Traffic sign recognition using group sparse coding
Information Sciences(2014)
摘要
Recognizing traffic signs is a challenging problem; and it has captured the attention of the computer vision community for several decades. Essentially, traffic sign recognition is a multi-class classification problem that has become a real challenge for computer vision and machine learning techniques. Although many machine learning approaches are used for traffic sign recognition, they are primarily used for classification, not feature design. Identifying rich features using modern machine learning methods has recently attracted attention and has achieved success in many benchmarks. However these approaches have not been fully implemented in the traffic sign recognition problem. In this paper, we propose a new approach to tackle the traffic sign recognition problem. First, we introduce a new feature learning approach using group sparse coding. The primary goal is to exploit the intrinsic structure of the pre-learned visual codebook. This new coding strategy preserves locality and encourages similar descriptors to share similar sparse representation patterns. Second, we use a non-uniform quantization approach based on log-polar mapping. Using the log-polar mapping of the traffic sign image, rotated and scaled patterns are converted into shifted patterns in the new space. We extract the local descriptors from these patterns to learn the features. Finally, by evaluating the proposed approach using the German Traffic Sign Recognition Benchmark dataset, we show that the proposed coding strategy outperforms existing coding methods and the obtained results are comparable to the state-of-the-art.
更多查看译文
关键词
multi-class classification problem,traffic sign recognition,traffic sign recognition problem,group sparse coding,log-polar mapping,coding method,challenging problem,new approach,recognizing traffic sign,traffic sign image,sparse coding
AI 理解论文
溯源树
样例
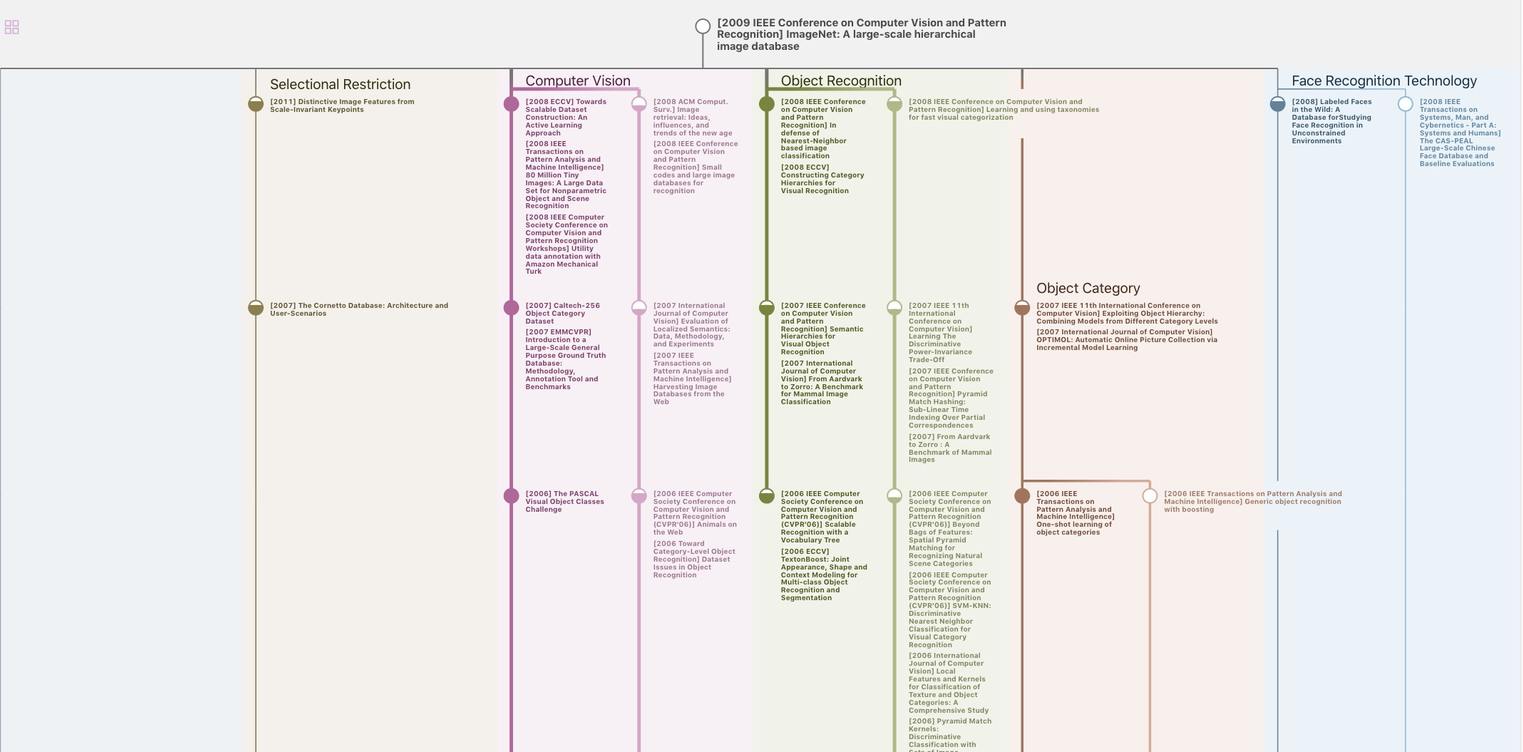
生成溯源树,研究论文发展脉络
Chat Paper
正在生成论文摘要