Dependent binary relevance models for multi-label classification
Pattern Recognition(2014)
摘要
Several meta-learning techniques for multi-label classification (MLC), such as chaining and stacking, have already been proposed in the literature, mostly aimed at improving predictive accuracy through the exploitation of label dependencies. In this paper, we propose another technique of that kind, called dependent binary relevance (DBR) learning. DBR combines properties of both, chaining and stacking. We provide a careful analysis of the relationship between these and other techniques, specifically focusing on the underlying dependency structure and the type of training data used for model construction. Moreover, we offer an extensive empirical evaluation, in which we compare different techniques on MLC benchmark data. Our experiments provide evidence for the good performance of DBR in terms of several evaluation measures that are commonly used in MLC.
更多查看译文
关键词
different technique,training data,dependent binary relevance,extensive empirical evaluation,evaluation measure,dependent binary relevance model,good performance,mlc benchmark data,multi-label classification,label dependency,careful analysis,meta-learning technique,stacking,chaining
AI 理解论文
溯源树
样例
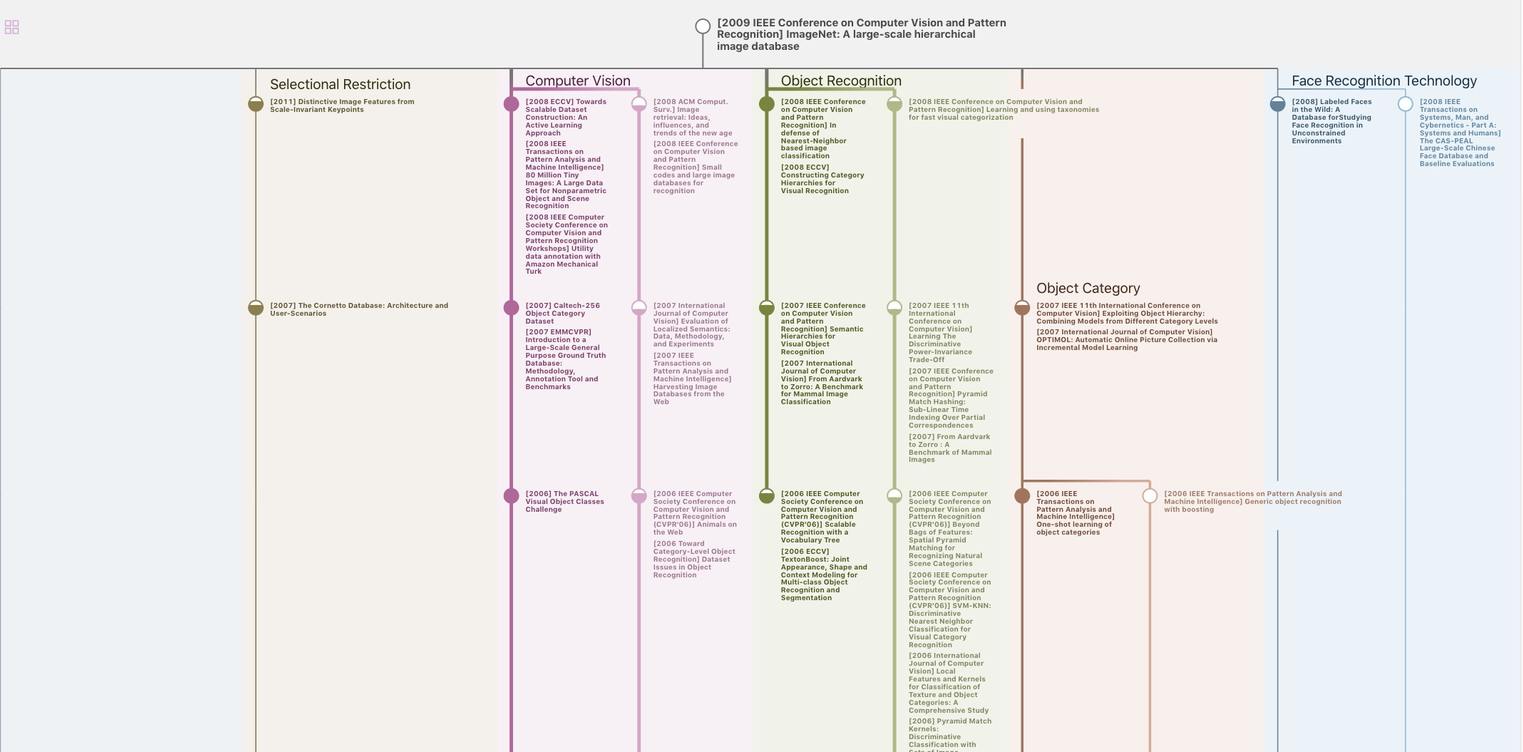
生成溯源树,研究论文发展脉络
Chat Paper
正在生成论文摘要