Total Variation Regularization for Functions with Values in a Manifold
Computer Vision(2013)
摘要
While total variation is among the most popular regularizers for variational problems, its extension to functions with values in a manifold is an open problem. In this paper, we propose the first algorithm to solve such problems which applies to arbitrary Riemannian manifolds. The key idea is to reformulate the variational problem as a multilabel optimization problem with an infinite number of labels. This leads to a hard optimization problem which can be approximately solved using convex relaxation techniques. The framework can be easily adapted to different manifolds including spheres and three-dimensional rotations, and allows to obtain accurate solutions even with a relatively coarse discretization. With numerous examples we demonstrate that the proposed framework can be applied to variational models that incorporate chromaticity values, normal fields, or camera trajectories.
更多查看译文
关键词
cameras,convex programming,image denoising,arbitrary Riemannian manifolds,camera trajectories,chromaticity values,coarse discretization,convex relaxation techniques,denoising,multilabel optimization problem,optimization problem,three-dimensional rotations,total variation regularization,variational models,variational problems,angular data,denoising,manifold,rotation group,tensor,total variation,variational methods
AI 理解论文
溯源树
样例
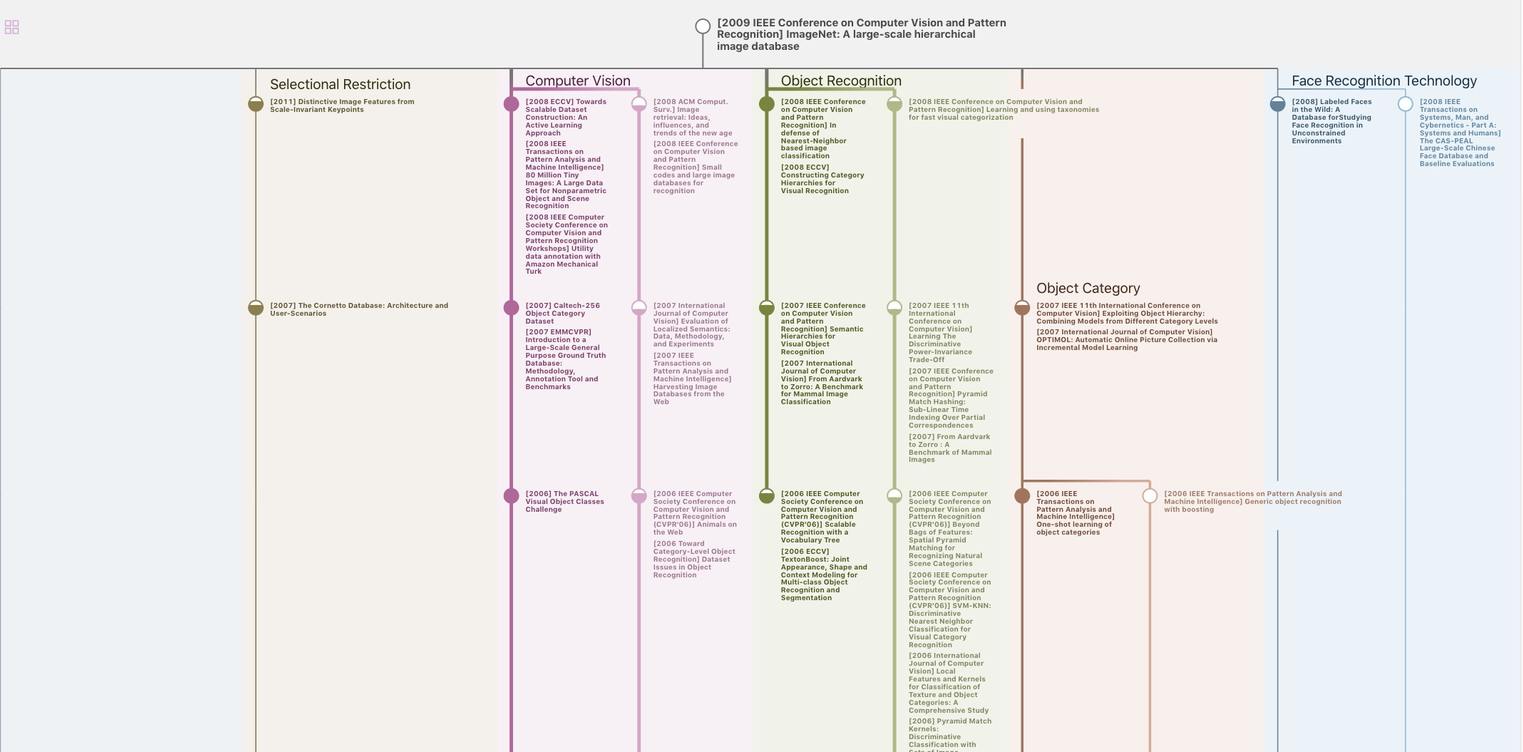
生成溯源树,研究论文发展脉络
Chat Paper
正在生成论文摘要