Pairwise Optimization of Bayesian Classifiers for Multi-class Cost-Sensitive Learning
Tools with Artificial Intelligence(2013)
摘要
In this paper, we present a new approach to enhance the performance of Bayesian classifiers. Our method relies on the combination of two ideas: pairwise classification on the one hand, and threshold optimization on the other hand. Introducing one threshold per pair of classes increases the expressivity of the model, therefore its performance on complex problems such as cost-sensitive problems increases as well. Indeed a comparison of our algorithm to other cost-sensitive approaches shows that it reduces the total misclassification cost.
更多查看译文
关键词
optimisation,cost-sensitive problems,bayesian classifier,pairwise classification,bayes methods,learning (artificial intelligence),threshold optimization,complex problem,pattern classification,bayesian classifiers,multi-class cost-sensitive learning,cost-sensitive problems increase,binarization,multi-class learning,misclassification cost,multiclass cost-sensitive learning,cost-sensitive approach,new approach,total misclassification cost,cost-sensitive learning,pairwise optimization,learning artificial intelligence
AI 理解论文
溯源树
样例
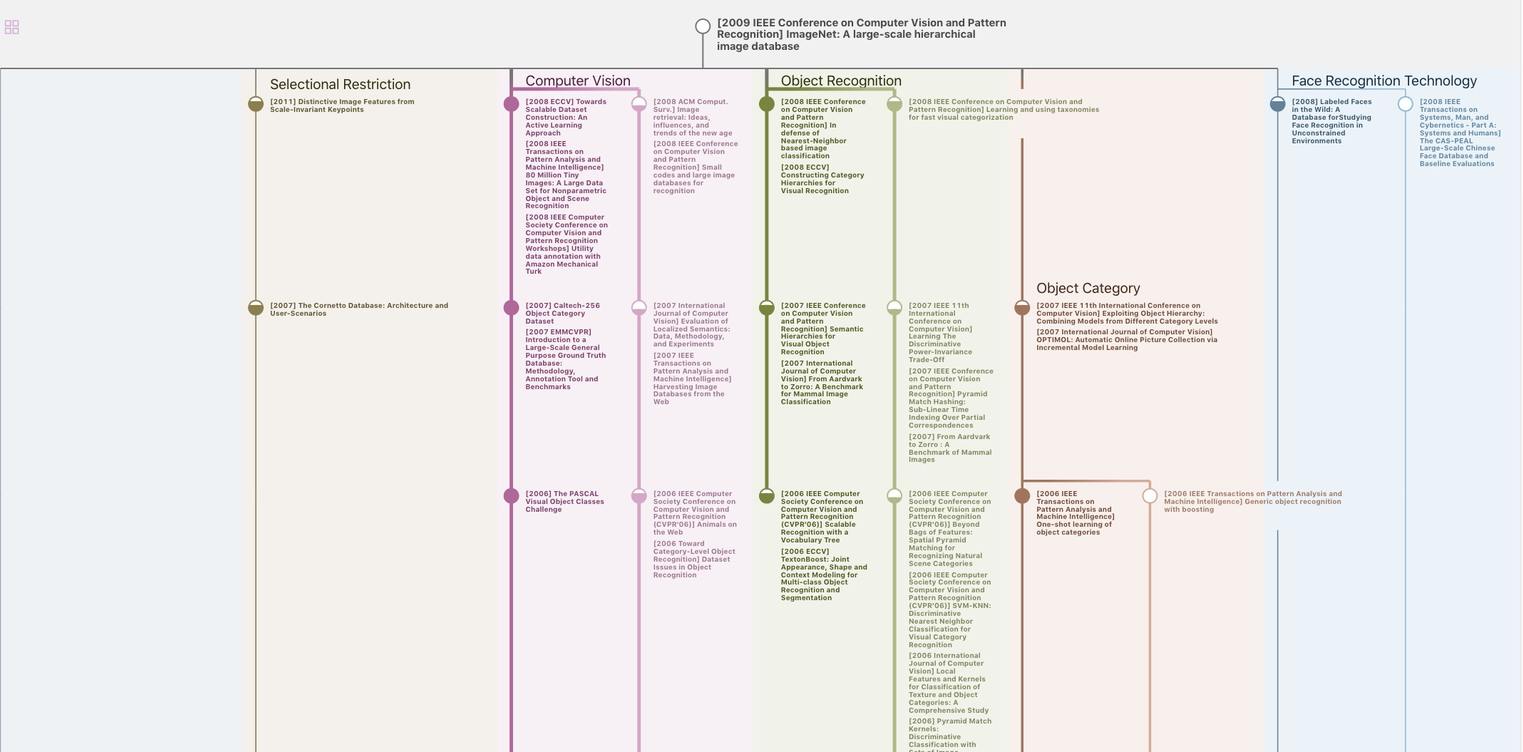
生成溯源树,研究论文发展脉络
Chat Paper
正在生成论文摘要