Decomposition and Approximation of Loopy Bayesian Networks
FUNDAMENTA INFORMATICAE(2013)
摘要
This paper proposes a new method, conditional probability table CPT decomposition, to analyze the independent and deterministic components of CPT. This method can be used to approximate and analyze Baysian networks. The decomposition of Bayesian networks is accomplished by representing CPTs as a linear combination of extreme CPTs, which forms a new framework to conduct inference. Based on this new framework, inference in Bayesian networks can be done by decomposing them into less connected and weighted subnetworks. We can achieve exact inference if the original network is decomposed into singly-connected subnetworks. Besides, approximate inference can be done by discarding the subnetworks with small weights or by a partial decomposition and application of belief propagation BP on the still multiply-connected subnetworks. Experiments show that the decomposition-based approximation outperforms BP in most cases.
更多查看译文
关键词
singly-connected subnetworks,exact inference,bayesian network,belief propagation bp,multiply-connected subnetworks,approximate inference,new framework,partial decomposition,new method,weighted subnetworks,loopy bayesian networks,conditional probability table,decomposition
AI 理解论文
溯源树
样例
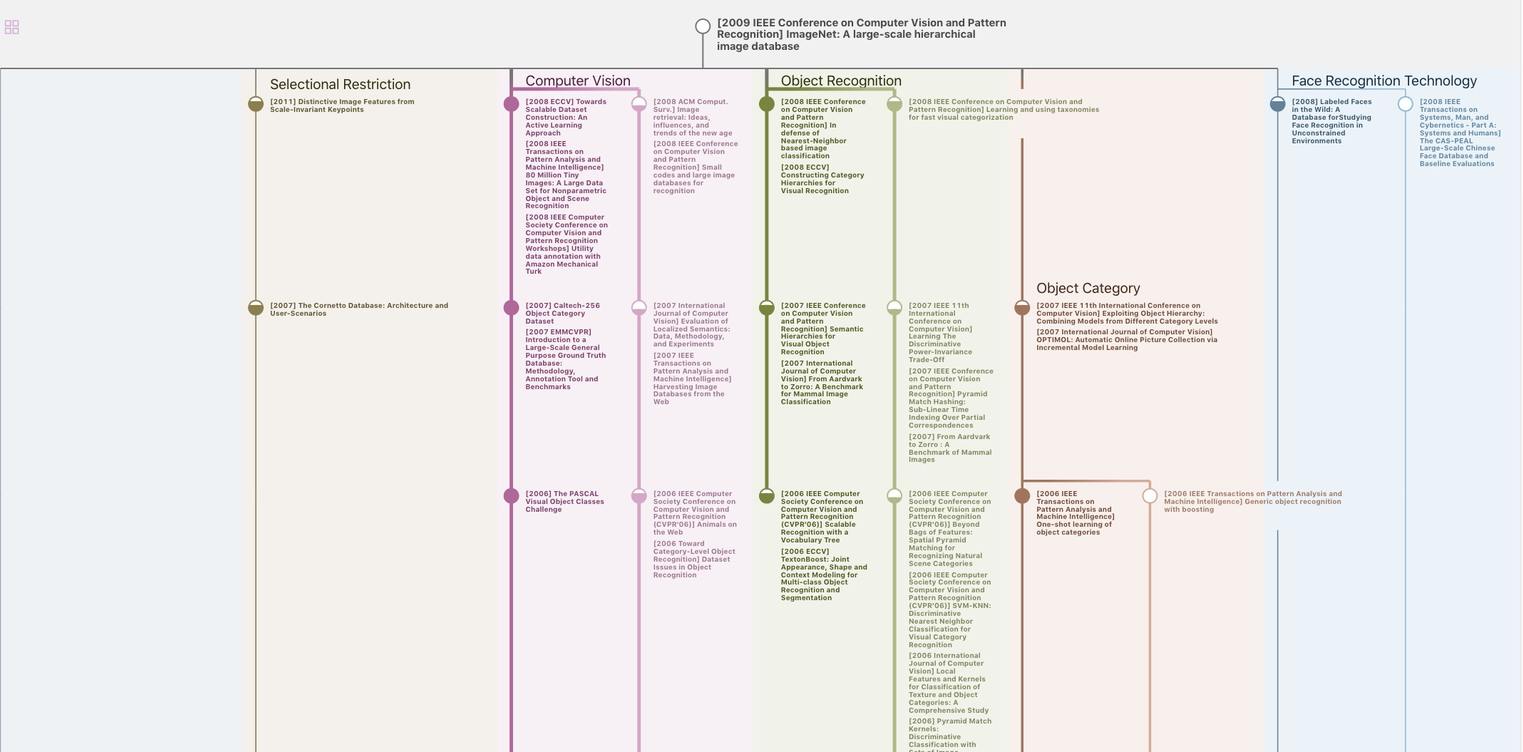
生成溯源树,研究论文发展脉络
Chat Paper
正在生成论文摘要