Pairwise Regression With Upper Confidence Bound For Contextual Bandit With Multiple Actions
TAAI '13 Proceedings of the 2013 Conference on Technologies and Applications of Artificial Intelligence(2013)
摘要
The contextual bandit problem is typically used to model online applications such as article recommendation. However, the problem cannot fully meet certain needs of these applications, such as performing multiple actions at the same time. We defined a new Contextual Bandit Problem with Multiple Actions (CBMA), which is an extension of the traditional contextual bandit problem and fits the online applications better. We adapt some existing contextual bandit algorithms for our CBMA problem, and developed the new Pairwise Regression with Upper Confidence Bound (PairUCB) algorithm which addresses the new properties of the new CBMA problem. Experimental results demonstrate that PairUCB significantly outperforms other approaches.
更多查看译文
关键词
machine learning,contextual bandit,upper confidence bound
AI 理解论文
溯源树
样例
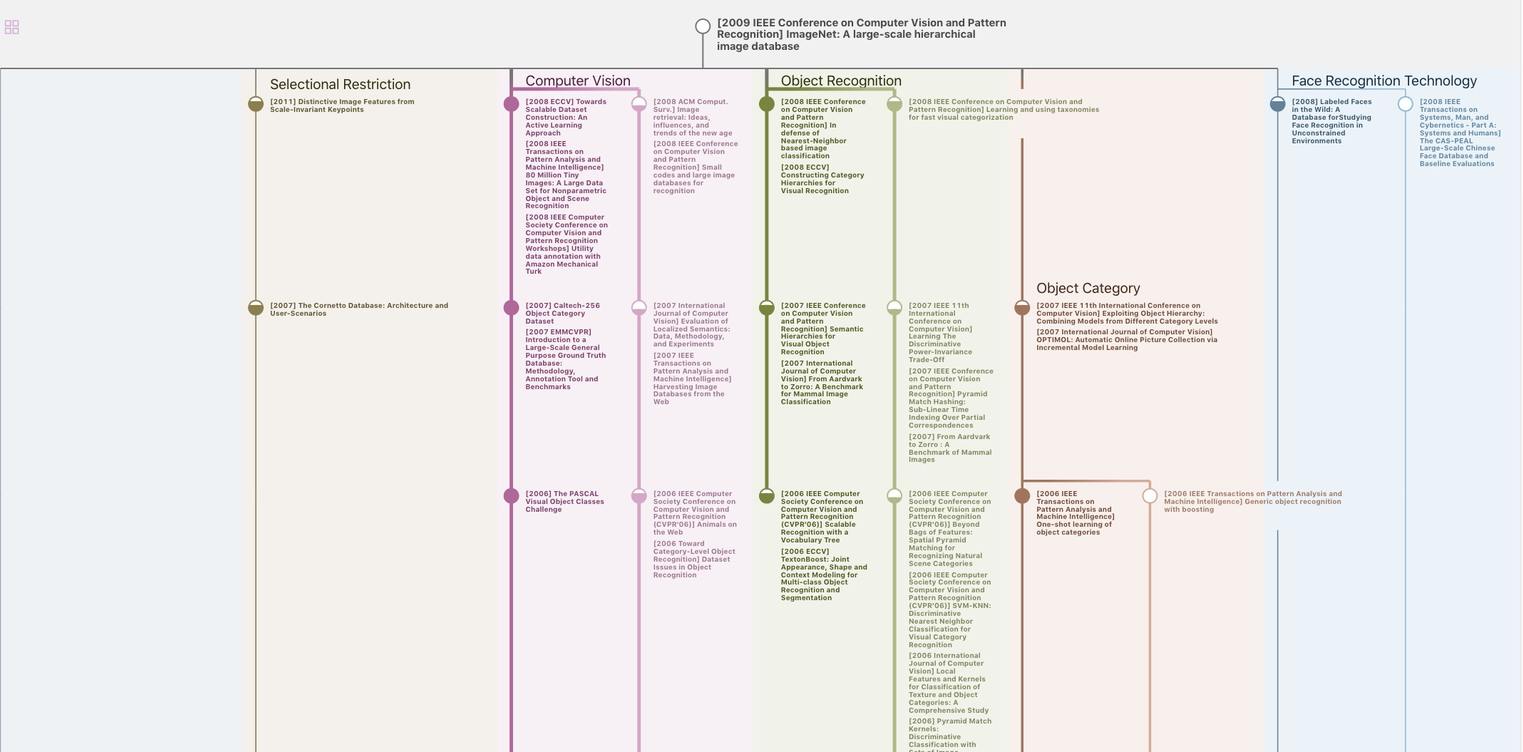
生成溯源树,研究论文发展脉络
Chat Paper
正在生成论文摘要