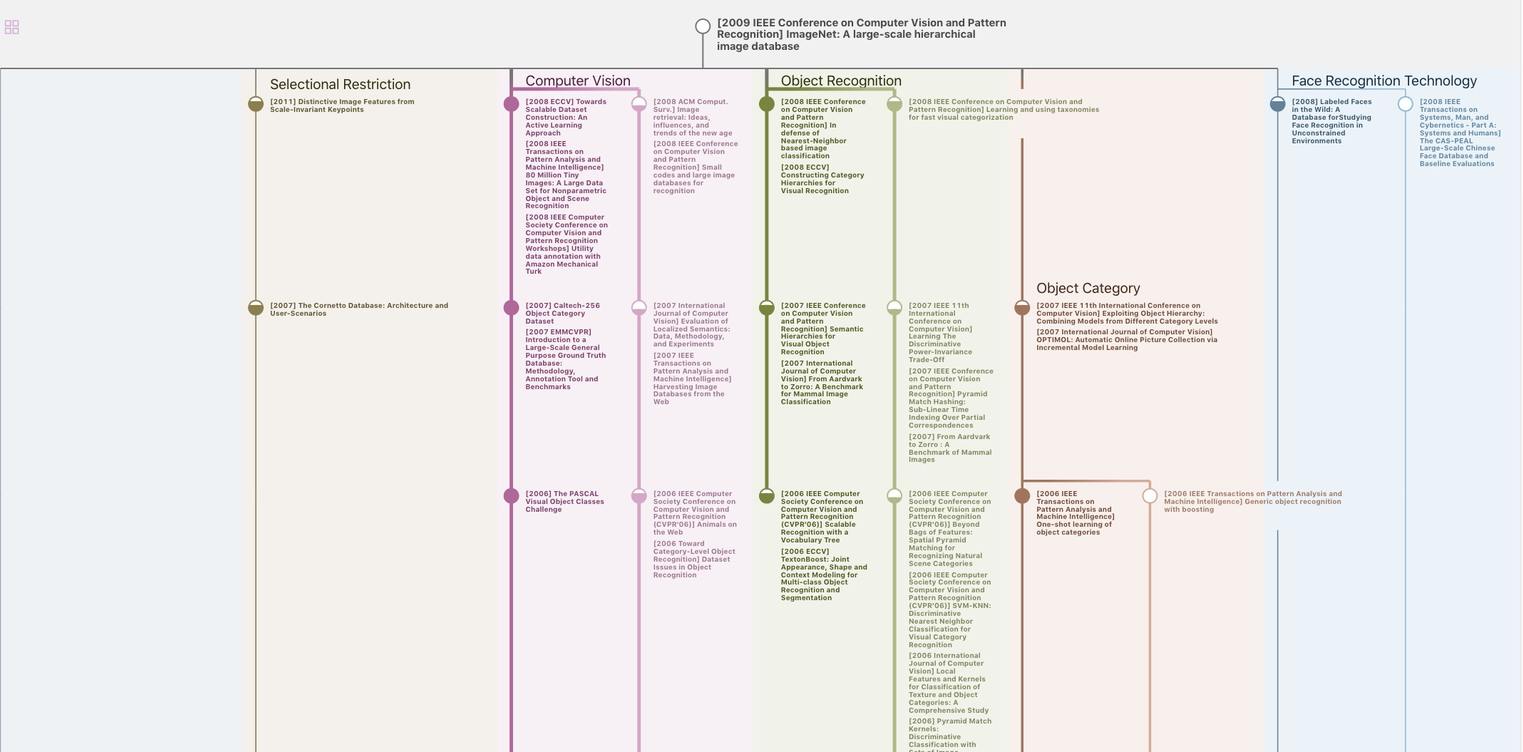
生成溯源树,研究论文发展脉络
Chat Paper
正在生成论文摘要