A comparative study of two approaches for supporting optimal network location queries
GeoInformatica(2013)
摘要
Given a set S of sites and a set O of weighted objects, an optimal location query finds the location(s) where introducing a new site maximizes the total weight of the objects that are closer to the new site than to any other site. With such a query, for instance, a franchise corporation (e.g., McDonald’s) can find a location to open a new store such that the number of potential store customers (i.e., people living close to the store) is maximized. Optimal location queries are computationally complex to compute and require efficient solutions that scale with large datasets. Previously, two specific approaches have been proposed for efficient computation of optimal location queries. However, they both assume p-norm distance (namely, L 1 and L 2 /Euclidean); hence, they are not applicable where sites and objects are located on spatial networks. In this article, we focus on optimal network location ( ONL ) queries, i.e., optimal location queries in which objects and sites reside on a spatial network. We introduce two complementary approaches, namely EONL (short for Expansion-based ONL ) and BONL (short for Bound-based ONL ), which enable efficient computation of ONL queries with datasets of uniform and skewed distributions, respectively. Moreover, with an extensive experimental study we verify and compare the efficiency of our proposed approaches with real world datasets, and we demonstrate the importance of considering network distance (rather than p-norm distance) with ONL queries.
更多查看译文
关键词
Optimal location queries,Spatial network databases
AI 理解论文
溯源树
样例
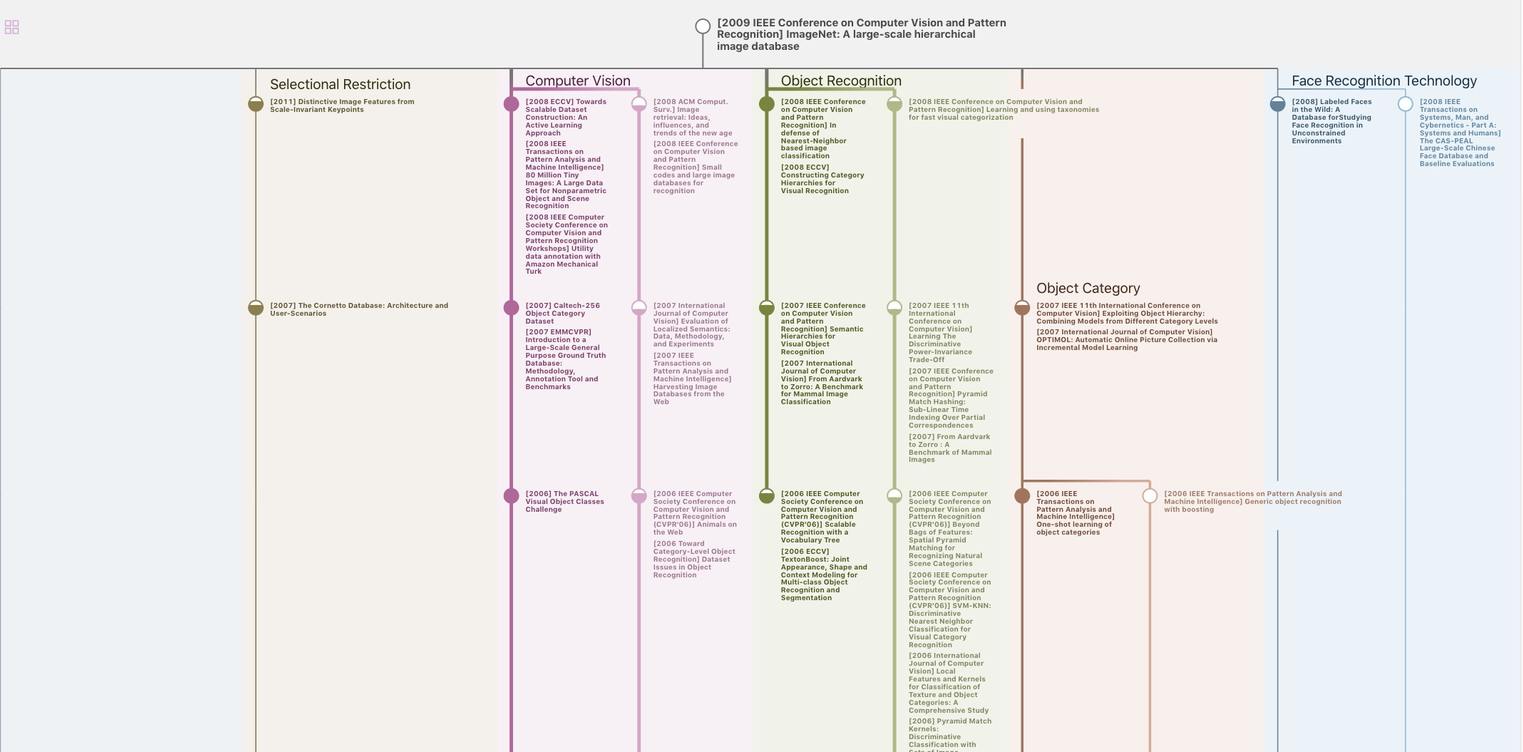
生成溯源树,研究论文发展脉络
Chat Paper
正在生成论文摘要