Multi-task Forest for Human Pose Estimation in Depth Images
Seattle, WA(2013)
摘要
In this paper, we address the problem of human body pose estimation from depth data. Previous works Based on random forests relied either on a classification strategy to infer the different body parts or on a regression approach to predict directly the joint positions. To permit the inference of very generic poses, those approaches did not consider additional information during the learning phase, e.g. the performed activity. In the present work, we introduce a novel approach to integrate additional information at training time that actually improves the pose prediction during the testing. Our main contribution is a multi-task forest that aims at solving a joint regression-classification task: each foreground pixel from a depth image is associated to its relative displacements to the 3D joint positions as well as the activity class. Integrating activity information in the objective function during forest training permits a better partitioning of the 3D pose space that leads to a better modelling of the posterior. Thereby, our approach provides an improved pose prediction, and as a by-product, can give an estimate of the performed activity. We performed experiments on a dataset performed by 10 people associated with the ground truth body poses from a motion capture system. To demonstrate the benefits of our approach, poses are divided into 10 different activities for the training phase. Results on this dataset show that our multi-task forest provides improved human pose estimation compared to a pure regression forest approach.
更多查看译文
关键词
regression approach,novel approach,joint position,human pose estimation,forest training,activity class,additional information,different activity,multi-task forest,integrating activity information,pure regression forest approach,depth images,image resolution,image classification,pose estimation,regression analysis,learning artificial intelligence
AI 理解论文
溯源树
样例
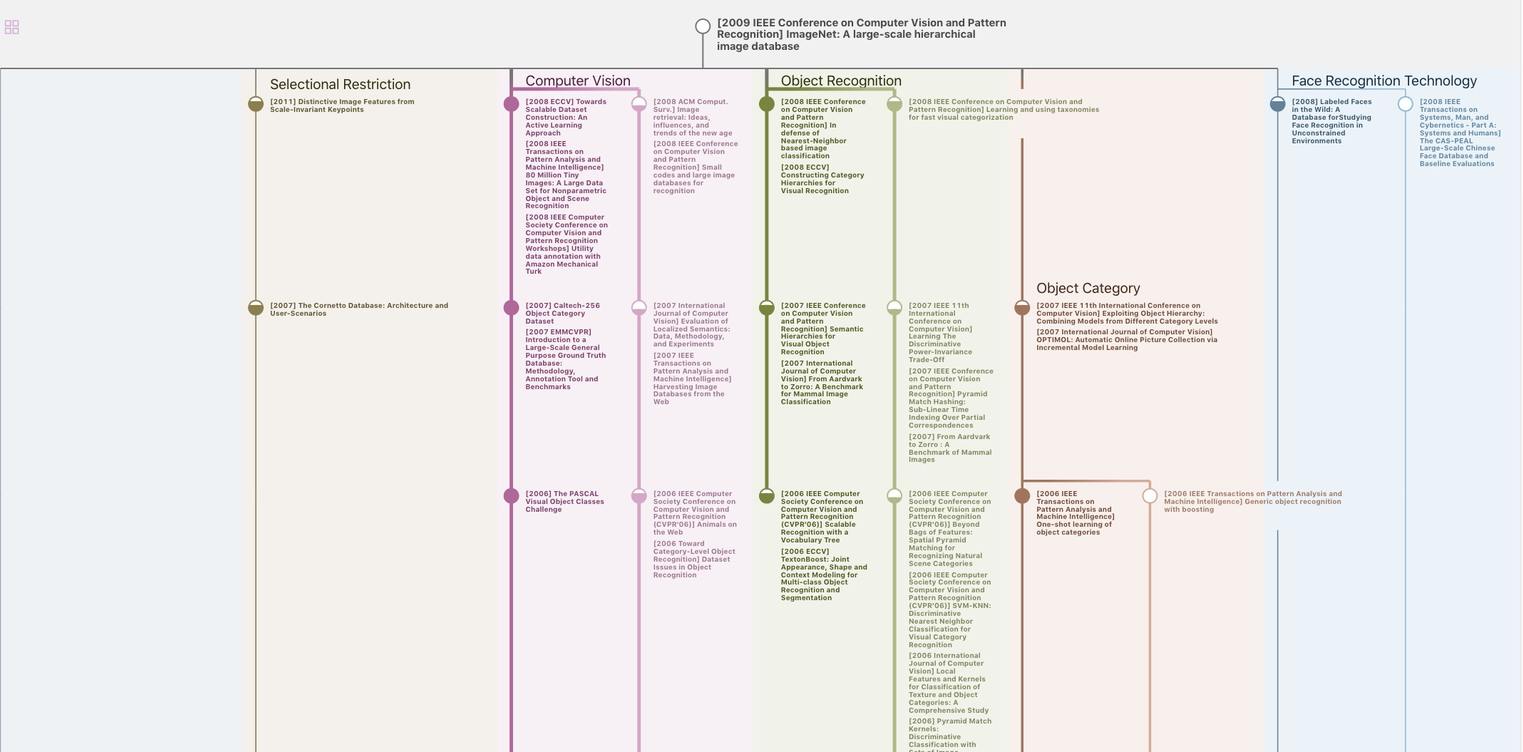
生成溯源树,研究论文发展脉络
Chat Paper
正在生成论文摘要