Elementary: Large-Scale Knowledge-Base Construction via Machine Learning and Statistical Inference
INTERNATIONAL JOURNAL ON SEMANTIC WEB AND INFORMATION SYSTEMS(2012)
摘要
Researchers have approached knowledge-base construction KBC with a wide range of data resources and techniques. The authors present Elementary, a prototype KBC system that is able to combine diverse resources and different KBC techniques via machine learning and statistical inference to construct knowledge bases. Using Elementary, they have implemented a solution to the TAC-KBP challenge with quality comparable to the state of the art, as well as an end-to-end online demonstration that automatically and continuously enriches Wikipedia with structured data by reading millions of webpages on a daily basis. The authors describe several challenges and their solutions in designing, implementing, and deploying Elementary. In particular, the authors first describe the conceptual framework and architecture of Elementary to integrate different data resources and KBC techniques in a principled manner. They then discuss how they address scalability challenges to enable Web-scale deployment. The authors empirically show that this decomposition-based inference approach achieves higher performance than prior inference approaches. To validate the effectiveness of Elementary's approach to KBC, they experimentally show that its ability to incorporate diverse signals has positive impacts on KBC quality.
更多查看译文
关键词
large-scale knowledge-base construction,knowledge-base construction kbc,kbc technique,present elementary,statistical inference,machine learning,deploying elementary,different kbc technique,kbc quality,data resource,prototype kbc system,decomposition-based inference approach,authors empirically show,information extraction,systems
AI 理解论文
溯源树
样例
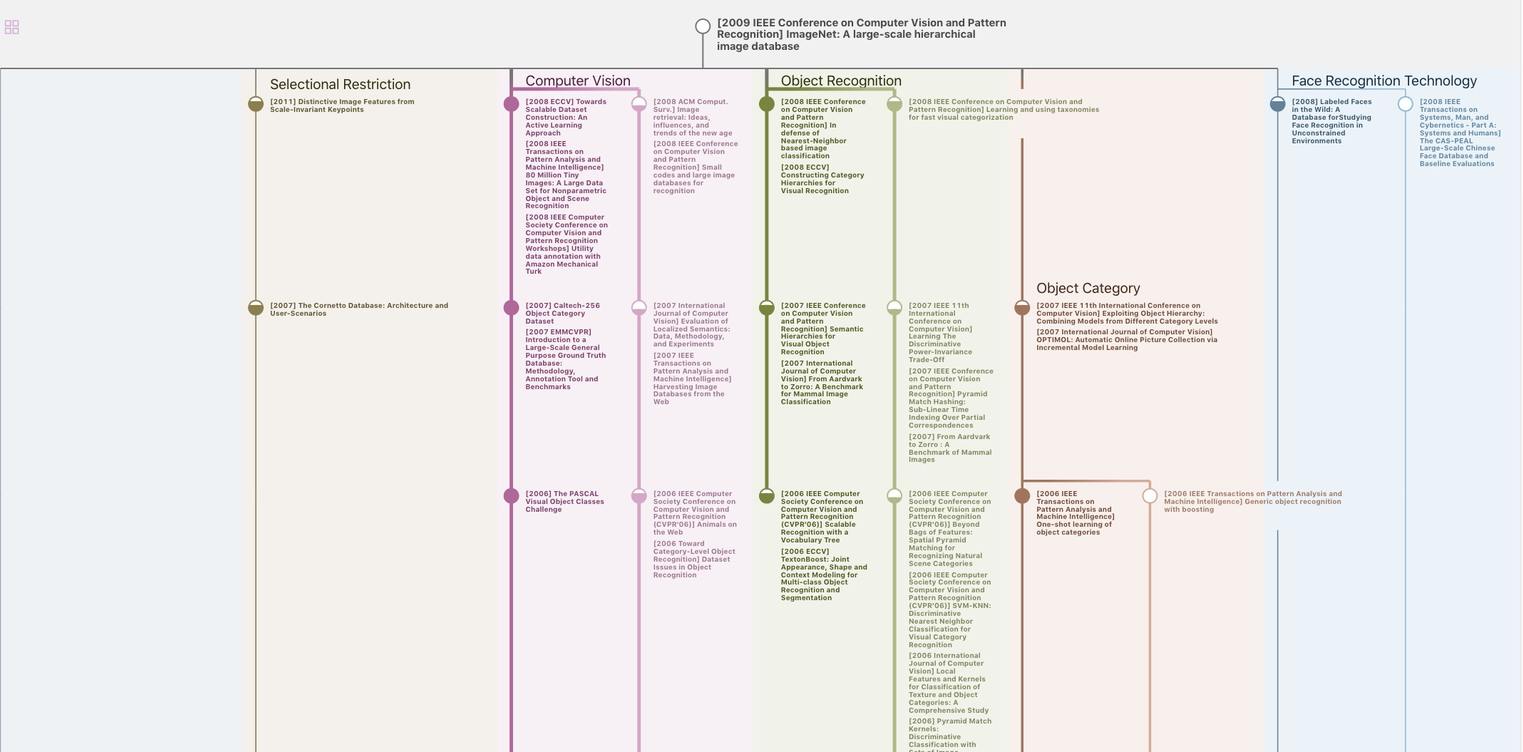
生成溯源树,研究论文发展脉络
Chat Paper
正在生成论文摘要