A Structure-Based Distance Metric for High-Dimensional Space Exploration with Multidimensional Scaling
IEEE Transactions on Visualization and Computer Graphics(2014)
摘要
Although the Euclidean distance does well in measuring data distances within high-dimensional clusters, it does poorly when it comes to gauging inter-cluster distances. This significantly impacts the quality of global, low-dimensional space embedding procedures such as the popular multi-dimensional scaling (MDS) where one can often observe non-intuitive layouts. We were inspired by the perceptual processes evoked in the method of parallel coordinates which enables users to visually aggregate the data by the patterns the polylines exhibit across the dimension axes. We call the path of such a polyline its structure and suggest a metric that captures this structure directly in high-dimensional space. This allows us to better gauge the distances of spatially distant data constellations and so achieve data aggregations in MDS plots that are more cognizant of existing high-dimensional structure similarities. Our bi-scale framework distinguishes far-distances from near-distances. The coarser scale uses the structural similarity metric to separate data aggregates obtained by prior classification or clustering, while the finer scale employs the appropriate Euclidean distance.
更多查看译文
关键词
indexes,data visualization,data visualisation,correlation,high dimensional data,embedded systems,computational geometry,visual analytics,clustering,multidimensional scaling,layout,information visualization,euclidean distance
AI 理解论文
溯源树
样例
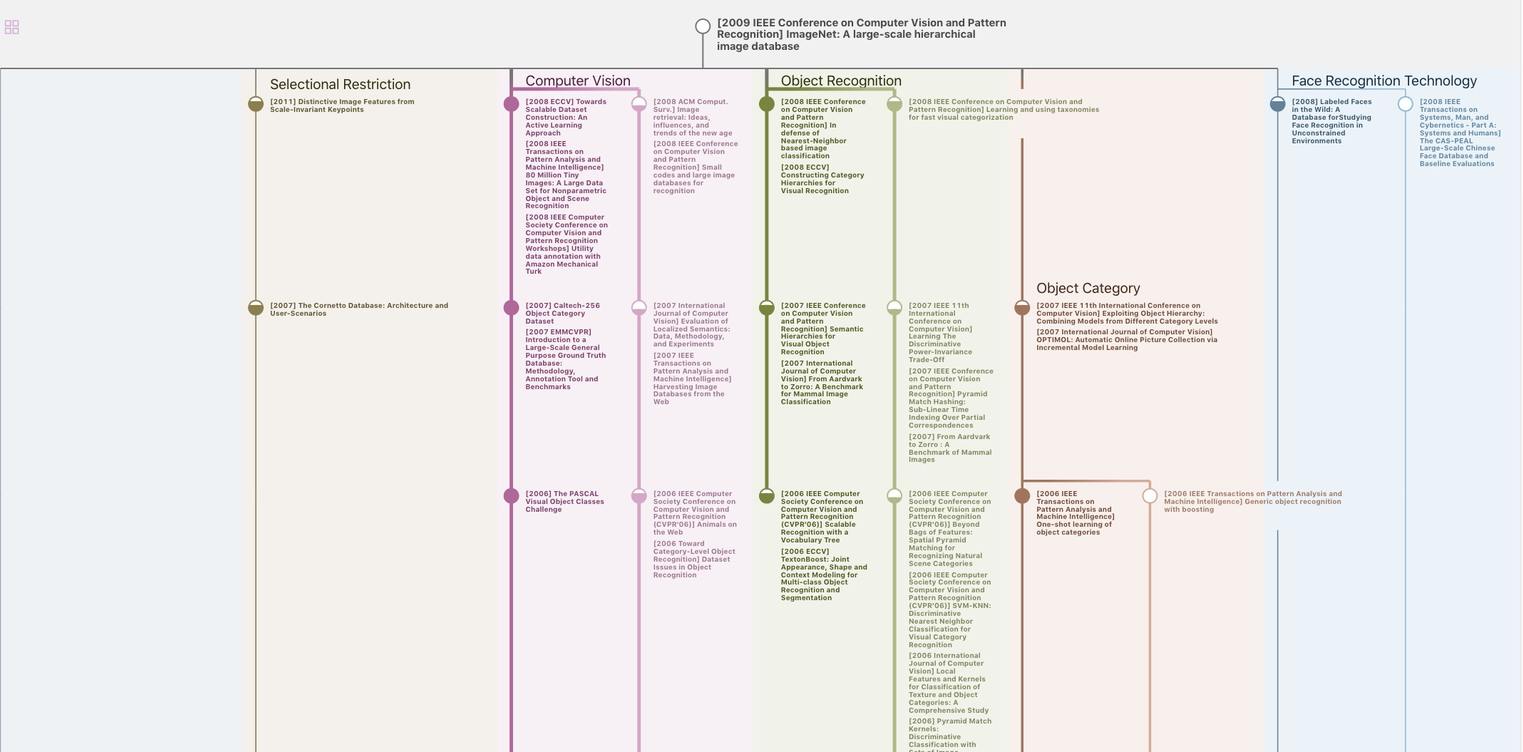
生成溯源树,研究论文发展脉络
Chat Paper
正在生成论文摘要