Bayesian predictive kernel discriminant analysis
Pattern Recognition Letters(2013)
摘要
Discriminant analysis using Kernel Density Estimator (KDE) is a common tool for classification, but depends on the choice of the bandwidth or smoothing parameter of kernel. In this paper, we introduce a Bayesian Predictive Kernel Discriminant Analysis (BPKDA) eliminating this dependence by integrating the KDE with respect to an appropriate prior probability distribution for the bandwidth. Keypoints of the method are: (1) the formulation of the classification rule in terms of mixture predictive densities obtained by integrating kernel; (2) use of Independent Components Analysis (ICA) to choose a transform matrix so that transformed components are as independent as possible; and (3) nonparametric estimation of the predictive density by KDE for each independent component. Results on benchmark data sets and simulations show that the performance of BPKDA is competitive with, and in some cases significantly better than, Linear Discriminant Analysis (LDA), Quadratic Discriminant Analysis (QDA) and Naives Bayes discriminant Analysis with normal distribution (NNBDA).
更多查看译文
关键词
linear discriminant analysis,quadratic discriminant analysis,independent components analysis,bayesian predictive kernel discriminant,discriminant analysis,kernel density estimator,mixture predictive density,appropriate prior probability distribution,independent component,classification rule,bayesian estimation
AI 理解论文
溯源树
样例
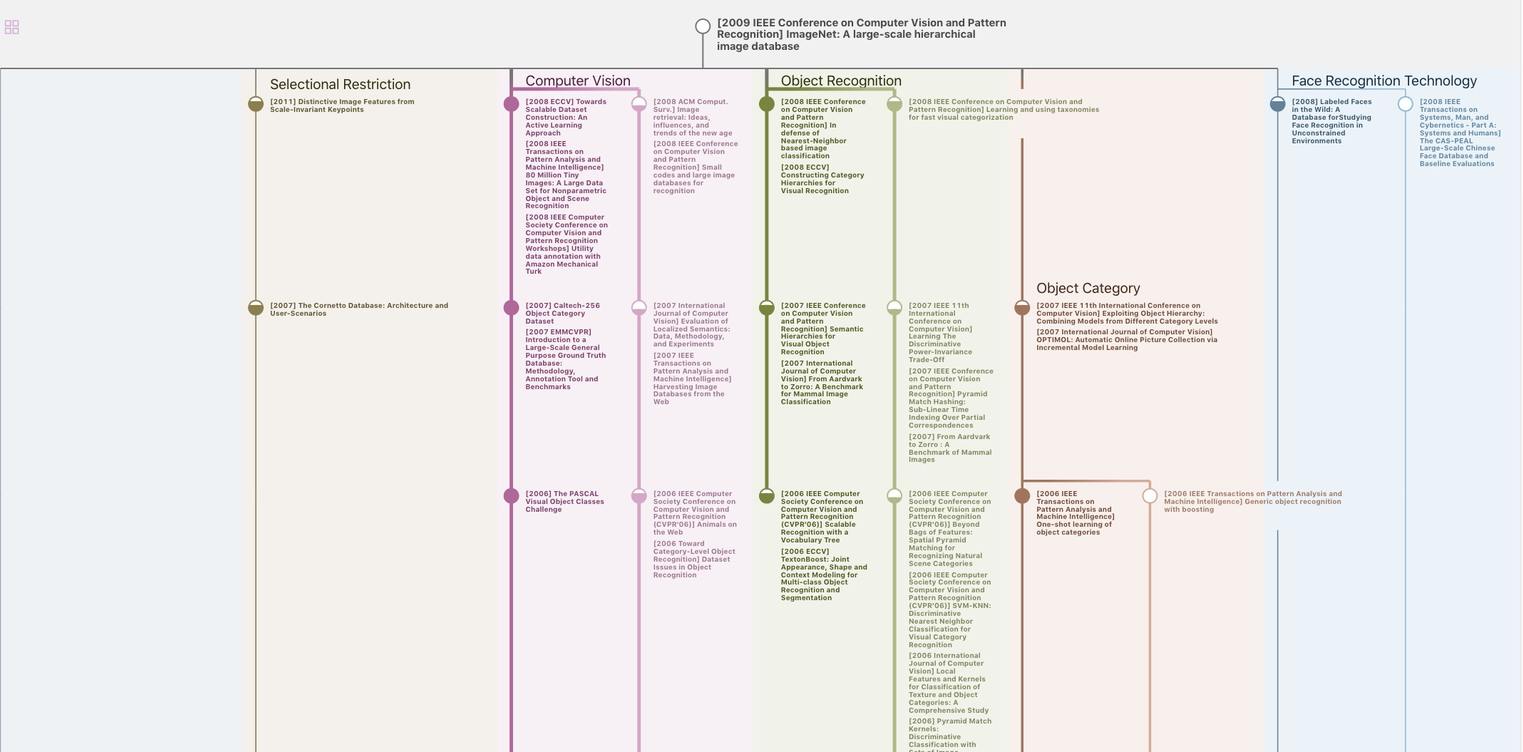
生成溯源树,研究论文发展脉络
Chat Paper
正在生成论文摘要