A size-insensitive integrity-based fuzzy c-means method for data clustering
Pattern Recognition(2014)
摘要
Fuzzy c-means (FCM) is one of the most popular techniques for data clustering. Since FCM tends to balance the number of data points in each cluster, centers of smaller clusters are forced to drift to larger adjacent clusters. For datasets with unbalanced clusters, the partition results of FCM are usually unsatisfactory. Cluster size insensitive FCM (csiFCM) dealt with ''cluster-size sensitivity'' problem by dynamically adjusting the condition value for the membership of each data point based on cluster size after the defuzzification step in each iterative cycle. However, the performance of csiFCM is sensitive to both the initial positions of cluster centers and the ''distance'' between adjacent clusters. In this paper, we present a cluster size insensitive integrity-based FCM method called siibFCM to improve the deficiency of csiFCM. The siibFCM method can determine the membership contribution of every data point to each individual cluster by considering cluster's integrity, which is a combination of compactness and purity. ''Compactness'' represents the distribution of data points within a cluster while ''purity'' represents how far a cluster is away from its adjacent cluster. We tested our siibFCM method and compared with the traditional FCM and csiFCM methods extensively by using artificially generated datasets with different shapes and data distributions, synthetic images, real images, and Escherichia coli dataset. Experimental results showed that the performance of siibFCM is superior to both traditional FCM and csiFCM in terms of the tolerance for ''distance'' between adjacent clusters and the flexibility of selecting initial cluster centers when dealing with datasets with unbalanced clusters.
更多查看译文
关键词
individual cluster,initial cluster center,smaller cluster,fuzzy c-means method,data point,unbalanced cluster,larger adjacent cluster,traditional fcm,cluster size,cluster center,adjacent cluster,compactness,data clustering
AI 理解论文
溯源树
样例
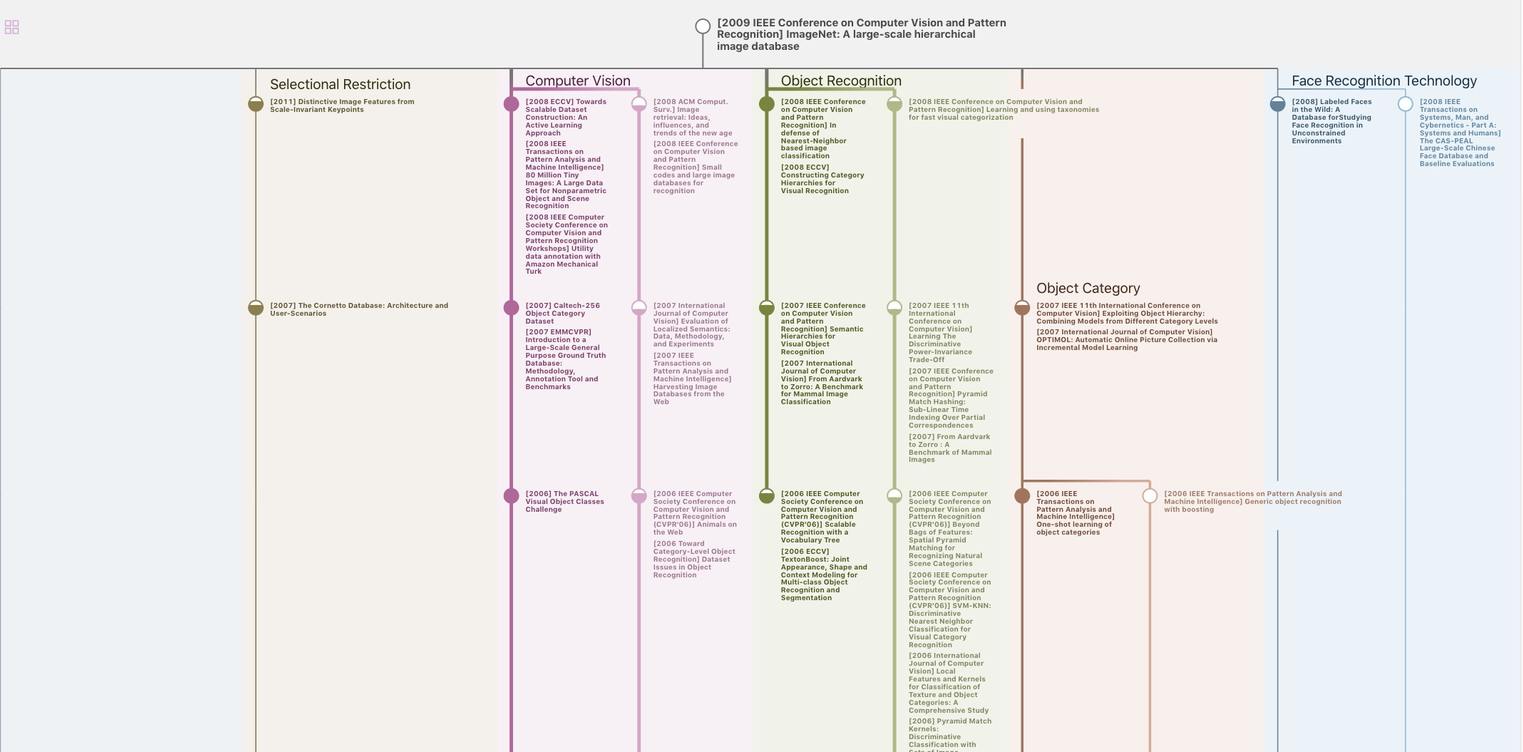
生成溯源树,研究论文发展脉络
Chat Paper
正在生成论文摘要