Probabilistic models in IR and their relationships
Information Retrieval(2014)
摘要
A solid research path towards new information retrieval models is to further develop the theory behind existing models. A profound understanding of these models is therefore essential. In this paper, we revisit probability ranking principle (PRP)-based models, probability of relevance (PR) models, and language models, finding conceptual differences in their definition and interrelationships. The probabilistic model of the PRP has not been explicitly defined previously, but doing so leads to the formulation of two actual principles with different objectives. First, the belief probability ranking principle (BPRP), which considers uncertain relevance between known documents and the current query, and second, the popularity probability ranking principle (PPRP), which considers the probability of relevance of documents among multiple queries with the same features. Our analysis shows how some of the discussed PR models implement the BPRP or the PPRP while others do not. However, for some models the parameter estimation is challenging. Finally, language models are often presented as related to PR models. However, we find that language models differ from PR models in every aspect of a probabilistic model and the effectiveness of language models cannot be explained by the PRP.
更多查看译文
关键词
Probabilistic models,Probability of relevance,Probability ranking principle,Language models
AI 理解论文
溯源树
样例
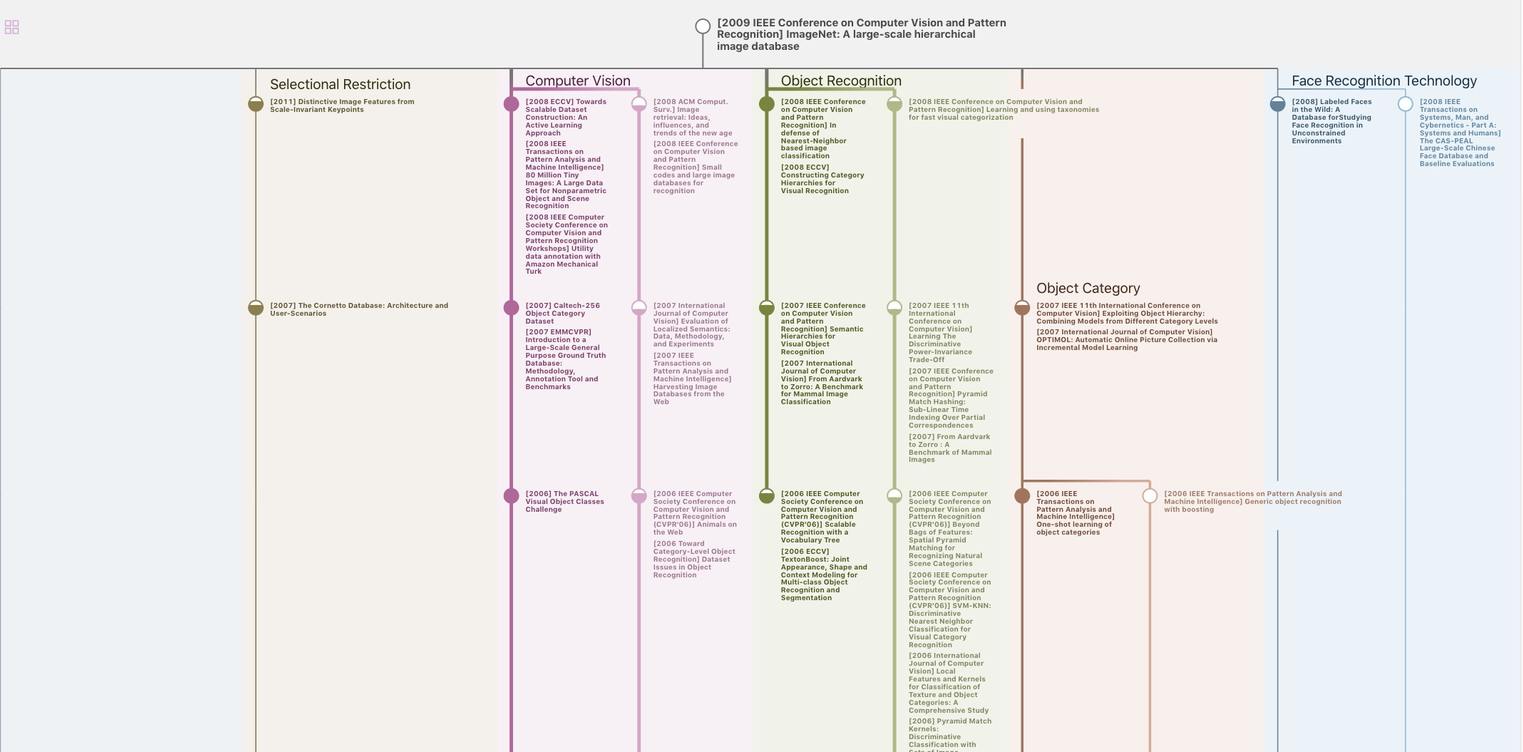
生成溯源树,研究论文发展脉络
Chat Paper
正在生成论文摘要