Sentiment classification: The contribution of ensemble learning
Decision Support Systems(2014)
摘要
With the rapid development of information technologies, user-generated contents can be conveniently posted online. While individuals, businesses, and governments are interested in evaluating the sentiments behind this content, there are no consistent conclusions on which sentiment classification technologies are best. Recent studies suggest that ensemble learning methods may have potential applicability in sentiment classification. In this study, we conduct a comparative assessment of the performance of three popular ensemble methods (Bagging, Boosting, and Random Subspace) based on five base learners (Naive Bayes, Maximum Entropy, Decision Tree, K Nearest Neighbor, and Support Vector Machine) for sentiment classification. Moreover, ten public sentiment analysis datasets were investigated to verify the effectiveness of ensemble learning for sentiment analysis. Based on a total of 1200 comparative group experiments, empirical results reveal that ensemble methods substantially improve the performance of individual base learners for sentiment classification. Among the three ensemble methods, Random Subspace has the better comparative results, although it was seldom discussed in the literature. These results illustrate that ensemble learning methods can be used as a viable method for sentiment classification.
更多查看译文
关键词
random subspace,comparative group experiment,comparative assessment,comparative result,public sentiment analysis datasets,ensemble learning,ensemble method,sentiment classification technology,popular ensemble method,sentiment analysis,sentiment classification,bagging,boosting
AI 理解论文
溯源树
样例
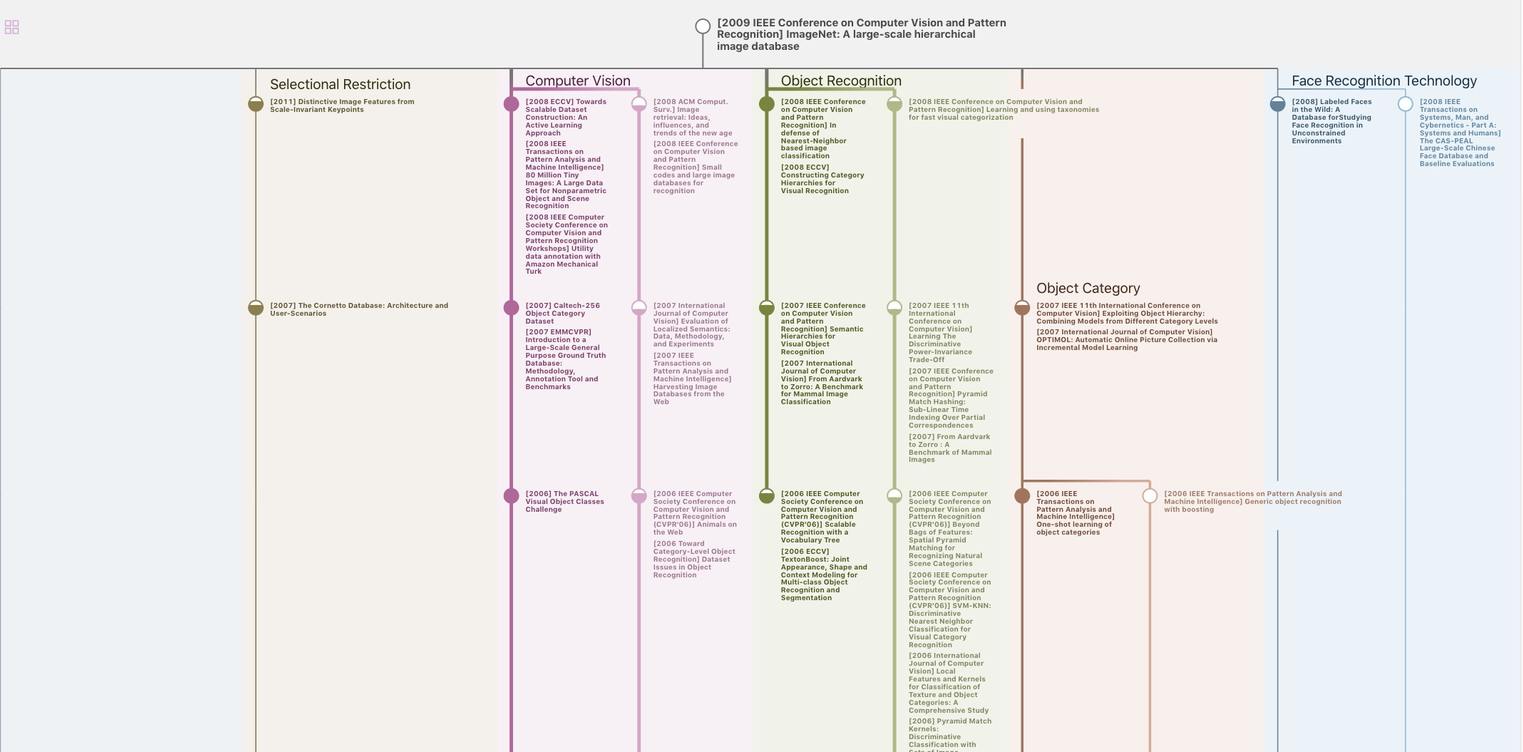
生成溯源树,研究论文发展脉络
Chat Paper
正在生成论文摘要