Adaptive Feature Selection via Boosting-Like Sparsity Regularization
2013 2nd IAPR Asian Conference on Pattern Recognition(2013)
摘要
In order to efficiently select a discriminative and complementary subset from a large feature pool, we propose a two-stage learning strategy considering both samples and their features simultaneously, namely sample selection and feature selection. The objective functions of both stages are consistent with a large margin loss. At the first stage, the support samples are selected by Support Vector Machine (SVM). At the second stage, a Boosting-like Sparsity Regularization (SRBoost) algorithm is presented to select a small number of complementary features. In detail, a weak learner is composed of a few features, which are selected by a sparsity enforcing mode, and an intermediate variable is gracefully used to reweight the corresponding sample. Extensive experimental results on the CASIA-IrisV4.0 database demonstrate that our method outperforms the state-of-the-art methods.
更多查看译文
关键词
feature selection,support vector machine,complementary subset,large margin loss,boosting-like sparsity regularization,support sample,sample selection,corresponding sample,adaptive feature selection,large feature pool,complementary feature,set theory,support vector machines,learning artificial intelligence
AI 理解论文
溯源树
样例
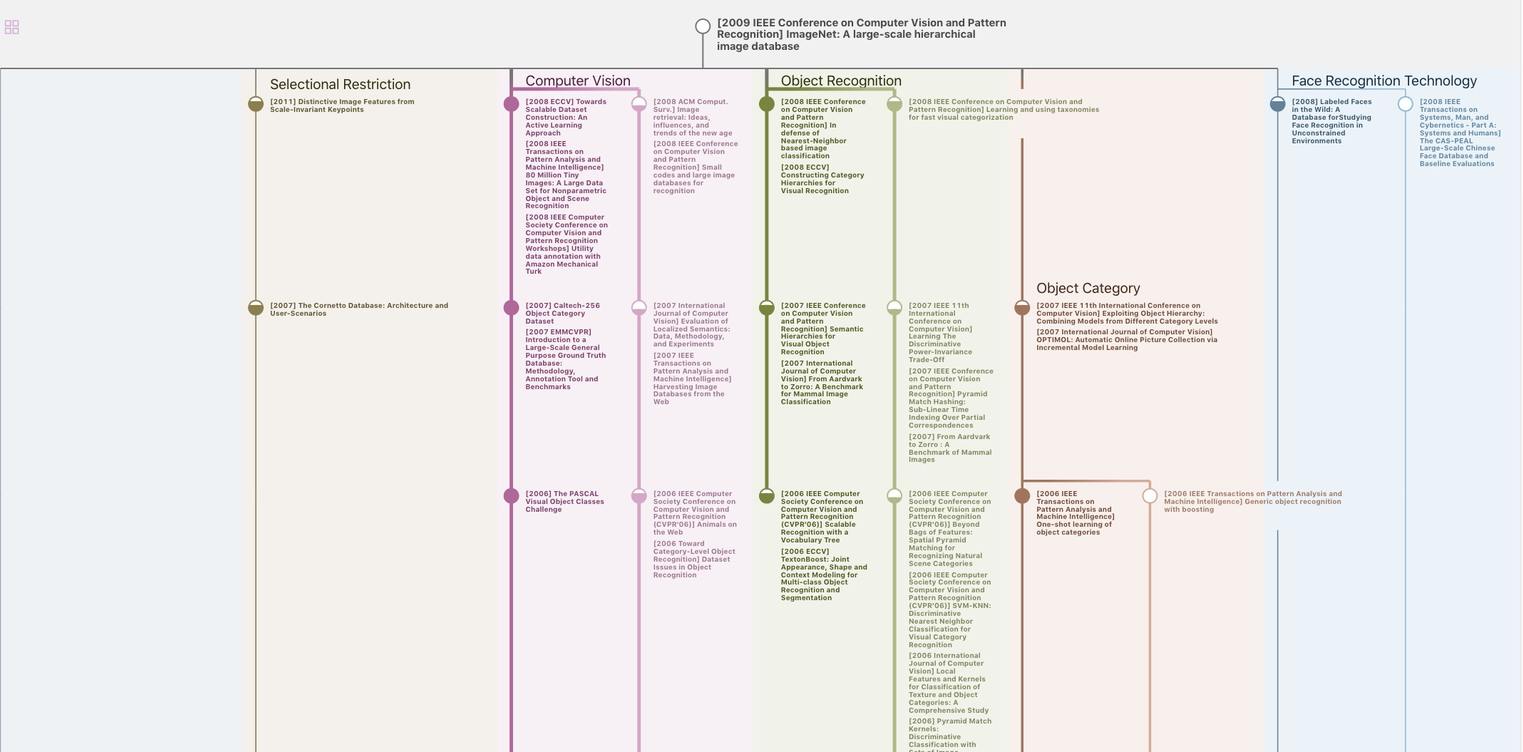
生成溯源树,研究论文发展脉络
Chat Paper
正在生成论文摘要