An Evaluation of Machine Learning Methods to Detect Malicious SCADA Communications
ICMLA), 2013 12th International Conference(2013)
摘要
Critical infrastructure Supervisory Control and Data Acquisition (SCADA) systems have been designed to operate on closed, proprietary networks where a malicious insider posed the greatest threat potential. The centralization of control and the movement towards open systems and standards has improved the efficiency of industrial control, but has also exposed legacy SCADA systems to security threats that they were not designed to mitigate. This work explores the viability of machine learning methods in detecting the new threat scenarios of command and data injection. Similar to network intrusion detection systems in the cyber security domain, the command and control communications in a critical infrastructure setting are monitored, and vetted against examples of benign and malicious command traffic, in order to identify potential attack events. Multiple learning methods are evaluated using a dataset of Remote Terminal Unit communications, which included both normal operations and instances of command and data injection attack scenarios.
更多查看译文
关键词
detect malicious scada communications,critical infrastructure,legacy scada system,critical infrastructure setting,malicious command traffic,data injection,control communication,data injection attack scenario,machine learning methods,industrial control,cyber security domain,greatest threat potential,intrusion detection,network,scada,computer network security,learning artificial intelligence,open systems,machine learning
AI 理解论文
溯源树
样例
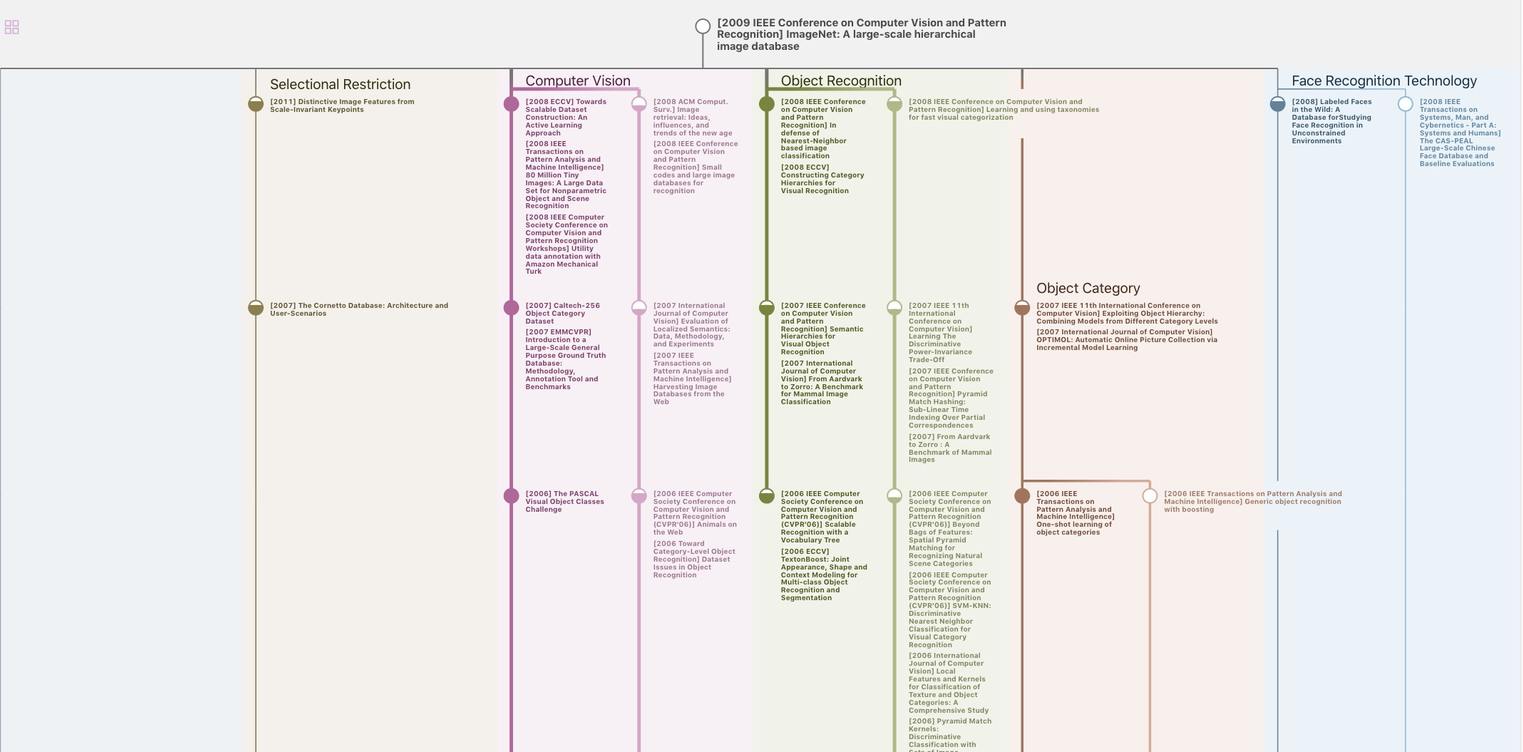
生成溯源树,研究论文发展脉络
Chat Paper
正在生成论文摘要