Hybrid Deep Neural Network--Hidden Markov Model (DNN-HMM) Based Speech Emotion Recognition
ACII '13 Proceedings of the 2013 Humaine Association Conference on Affective Computing and Intelligent Interaction(2013)
摘要
Deep Neural Network Hidden Markov Models, or DNN-HMMs, are recently very promising acoustic models achieving good speech recognition results over Gaussian mixture model based HMMs (GMM-HMMs). In this paper, for emotion recognition from speech, we investigate DNN-HMMs with restricted Boltzmann Machine (RBM) based unsupervised pre-training, and DNN-HMMs with discriminative pre-training. Emotion recognition experiments are carried out on these two models on the eNTERFACE'05 database and Berlin database, respectively, and results are compared with those from the GMM-HMMs, the shallow-NN-HMMs with two layers, as well as the Multi-layer Perceptrons HMMs (MLP-HMMs). Experimental results show that when the numbers of the hidden layers as well hidden units are properly set, the DNN could extend the labeling ability of GMM-HMM. Among all the models, the DNN-HMMs with discriminative pre-training obtain the best results. For example, for the eNTERFACE'05 database, the recognition accuracy improves 12.22% from the DNN-HMMs with unsupervised pre-training, 11.67% from the GMM-HMMs, 10.56% from the MLP-HMMs, and even 17.22% from the shallow-NN-HMMs, respectively.
更多查看译文
关键词
recognition accuracy,good speech recognition result,multilayer perceptrons hmm,boltzmann machines,speech recognition,berlin database,hidden layer,multi-layer perceptrons hmms,hybrid deep neural network-hidden markov model based speech emotion recognition,acoustic models,emotion recognition experiment,discriminative pre-training,hidden unit,emotion recognition,hidden markov model,rbm,gmm-hmm,speech emotion recognition,gaussian mixture model based hmm,unsupervised pre-training,dnn-hmm,hybrid deep neural network,hidden markov models,restricted boltzmann machine,unsupervised learning,enterface 05 database
AI 理解论文
溯源树
样例
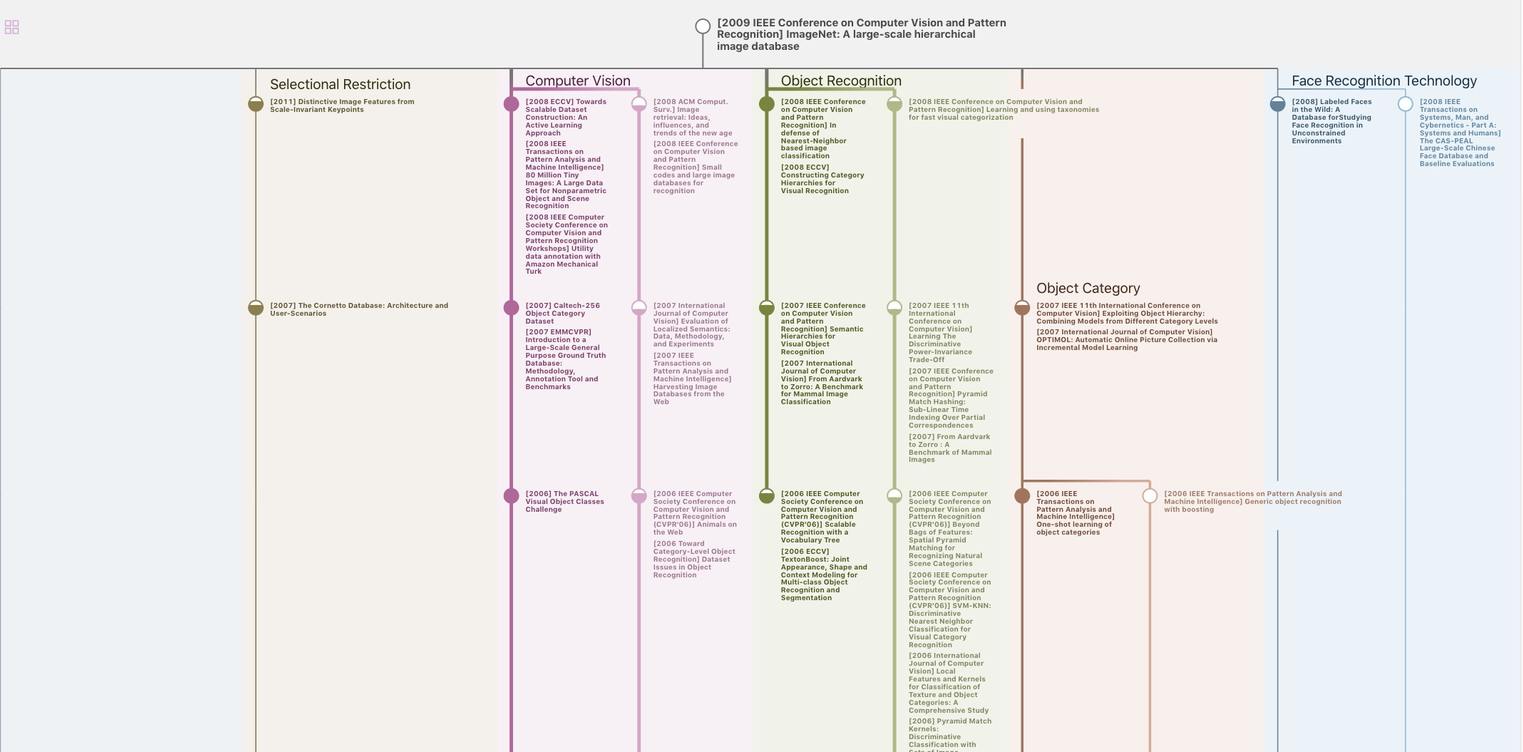
生成溯源树,研究论文发展脉络
Chat Paper
正在生成论文摘要