Scheduling Sensors For Monitoring Sentient Spaces Using An Approximate Pomdp Policy
2013 IEEE INTERNATIONAL CONFERENCE ON PERVASIVE COMPUTING AND COMMUNICATIONS (PERCOM)(2013)
摘要
We present a framework for sensor actuation and control in sentient spaces, in which sensors are used to observe a physical phenomena. We focus on sentient spaces that enable pervasive computing applications, such as smart video surveillance and situational awareness in instrumented office environments. Our framework utilizes the spatio-temporal statistical properties of an observed phenomena, with the goal of maximizing an application-specified reward. Specifically, we define an observation of a phenomena by assigning it a discrete value (state) and we model its semantics as the transition between these values (states). This semantic model is used to predict the future states in which the phenomena is likely to be at, based on partially-observed past states. To accomplish real-time agility, we designed an approximate, adaptive-grid solution for Partially Observable Markov Decision Processes (POMDPs) that yields practically good results, and in some cases, guarantees on the quality of the approximation. We use our framework to control and actuate a large-scale camera network so as to maximize the number and type of captured events. To enable real-time control, we implement an action schedule using a table lookup and make use of a factored probability model to capture state semantics. To the best of our knowledge, we are the first to address the problem of actuating a large-scale sensor network based on a real-time POMDP formulation.
更多查看译文
关键词
semantic model,partially-observed past state,scheduling sensor,approximate pomdp policy,real-time pomdp formulation,large-scale sensor network,real-time control,factored probability model,future state,real-time agility,sentient space,large-scale camera network,algorithms,statistical analysis,partially observable markov decision process,real time,pomdp,ubiquitous computing,pervasive computing,sensor network,markov processes,semantics
AI 理解论文
溯源树
样例
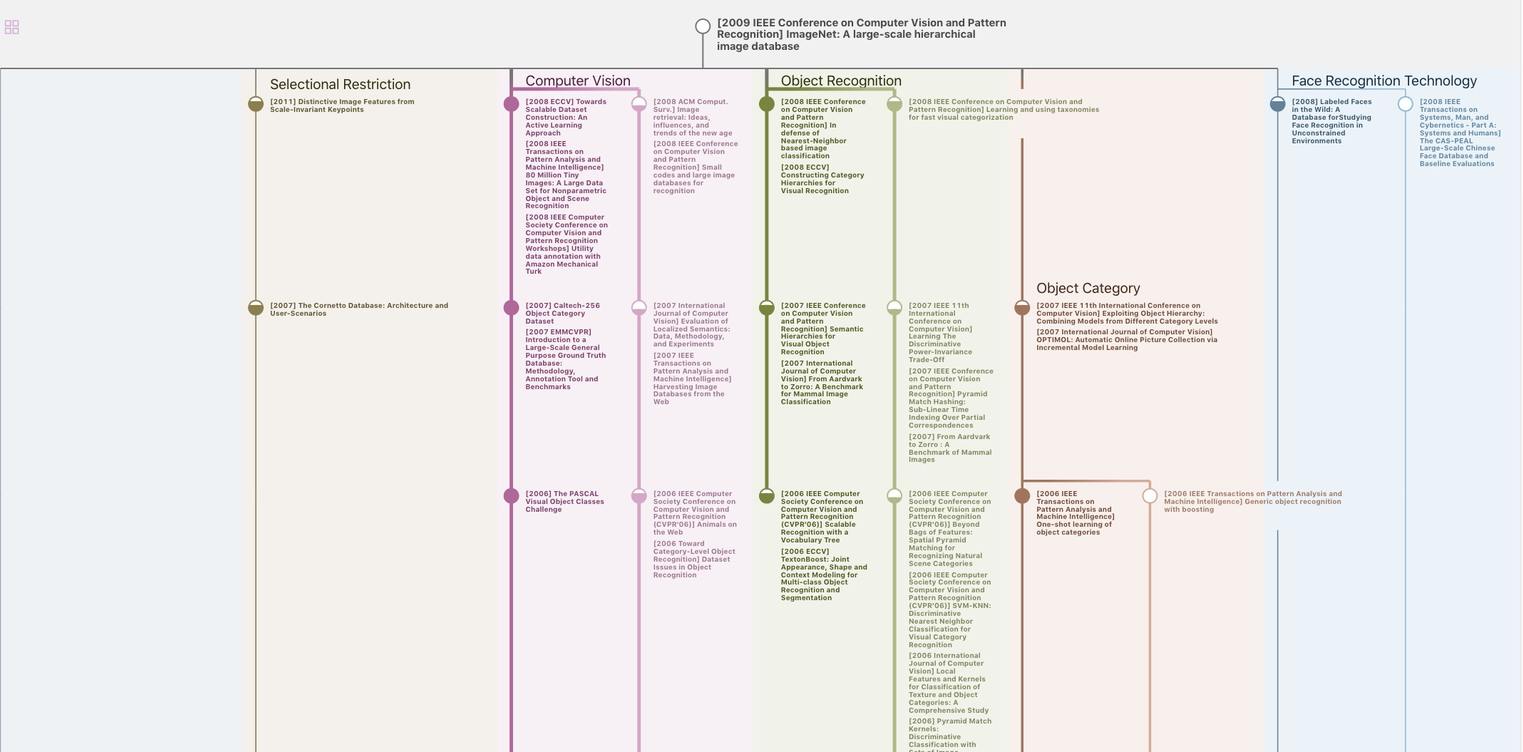
生成溯源树,研究论文发展脉络
Chat Paper
正在生成论文摘要